EDKE: Encoder-Decoder based Kernel Estimation for Blind Image Super-resolution
2021 INTERNATIONAL JOINT CONFERENCE ON NEURAL NETWORKS (IJCNN)(2021)
摘要
Deep neural networks (DNNs) have recently achieved wonderful results in single image super-resolution (SISR). These DNN-based SR methods are usually trained on supervised training datasets downscaled with a fixed kernel (e.g., bicubic), and suffer from the generalization problem on real world images due to the complexity of the degradation process. More recent works (e.g., KernelGAN [1]) use a generator network to realize kernel estimation from a single image. However, such methods neglect the anisotropy of kernel, thus limiting the estimation accuracy of kernel in practice. To address this issue, in this paper, we propose a novel encoder-decoder based kernel estimation (EDKE) framework by considering the anisotropy of kernel. In our EDKE framework, the input image is first fed into an encoder network to learn the degradation process, followed by a decoder network to map the encoder image into the original image itself. In this way, the encoder image contains more compact information for the degradation kernel. Besides, our EDKE can be plugged into other blind SR methods. Extensive experiments demonstrate the effectiveness of our method in kernel estimation, and show the superiority of our method over other state-of-the-art methods.
更多查看译文
关键词
Super-Resolution, Blind Super Resolution, Kernel Estimation
AI 理解论文
溯源树
样例
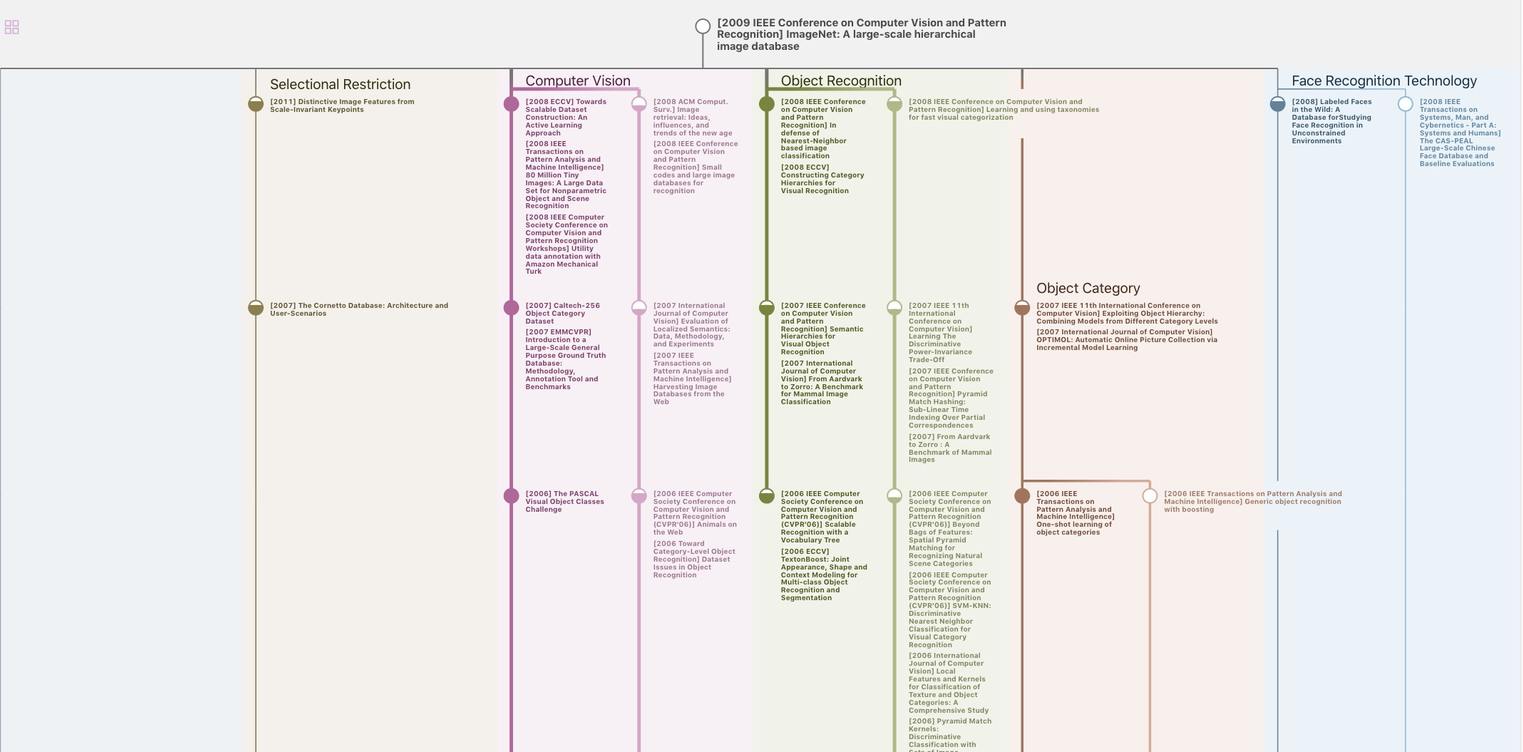
生成溯源树,研究论文发展脉络
Chat Paper
正在生成论文摘要