Audio Keyword Reconstruction from On-Device Motion Sensor Signals via Neural Frequency Unfolding.
Proc. ACM Interact. Mob. Wearable Ubiquitous Technol.(2021)
摘要
In this paper, we present a novel deep neural network architecture that reconstructs the high-frequency audio of selected spoken human words from low-sampling-rate signals of (ego-)motion sensors, such as accelerometer and gyroscope data, recorded on everyday mobile devices. As the sampling rate of such motion sensors is much lower than the Nyquist rate of ordinary human voice (around 6kHz+), these motion sensor recordings suffer from a significant frequency aliasing effect. In order to recover the original high-frequency audio signal, our neural network introduces a novel layer, called the alias unfolding layer, specialized in expanding the bandwidth of an aliased signal by reversing the frequency folding process in the time-frequency domain. While perfect unfolding is known to be unrealizable, we leverage the sparsity of the original signal to arrive at a sufficiently accurate statistical approximation. Comprehensive experiments show that our neural network significantly outperforms the state of the art in audio reconstruction from motion sensor data, effectively reconstructing a pre-trained set of spoken keywords from low-frequency motion sensor signals (with a sampling rate of 100-400 Hz). The approach demonstrates the potential risk of information leakage from motion sensors in smart mobile devices.
更多查看译文
关键词
Motion sensors, Deep learning, Time frequency analysis
AI 理解论文
溯源树
样例
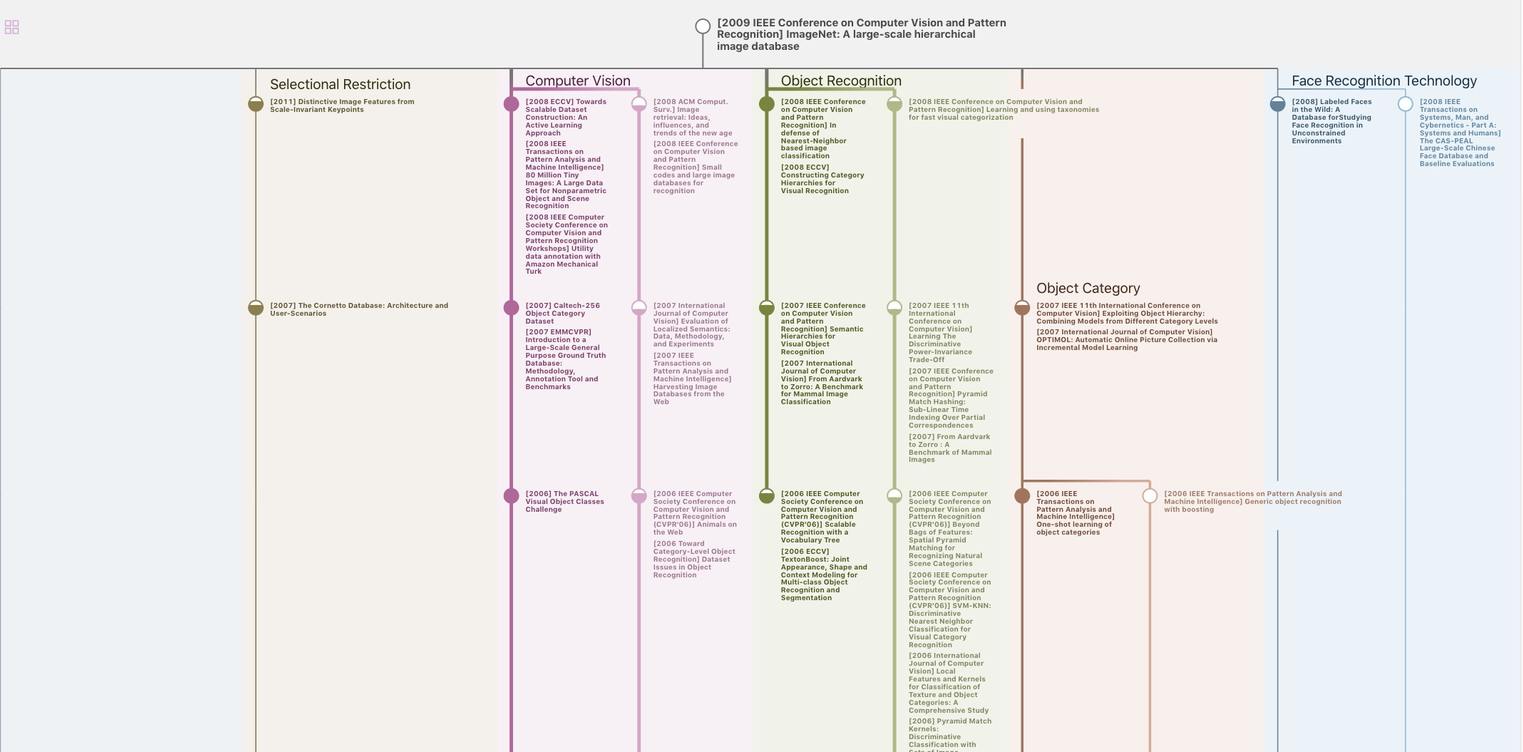
生成溯源树,研究论文发展脉络
Chat Paper
正在生成论文摘要