Recurrent networks improve neural response prediction and provide insights into underlying cortical circuits
arxiv(2022)
摘要
Feedforward CNN models have proven themselves in recent years as state-of-the-art models for predicting single-neuron responses to natural images in early visual cortical neurons. In this paper, we extend these models with recurrent convolutional layers, reflecting the well-known massive recurrence in the cortex, and show robust increases in predictive performance over feedforward models across thousands of hyperparameter combinations in three datasets of macaque V1 and V2 single-neuron responses. We propose the recurrent circuit can be conceptualized as a form of ensemble computing, with each iteration generating more effective feedforward paths of various path lengths to allow a combination of solutions in the final approximation. The statistics of the paths in the ensemble provide insights to the differential performance increases among our recurrent models. We also assess whether the recurrent circuits learned for neural response prediction can be related to cortical circuits. We find that the hidden units in the recurrent circuits of the appropriate models, when trained on long-duration wide-field image presentations, exhibit similar temporal response dynamics and classical contextual modulations as observed in V1 neurons. This work provides insights to the computational rationale of recurrent circuits and suggests that neural response prediction could be useful for characterizing the recurrent neural circuits in the visual cortex.
更多查看译文
关键词
neural response prediction,recurrent networks,cortical circuits
AI 理解论文
溯源树
样例
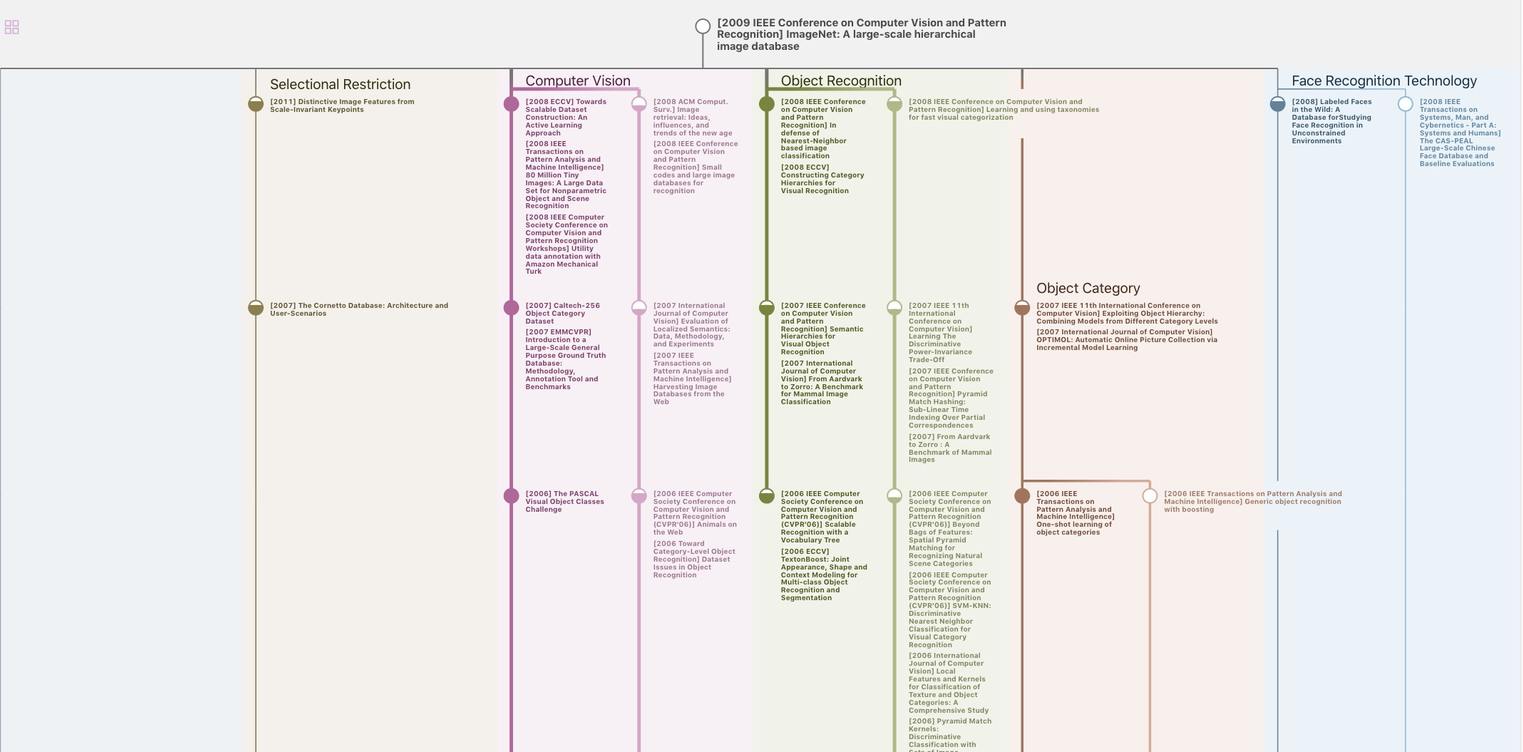
生成溯源树,研究论文发展脉络
Chat Paper
正在生成论文摘要