Curvilinear Collaborative Metric Learning with Macro-micro Attentions
2021 INTERNATIONAL JOINT CONFERENCE ON NEURAL NETWORKS (IJCNN)(2021)
摘要
Although matrix factorization and its variants have proved their effectiveness in learning user preferences, they violate the triangle inequality and may fail to capture the inner grained preference information. Thus, metric learning based models have attracted increasing interests in recommender systems. However, most of these models only measure the distance in Euclidean space, though it is known to limit the prediction performance. To address this problem, this paper proposes curvilinear collaborative metric learning (CCML) as a solution. Rather than learning from Euclidean distance, CCML measures the "path" (i.e. curvilinear distance) between the user and items. Therefore, it is able to better infer information (in geometrical representation) and user-item relations behind the observed data points. Under this setting, the relations between the user and items are not linearly reflected by the relation vector. In order to effectively learn the representation of items, we propose to fuse macro features of categories and micro representations of items to form their latent vectors. Our model can be less prone to be affected by the noise commonly presented in datasets by separately learning macro and micro features. Experimental results on eight public recommendation datasets demonstrate that CCML produces a outstanding performance compared with five competitive baselines. Finally, quantitative analysis is included to verify the efficiency and effectiveness of CCML and the macro-micro module.
更多查看译文
关键词
matrix factorization,macromicromodule,recommender systems,macromicroattentions,user preferences,user-item relations,Euclidean distance,CCML,curvilinear collaborative metric learning,inner grained preference information
AI 理解论文
溯源树
样例
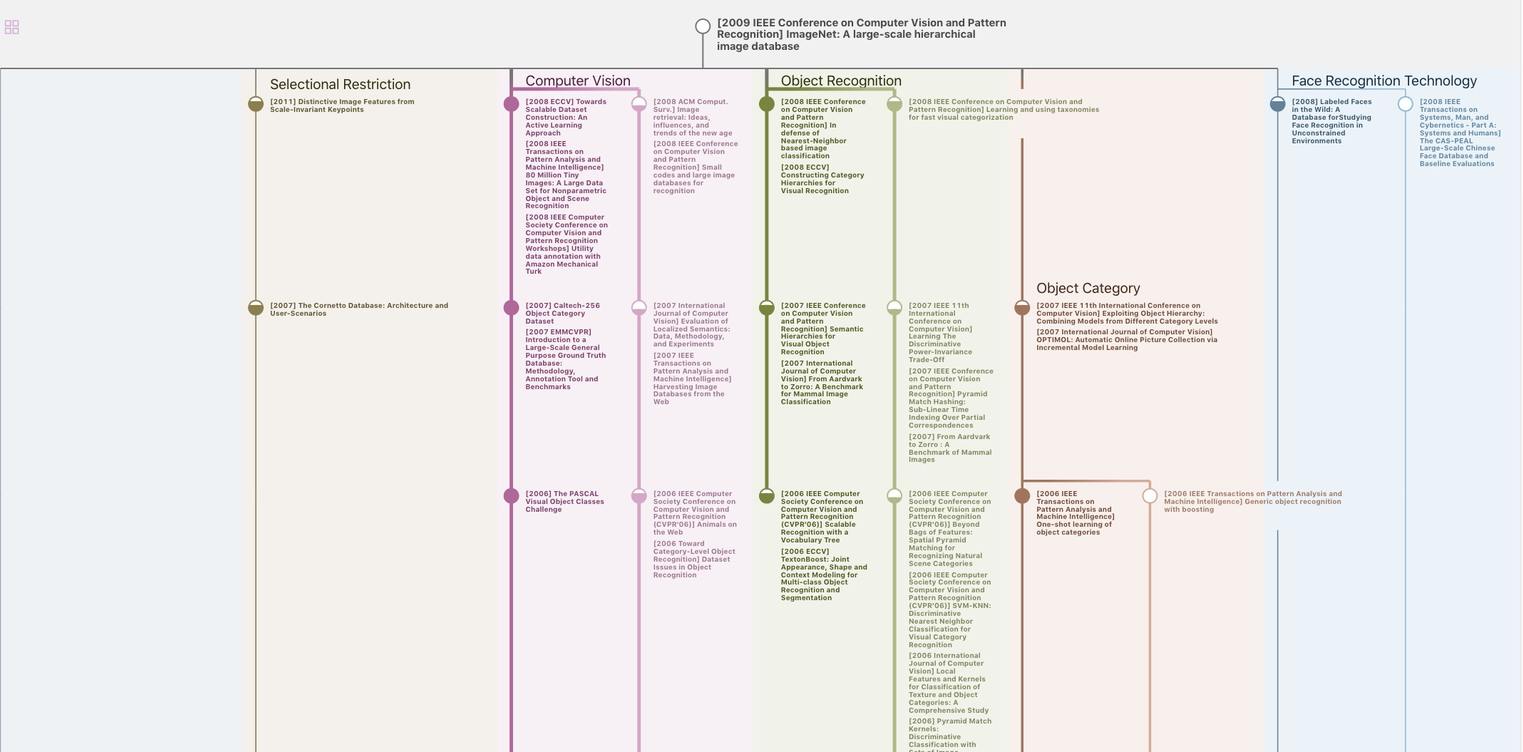
生成溯源树,研究论文发展脉络
Chat Paper
正在生成论文摘要