SPEC: Seeing People in the Wild with an Estimated Camera
ICCV(2021)
摘要
Due to the lack of camera parameter information for in-the-wild images, existing 3D human pose and shape (HPS) estimation methods make several simplifying assumptions: weak-perspective projection, large constant focal length, and zero camera rotation. These assumptions often do not hold and we show, quantitatively and qualitatively, that they cause errors in the reconstructed 3D shape and pose. To address this, we introduce SPEC, the first in-the-wild 3D HPS method that estimates the perspective camera from a single image and employs this to reconstruct 3D human bodies more accurately. First, we train a neural network to estimate the field of view, camera pitch, and roll given an input image. We employ novel losses that improve the calibration accuracy over previous work. We then train a novel network that concatenates the camera calibration to the image features and uses these together to regress 3D body shape and pose. SPEC is more accurate than the prior art on the standard benchmark (3DPW) as well as two new datasets with more challenging camera views and varying focal lengths. Specifically, we create a new photorealistic synthetic dataset (SPEC-SYN) with ground truth 3D bodies and a novel in-the-wild dataset (SPEC-MTP) with calibration and high-quality reference bodies. Code and datasets are available for research purposes at https://spec.is.tue.mpg.de/.
更多查看译文
关键词
Gestures and body pose,3D from a single image and shape-from-x
AI 理解论文
溯源树
样例
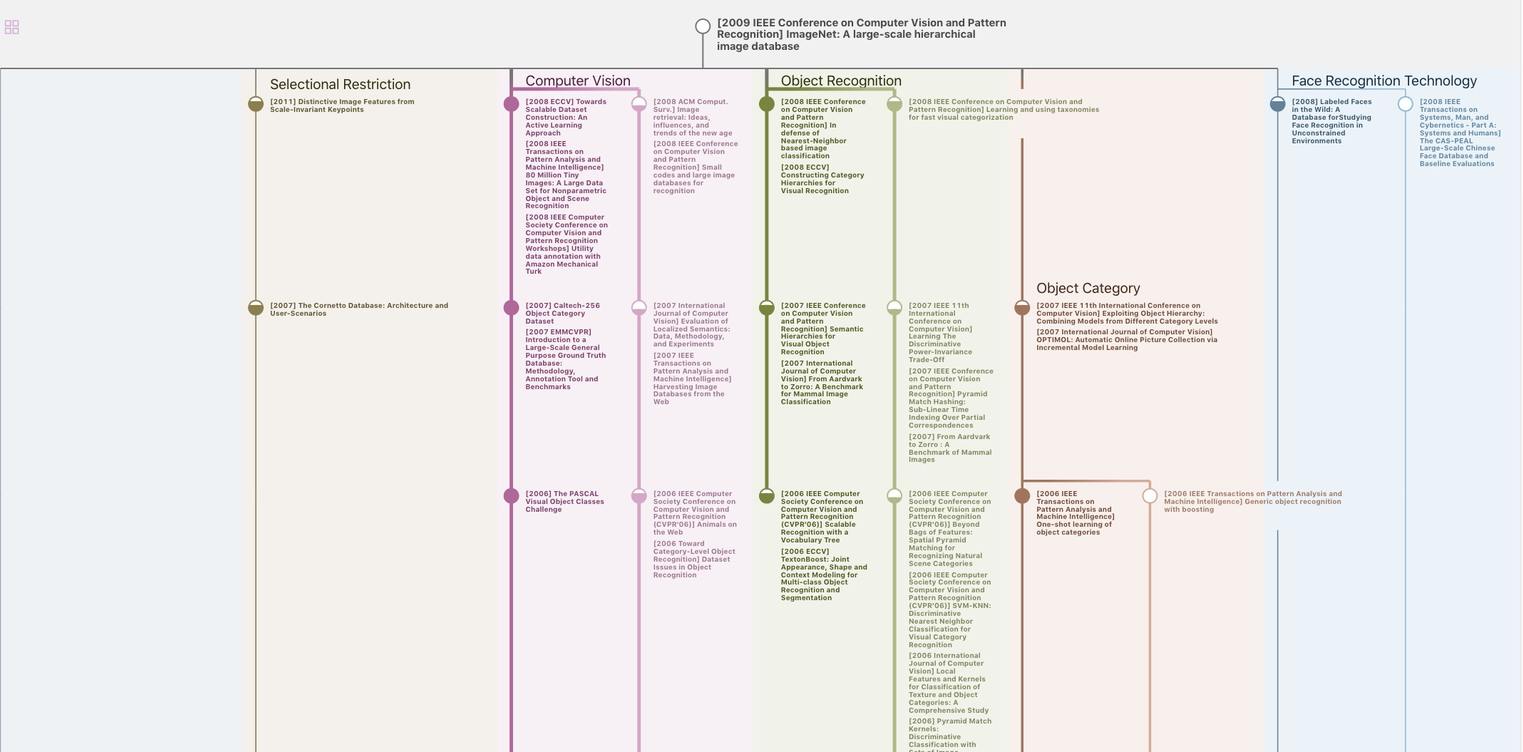
生成溯源树,研究论文发展脉络
Chat Paper
正在生成论文摘要