Deep Learning-Based Action Detection in Untrimmed Videos: A Survey
IEEE Transactions on Pattern Analysis and Machine Intelligence(2023)
摘要
Understanding human behavior and activity facilitates advancement of numerous real-world applications, and is critical for video analysis. Despite the progress of action recognition algorithms in trimmed videos, the majority of real-world videos are lengthy and untrimmed with sparse segments of interest. The task of temporal activity detection in untrimmed videos aims to localize the temporal boundary of actions and classify the action categories. Temporal activity detection task has been investigated in full and limited supervision settings depending on the availability of action annotations. This article provides an extensive overview of deep learning-based algorithms to tackle temporal action detection in untrimmed videos with different supervision levels including fully-supervised, weakly-supervised, unsupervised, self-supervised, and semi-supervised. In addition, this article reviews advances in spatio-temporal action detection where actions are localized in both temporal and spatial dimensions. Action detection in online setting is also reviewed where the goal is to detect actions in each frame without considering any future context in a live video stream. Moreover, the commonly used action detection benchmark datasets and evaluation metrics are described, and the performance of the state-of-the-art methods are compared. Finally, real-world applications of temporal action detection in untrimmed videos and a set of future directions are discussed.
更多查看译文
关键词
Action understanding,temporal action detection,untrimmed videos,deep learning,full and limited supervision
AI 理解论文
溯源树
样例
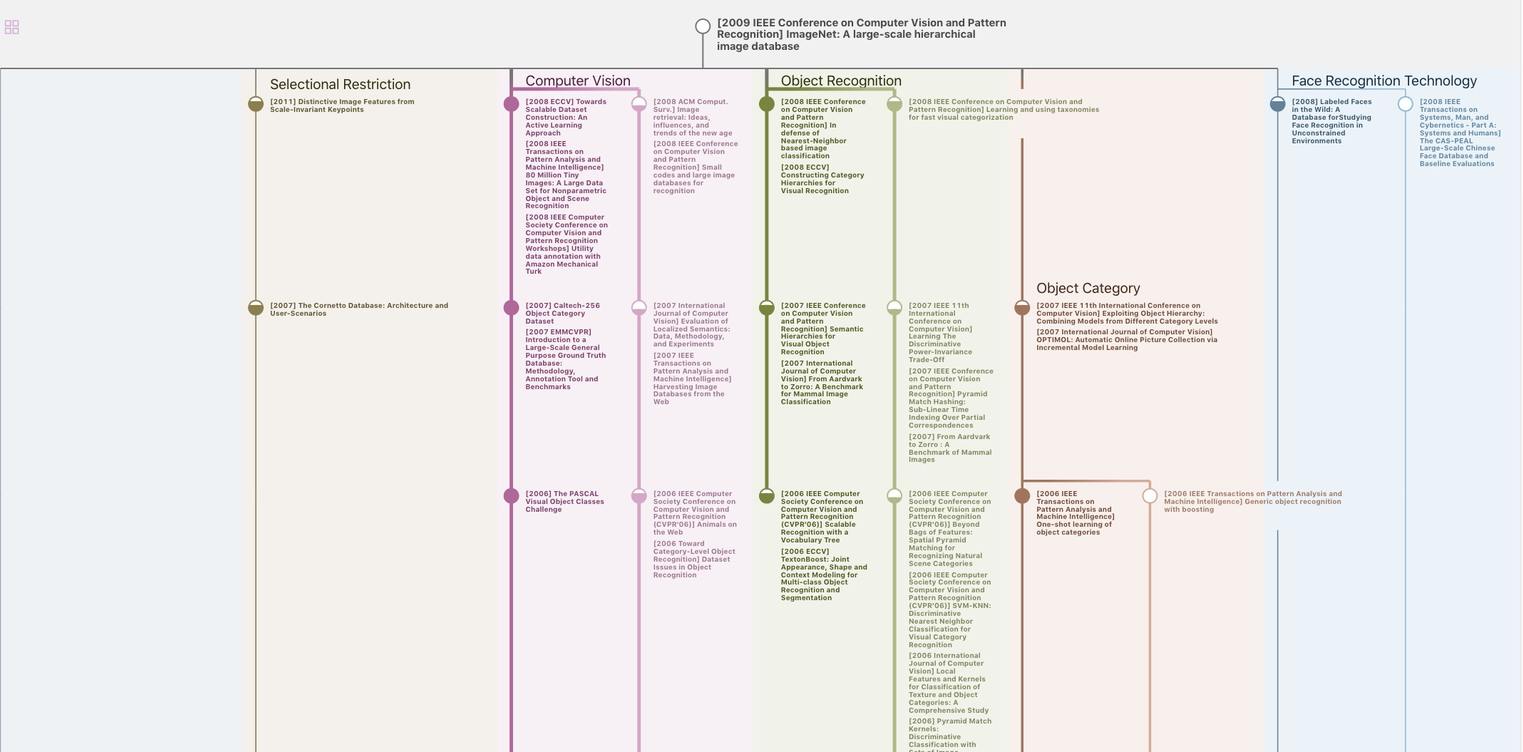
生成溯源树,研究论文发展脉络
Chat Paper
正在生成论文摘要