RoomStructNet: Learning to Rank Non-Cuboidal Room Layouts From Single View
arxiv(2021)
摘要
In this paper, we present a new approach to estimate the layout of a room from its single image. While recent approaches for this task use robust features learnt from data, they resort to optimization for detecting the final layout. In addition to using learnt robust features, our approach learns an additional ranking function to estimate the final layout instead of using optimization. To learn this ranking function, we propose a framework to train a CNN using max-margin structure cost. Also, while most approaches aim at detecting cuboidal layouts, our approach detects non-cuboidal layouts for which we explicitly estimates layout complexity parameters. We use these parameters to propose layout candidates in a novel way. Our approach shows state-of-the-art results on standard datasets with mostly cuboidal layouts and also performs well on a dataset containing rooms with non-cuboidal layouts.
更多查看译文
关键词
single view,roomstructnet,learning,non-cuboidal
AI 理解论文
溯源树
样例
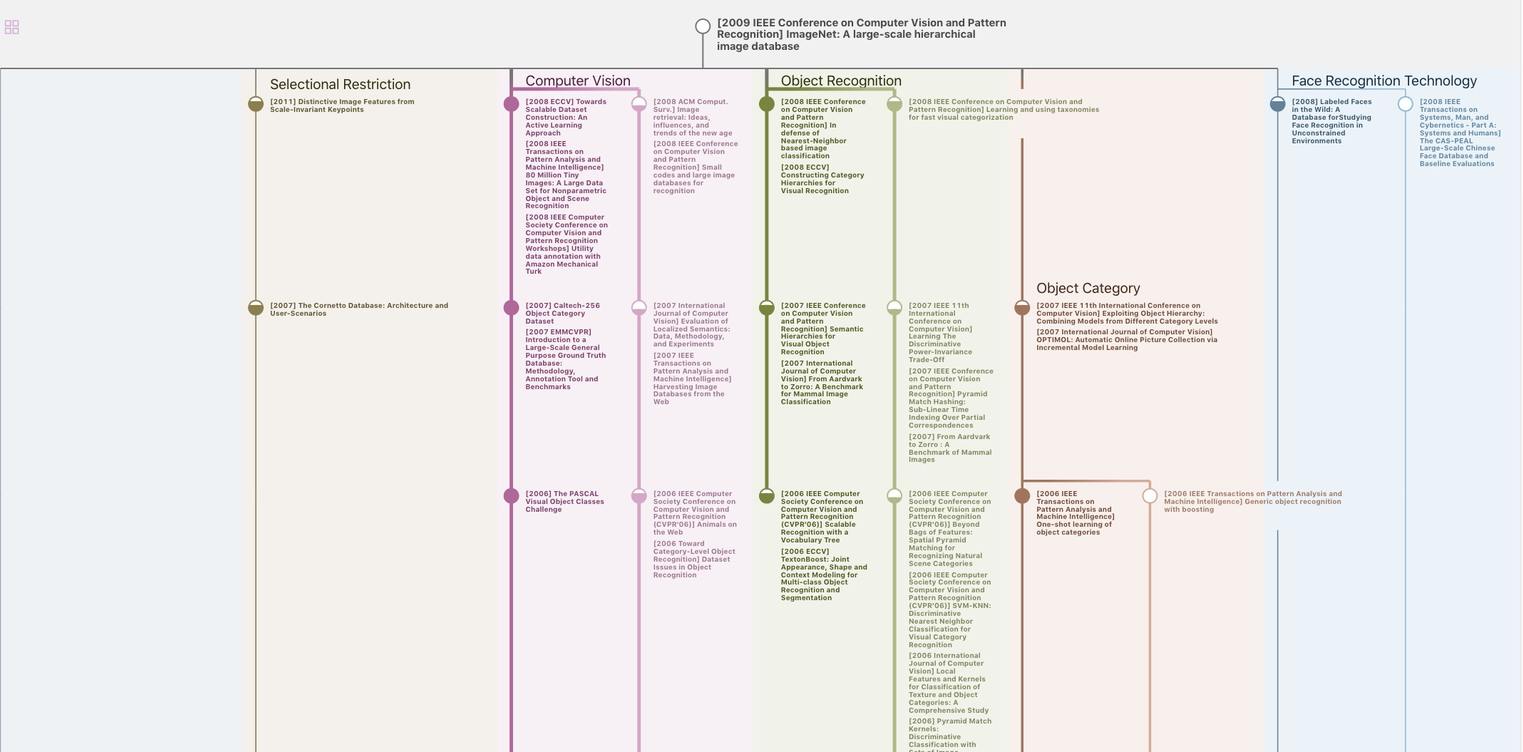
生成溯源树,研究论文发展脉络
Chat Paper
正在生成论文摘要