MetaTP: Traffic Prediction with Unevenly-Distributed Road Sensing Data via Fast Adaptation.
Proc. ACM Interact. Mob. Wearable Ubiquitous Technol.(2021)
摘要
With the popularity of smartphones, large-scale road sensing data is being collected to perform traffic prediction, which is an important task in modern society. Due to the nature of the roving sensors on smartphones, the collected traffic data which is in the form of multivariate time series, is often temporally sparse and unevenly distributed across regions. Moreover, different regions can have different traffic patterns, which makes it challenging to adapt models learned from regions with sufficient training data to target regions. Given that many regions may have very sparse data, it is also impossible to build individual models for each region separately. In this paper, we propose a meta-learning based framework named MetaTP to overcome these challenges. MetaTP has two key parts, i.e., basic traffic prediction network (base model) and meta-knowledge transfer. In base model, a two-layer interpolation network is employed to map original time series onto uniformly-spaced reference time points, so that temporal prediction can be effectively performed in the reference space. The meta-learning framework is employed to transfer knowledge from source regions with a large amount of data to target regions with a few data examples via fast adaptation, in order to improve model generalizability on target regions. Moreover, we use two memory networks to capture the global patterns of spatial and temporal information across regions. We evaluate the proposed framework on two real-world datasets, and experimental results show the effectiveness of the proposed framework.
更多查看译文
关键词
traffic prediction, meta learning
AI 理解论文
溯源树
样例
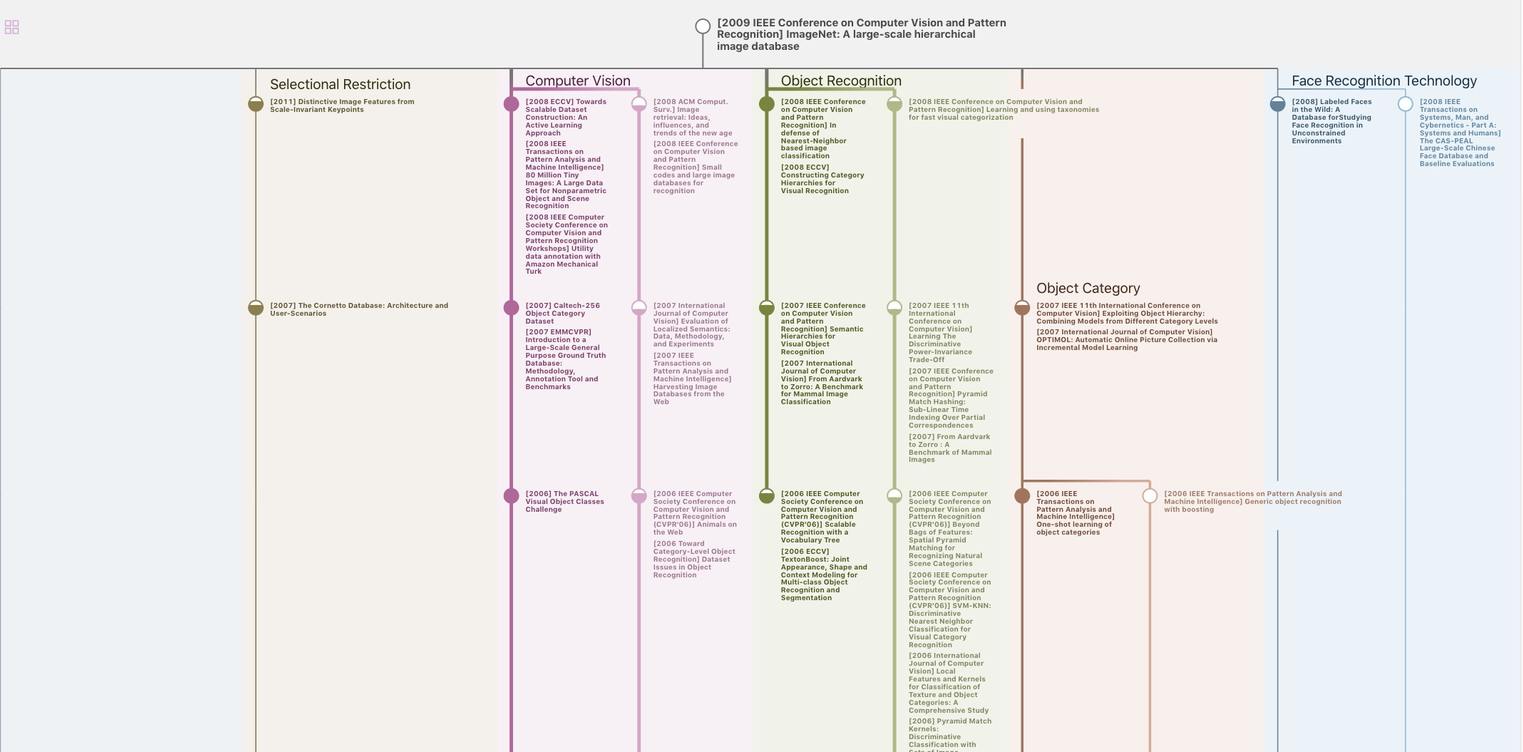
生成溯源树,研究论文发展脉络
Chat Paper
正在生成论文摘要