A survey on datasets for fairness-aware machine learning
WILEY INTERDISCIPLINARY REVIEWS-DATA MINING AND KNOWLEDGE DISCOVERY(2022)
摘要
As decision-making increasingly relies on machine learning (ML) and (big) data, the issue of fairness in data-driven artificial intelligence systems is receiving increasing attention from both research and industry. A large variety of fairness-aware ML solutions have been proposed which involve fairness-related interventions in the data, learning algorithms, and/or model outputs. However, a vital part of proposing new approaches is evaluating them empirically on benchmark datasets that represent realistic and diverse settings. Therefore, in this paper, we overview real-world datasets used for fairness-aware ML. We focus on tabular data as the most common data representation for fairness-aware ML. We start our analysis by identifying relationships between the different attributes, particularly with respect to protected attributes and class attribute, using a Bayesian network. For a deeper understanding of bias in the datasets, we investigate interesting relationships using exploratory analysis. This article is categorized under: Commercial, Legal, and Ethical Issues > Fairness in Data Mining Fundamental Concepts of Data and Knowledge > Data Concepts Technologies > Data Preprocessing
更多查看译文
关键词
benchmark datasets, bias, datasets for fairness, discrimination, fairness-aware machine learning
AI 理解论文
溯源树
样例
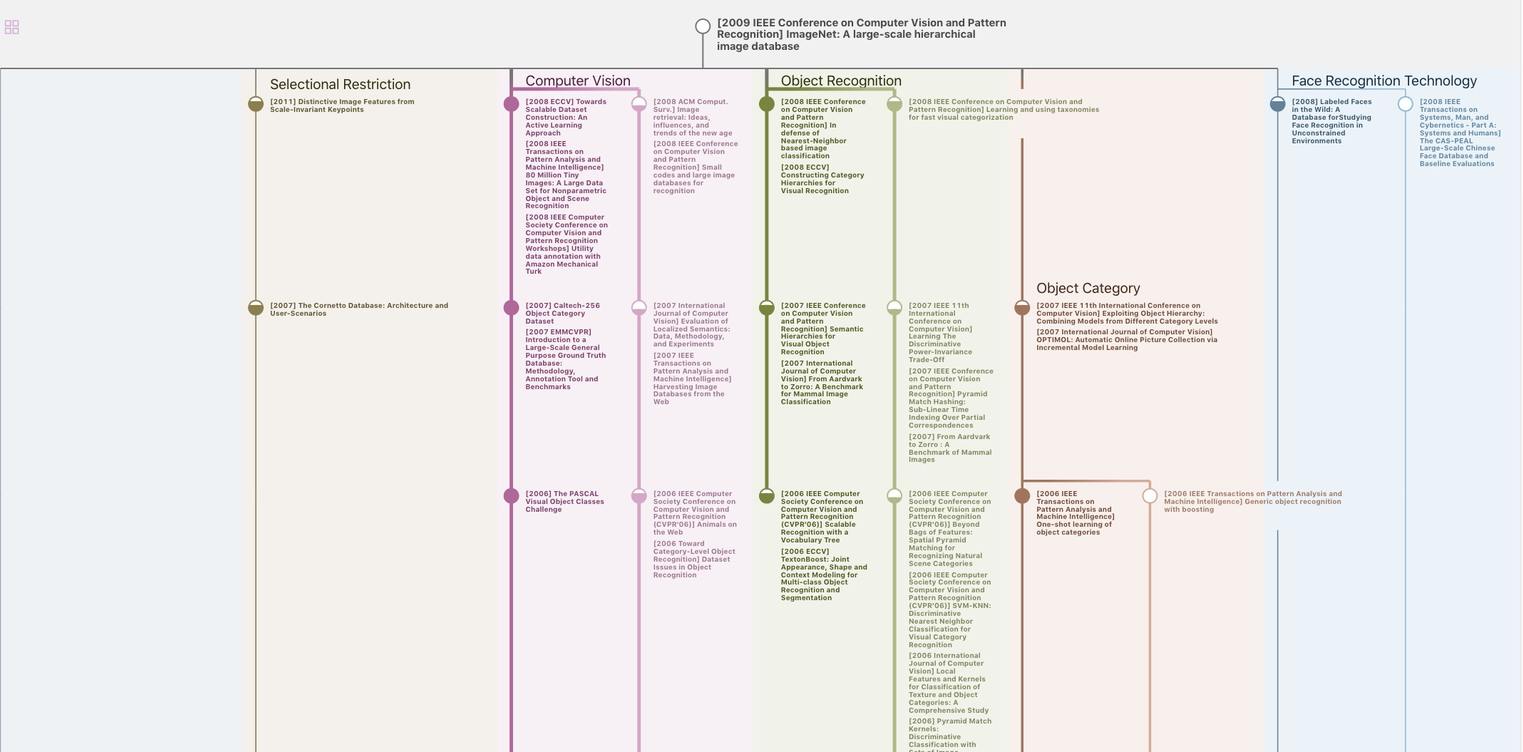
生成溯源树,研究论文发展脉络
Chat Paper
正在生成论文摘要