Improving Object Permanence using Agent Actions and Reasoning
2021 IEEE/RSJ INTERNATIONAL CONFERENCE ON INTELLIGENT ROBOTS AND SYSTEMS (IROS)(2021)
摘要
Object permanence in psychology means knowing that objects still exist even if they are no longer visible. It is a crucial concept for robots to operate autonomously in uncontrolled environments. Existing approaches learn object permanence from low-level perception, but perform poorly on more complex scenarios, like when objects are contained and carried by others. Knowledge about manipulation actions performed on an object prior to its disappearance allows us to reason about its location, e.g., that the object has been placed in a carrier. In this paper we argue that object permanence can be improved when the robot uses knowledge about executed actions and describe an approach to infer hidden object states from agent actions. We show that considering agent actions not only improves rule-based reasoning models but also purely neural approaches, showing its general applicability. Then, we conduct quantitative experiments on a snitch localization task using a dataset of 1,371 synthesized videos, where we compare the performance of different object permanence models with and without action annotations. We demonstrate that models with action annotations can significantly increase performance of both neural and rule-based approaches. Finally, we evaluate the usability of our approach in real-world applications by conducting qualitative experiments with two Universal Robots (UR5 and UR16e) in both lab and industrial settings. The robots complete benchmark tasks for a gearbox assembly and demonstrate the object permanence capabilities with real sensor data in an industrial environment.
更多查看译文
关键词
manipulation actions,hidden object states,considering agent actions,rule-based reasoning models,different object permanence models,action annotations,object permanence capabilities
AI 理解论文
溯源树
样例
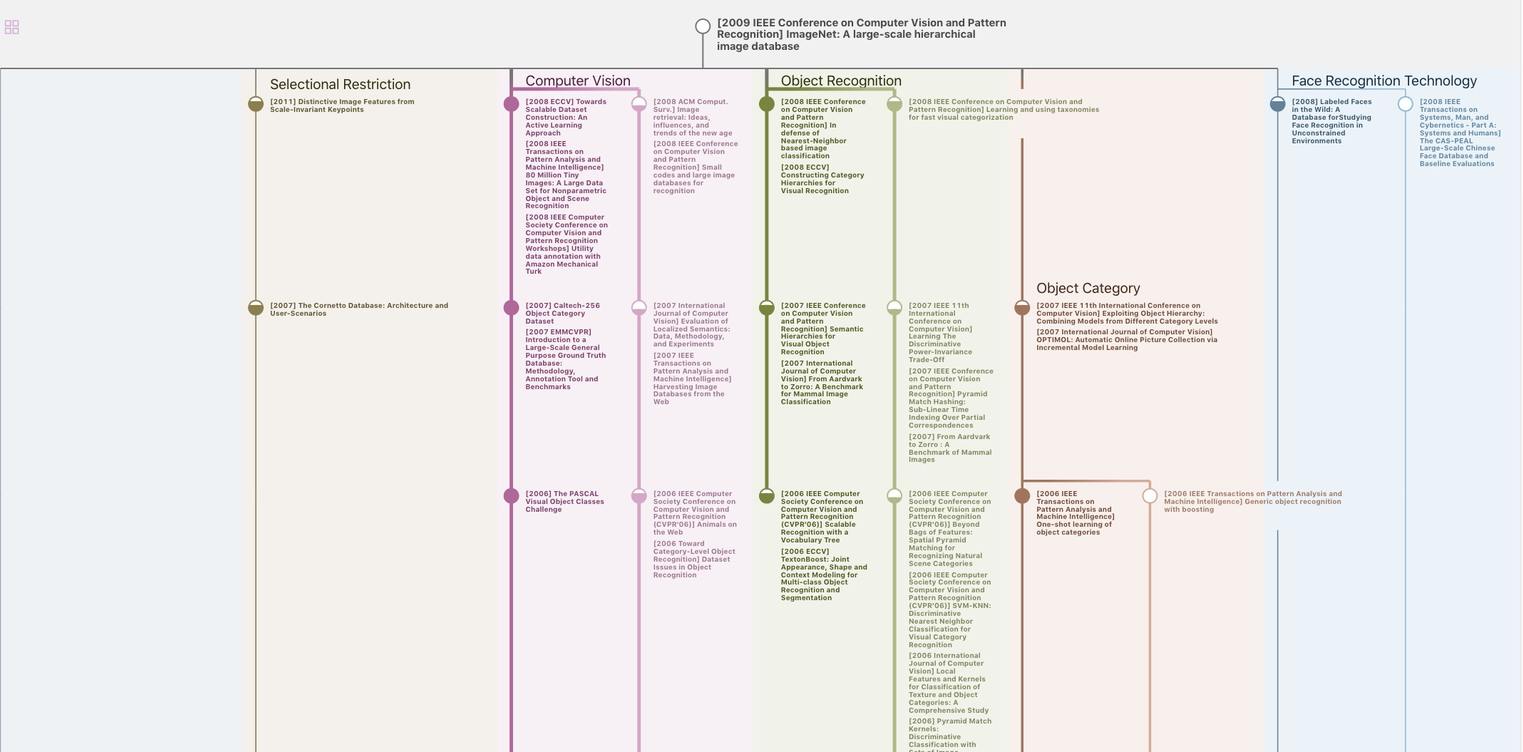
生成溯源树,研究论文发展脉络
Chat Paper
正在生成论文摘要