Free Adversarial Training with Layerwise Heuristic Learning.
ICIG(2021)
摘要
Due to the existence of adversarial attacks, various applications that employ deep neural networks (DNNs) have been under threat. Adversarial training enhances robustness of DNN-based systems by augmenting training data with adversarial samples. Projected gradient descent adversarial training (PGD AT), one of the promising defense methods, can resist strong attacks. We propose “free” adversarial training with layerwise heuristic learning ( LHFAT ) to remedy these problems. To reduce heavy computation cost, we couple model parameter updating with projected gradient descent (PGD) adversarial example updating while retraining the same mini-batch of data, where we “free” and unburden extra updates. Learning rate reflects weight updating speed. Weight gradient indicates weight updating efficiency. If weights are frequently updated towards opposite directions in one training epoch, then there are redundant updates. For higher level of weight updating efficiency, we design a new learning scheme, layerwise heuristic learning, which accelerates training convergence by restraining redundant weight updating and boosting efficient weight updating of layers according to weight gradient information. We demonstrate that LHFAT yields better defense performance on CIFAR-10 with approximately 8% GPU training time of PGD AT and LHFAT is also validated on ImageNet. We have released the code for our proposed method LHFAT at https://github.com/anonymous530/LHFAT .
更多查看译文
关键词
learning,training
AI 理解论文
溯源树
样例
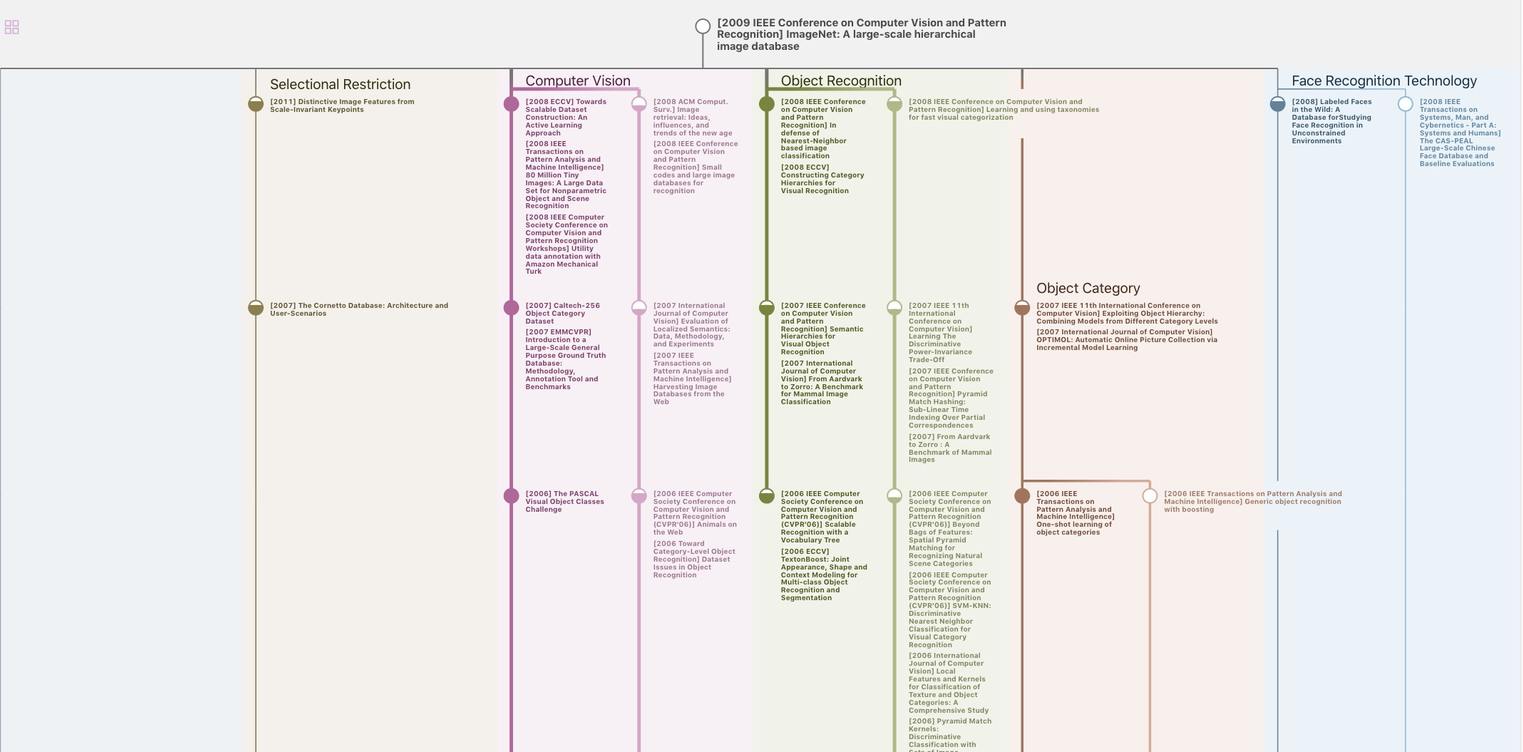
生成溯源树,研究论文发展脉络
Chat Paper
正在生成论文摘要