A Decision Rule Approach for Two-Stage Data-Driven Distributionally Robust Optimization Problems with Random Recourse
INFORMS JOURNAL ON COMPUTING(2023)
摘要
We study two-stage stochastic optimization problems with random recourse, where the coefficients of the adaptive decisions involve uncertain parameters. To deal with the infinite-dimensional recourse decisions, we propose a scalable approximation scheme via piecewise linear and piecewise quadratic decision rules. We develop a data-driven distributionally robust framework with two layers of robustness to address distributional uncertainty. We also establish out-of-sample performance guarantees for the proposed scheme. Applying known ideas, the resulting optimization problem can be reformulated as an exact copositive program that admits semidefinite programming approximations. We design an iterative decomposition algorithm, which converges under some regularity conditions, to reduce the runtime needed to solve this program. Through numerical examples for various known operations management applications, we demonstrate that our method produces significantly better solutions than the traditional sample-average approximation scheme especially when the data are limited. For the problem instances for which only the recourse cost coefficients are random, our method exhibits slightly inferior out-of sample performance but shorter runtimes compared with a competing approach.
更多查看译文
关键词
distributionally robust optimization,random recourse,piecewise decision rules,copositive programming decomposition algorithm
AI 理解论文
溯源树
样例
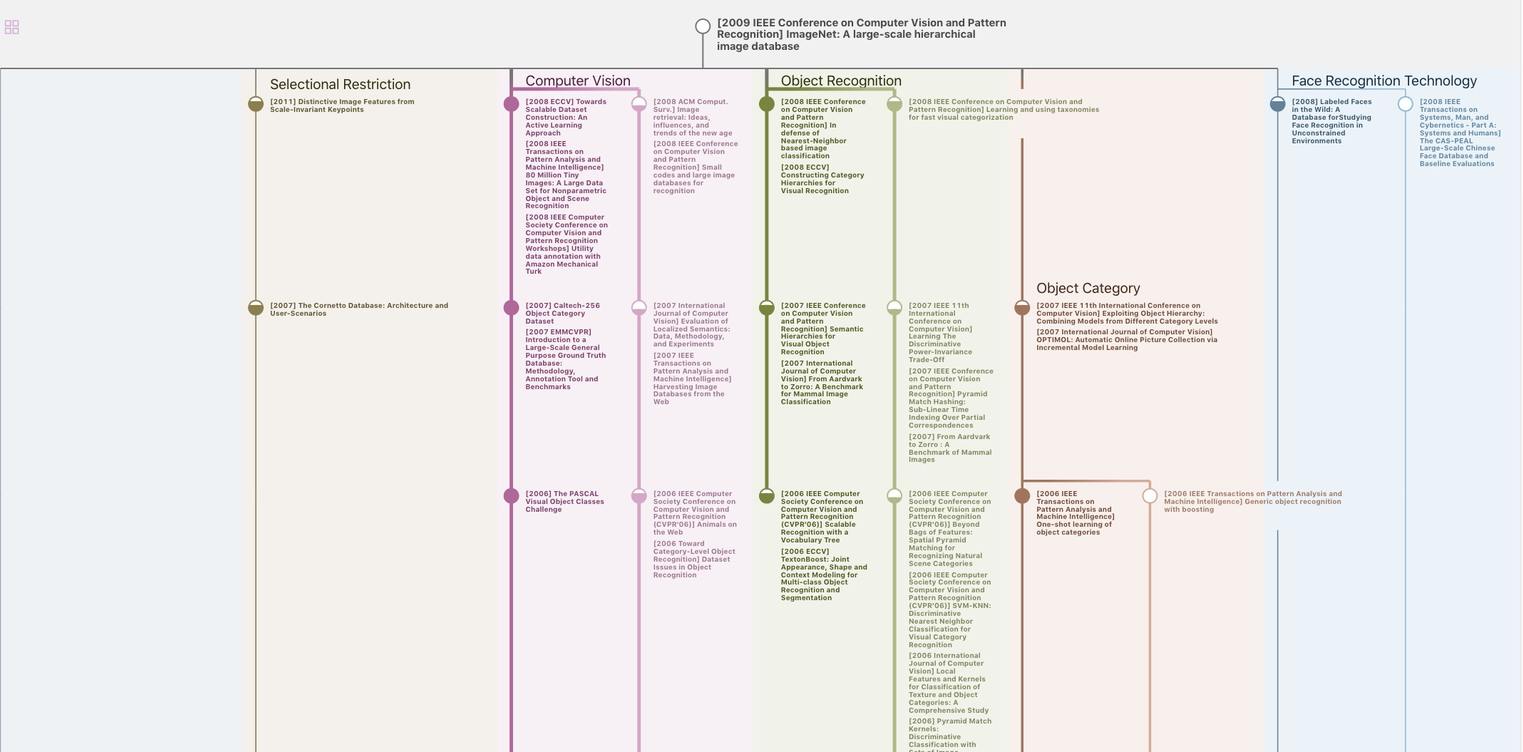
生成溯源树,研究论文发展脉络
Chat Paper
正在生成论文摘要