Student Helping Teacher: Teacher Evolution via Self-Knowledge Distillation
arxiv(2021)
摘要
Knowledge distillation usually transfers the knowledge from a pre-trained cumbersome teacher network to a compact student network, which follows the classical teacher-teaching-student paradigm. Based on this paradigm, previous methods mostly focus on how to efficiently train a better student network for deployment. Different from the existing practices, in this paper, we propose a novel student-helping-teacher formula, Teacher Evolution via Self-Knowledge Distillation (TESKD), where the target teacher (for deployment) is learned with the help of multiple hierarchical students by sharing the structural backbone. The diverse feedback from multiple students allows the teacher to improve itself through the shared feature representations. The effectiveness of our proposed framework is demonstrated by extensive experiments with various network settings on two standard benchmarks including CIFAR-100 and ImageNet. Notably, when trained together with our proposed method, ResNet-18 achieves 79.15% and 71.14% accuracy on CIFAR-100 and ImageNet, outperforming the baseline results by 4.74% and 1.43%, respectively. The code is available at: https://github.com/zhengli427/TESKD.
更多查看译文
关键词
teacher evolution,student,self-knowledge
AI 理解论文
溯源树
样例
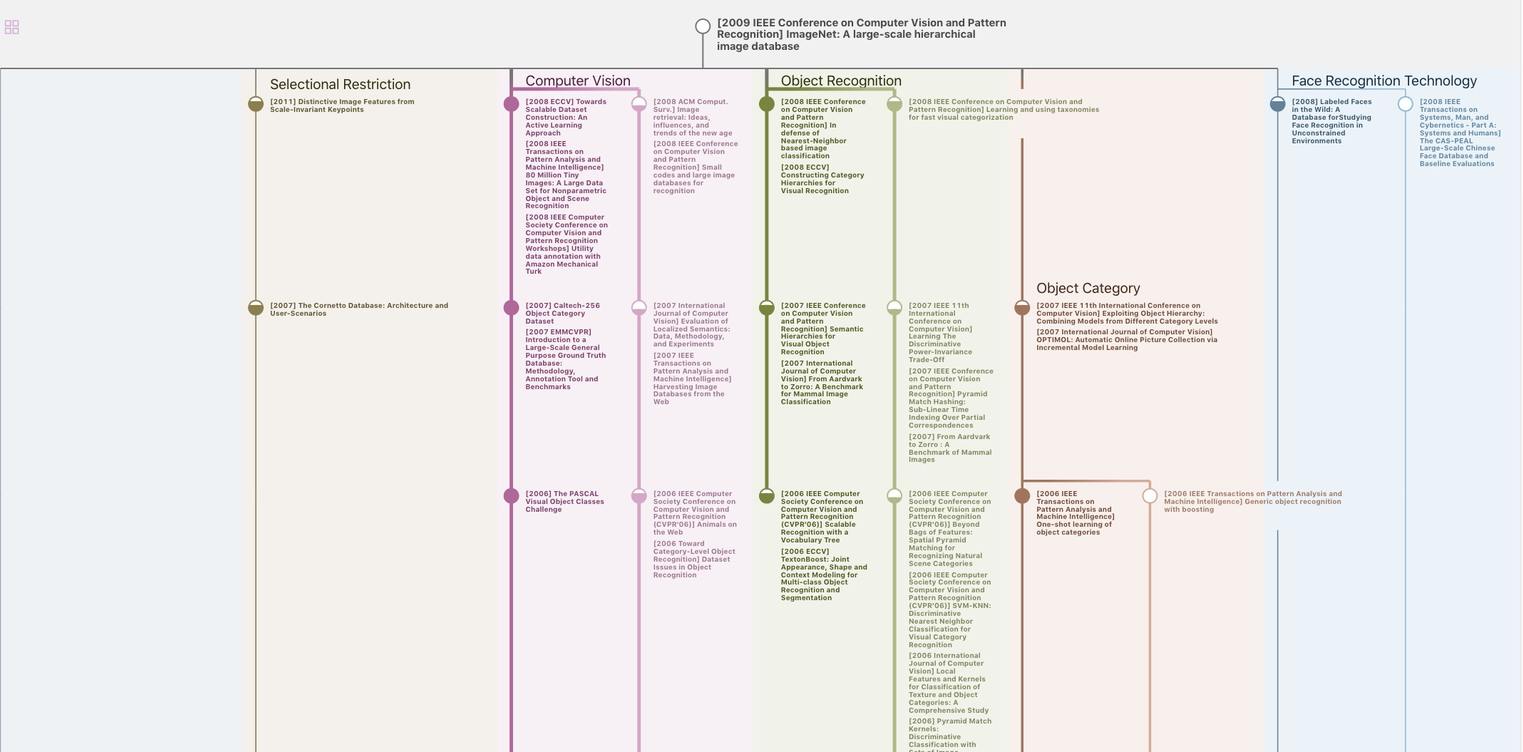
生成溯源树,研究论文发展脉络
Chat Paper
正在生成论文摘要