Dataset or Not? A Study on the Veracity of Semantic Markup for Dataset Pages
SEMANTIC WEB - ISWC 2021(2021)
摘要
Semantic markup, such as Schema.org, allows providers on the Web to describe content using a shared controlled vocabulary. This markup is invaluable in enabling a broad range of applications, from vertical search engines, to rich snippets in search results, to actions on emails, to many others. In this paper, we focus on semantic markup for datasets, specifically in the context of developing a vertical search engine for datasets on the Web, Google's Dataset Search. Dataset Search relies on Schema.org to identify pages that describe datasets. While Schema.org was the core enabling technology for this vertical search, we also discovered that we need to address the following problem: pages from 61% of internet hosts that provide Schema.org/Dataset markup do not actually describe datasets. We analyze the veracity of dataset markup for Dataset Search's Web-scale corpus and categorize pages where this markup is not reliable. We then propose a way to drastically increase the quality of the dataset metadata corpus by developing a deep neural-network classifier that identifies whether or not a page with Schema.org/Dataset markup is a dataset page. Our classifier achieves 96.7% recall at the 95% precision point. This level of precision enables Dataset Search to circumvent the noise in semantic markup and to use the metadata to provide high quality results to users.
更多查看译文
关键词
Datasets, Dataset search, Semantic markup
AI 理解论文
溯源树
样例
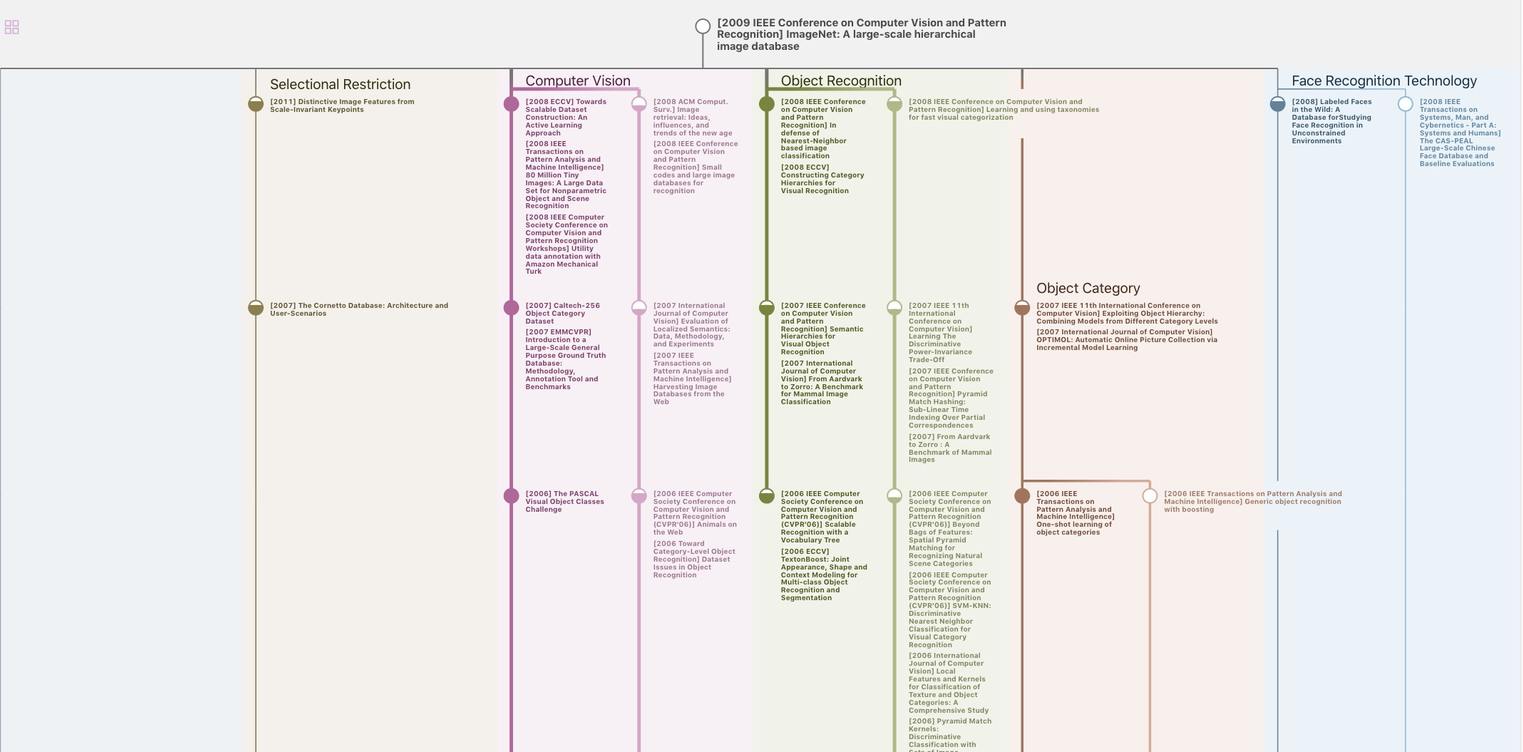
生成溯源树,研究论文发展脉络
Chat Paper
正在生成论文摘要