BlockFLow: Decentralized, Privacy-Preserving, and Accountable Federated Machine Learning
BLOCKCHAIN AND APPLICATIONS(2022)
摘要
Federated machine learning enables multiple clients to collectively train a machine learning model without sharing sensitive data. However, without proper accountability mechanisms, adversarial clients can weaken the collective model. BlockFLow is a fully decentralized, privacy-preserving, and accountable federated learning system. It introduces an Ethereum blockchain smart contract to coordinate a federated learning experiment and to hold clients accountable. BlockFLow rewards clients proportional to the quality of their individual contributions, does not reveal the underlying datasets, and is resilient to a minority of adversarial clients. Unlike existing systems, BlockFLow does not require a centralized test dataset, sharing of datasets between the clients, or any trusted entities. We evaluated BlockFLow on logistic regression models. Our results illustrate that BlockFLow successfully rewards honest clients and identifies adversarial clients. These results, along with blockchain costs that do not scale with model complexity, demonstrate the effectiveness of BlockFLow as an accountable federated learning system.
更多查看译文
关键词
Blockchain accountability, Federated machine learning
AI 理解论文
溯源树
样例
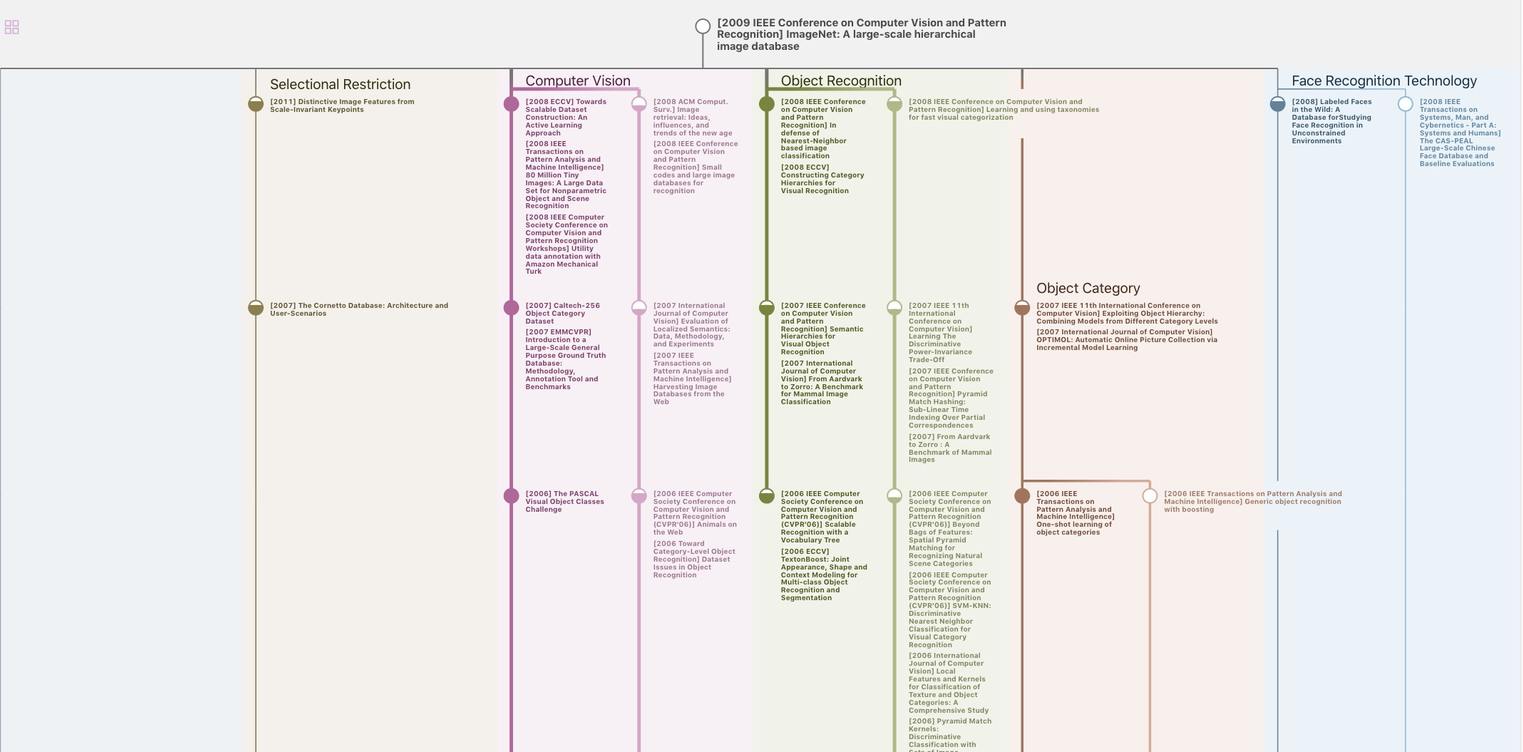
生成溯源树,研究论文发展脉络
Chat Paper
正在生成论文摘要