PIETS: Parallelised Irregularity Encoders for Forecasting with Heterogeneous Time-Series
2021 21ST IEEE INTERNATIONAL CONFERENCE ON DATA MINING (ICDM 2021)(2021)
摘要
Heterogeneity and irregularity of multi-source data sets present a significant challenge to time-series analysis. In the literature, the fusion of multi-source time-series has been achieved either by using ensemble learning models which ignore temporal patterns and correlation within features or by defining a fixed-size window to select specific parts of the data sets. On the other hand, many studies have shown major improvement to handle the irregularity of time-series, yet none of these studies has been applied to multi-source data. In this work, we design a novel architecture, PIETS, to model heterogeneous time-series. PIETS has the following characteristics: (1) irregularity encoders for multi-source samples that can leverage all available information and accelerate the convergence of the model; (2) parallelised neural networks to enable flexibility and avoid information over-whelming; and (3) attention mechanism that highlights different information and gives high importance to the most related data. Through extensive experiments on real-world data sets related to COVID-19, we show that the proposed architecture is able to effectively model heterogeneous temporal data and outperforms other state-of-the-art approaches in the prediction task.
更多查看译文
关键词
heterogeneous data sources, irregular time-series, sequences modeling, temporal data analysis
AI 理解论文
溯源树
样例
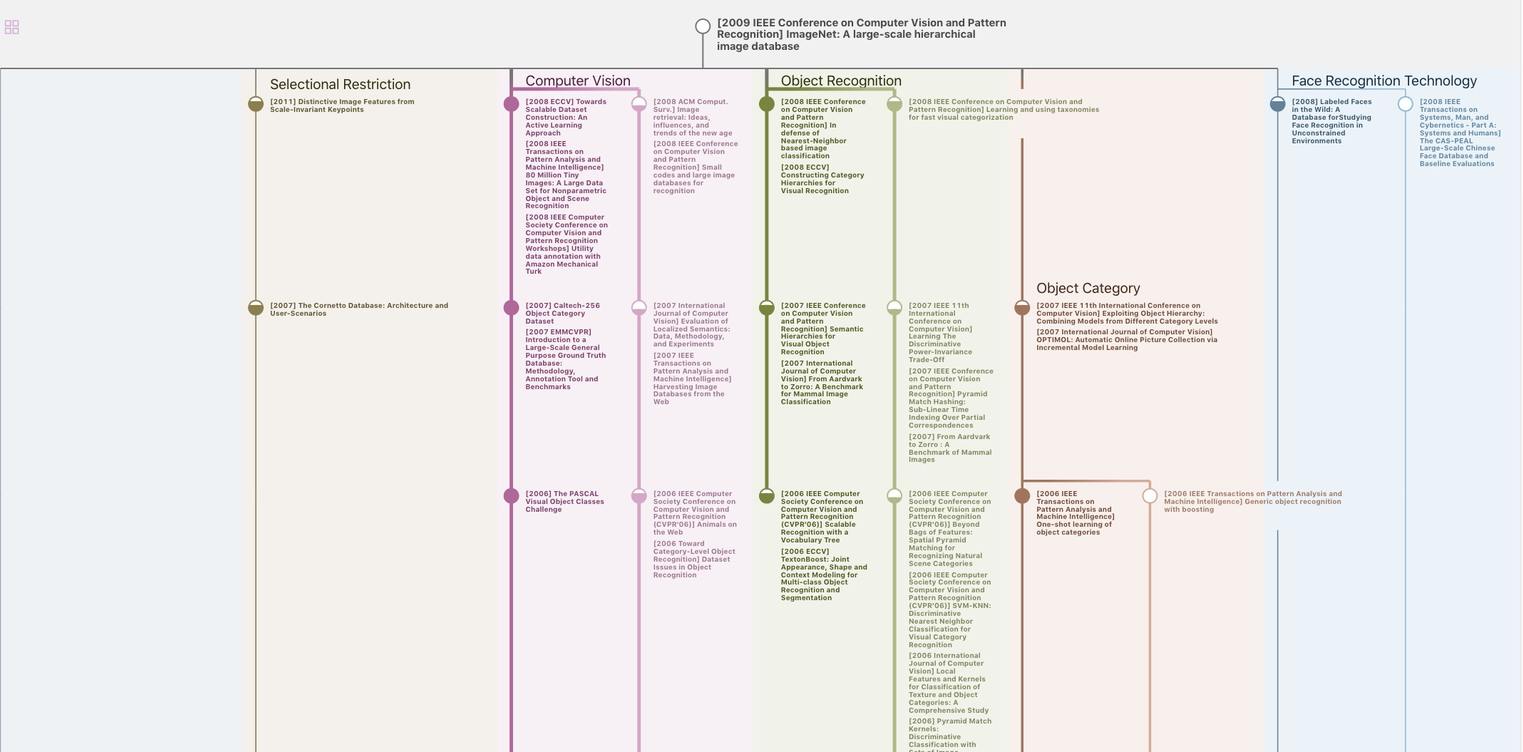
生成溯源树,研究论文发展脉络
Chat Paper
正在生成论文摘要