GKT-CD: Make Cognitive Diagnosis Model Enhanced by Graph-based Knowledge Tracing
2021 INTERNATIONAL JOINT CONFERENCE ON NEURAL NETWORKS (IJCNN)(2021)
摘要
Recent advancements in online education platforms have caused an increase in research on adaptive learning system, wherein student performance on coursework exercises is predicted over time and directed exercises are recommended. In adaptive learning systems, knowledge tracing and cognitive diagnosis are critical techniques for predicting student performance. The traditional cognitive diagnosis model's terms are suitable for the student abilities analysis, but they rely on handcrafted interaction functions and only use student's response records so that it is difficult to capture the dynamic knowledge mastery ability of students. Although using the knowledge tracing to enhance cognitive diagnosis is a meaningful attempt towards towards capturing student performance, the RNN-based knoweledge tracing model have limited effect. This paper proposes a new model, named GKT-CD, which fuses knowledge tracing and cognitive diagnosis in a synergistic framework. In GKTCD, we develop Gated-GNN to trace the student-knowledge response records and extract students' latent trait. And then, we use hierarchical structure in knowledge to construct exercise latent vector. At last, we use two-dimensional item response theory (IRT) to predict the probability of students answering exercises correctly. Extensive experiments conducted on real-world datasets show that the GKT-CD model is feasible and obtain excellent performance.
更多查看译文
关键词
Adaptive Learning System, Cognitive Diagnosis, Item Response Theory, Knowledge Tracing, Graph Neural Network
AI 理解论文
溯源树
样例
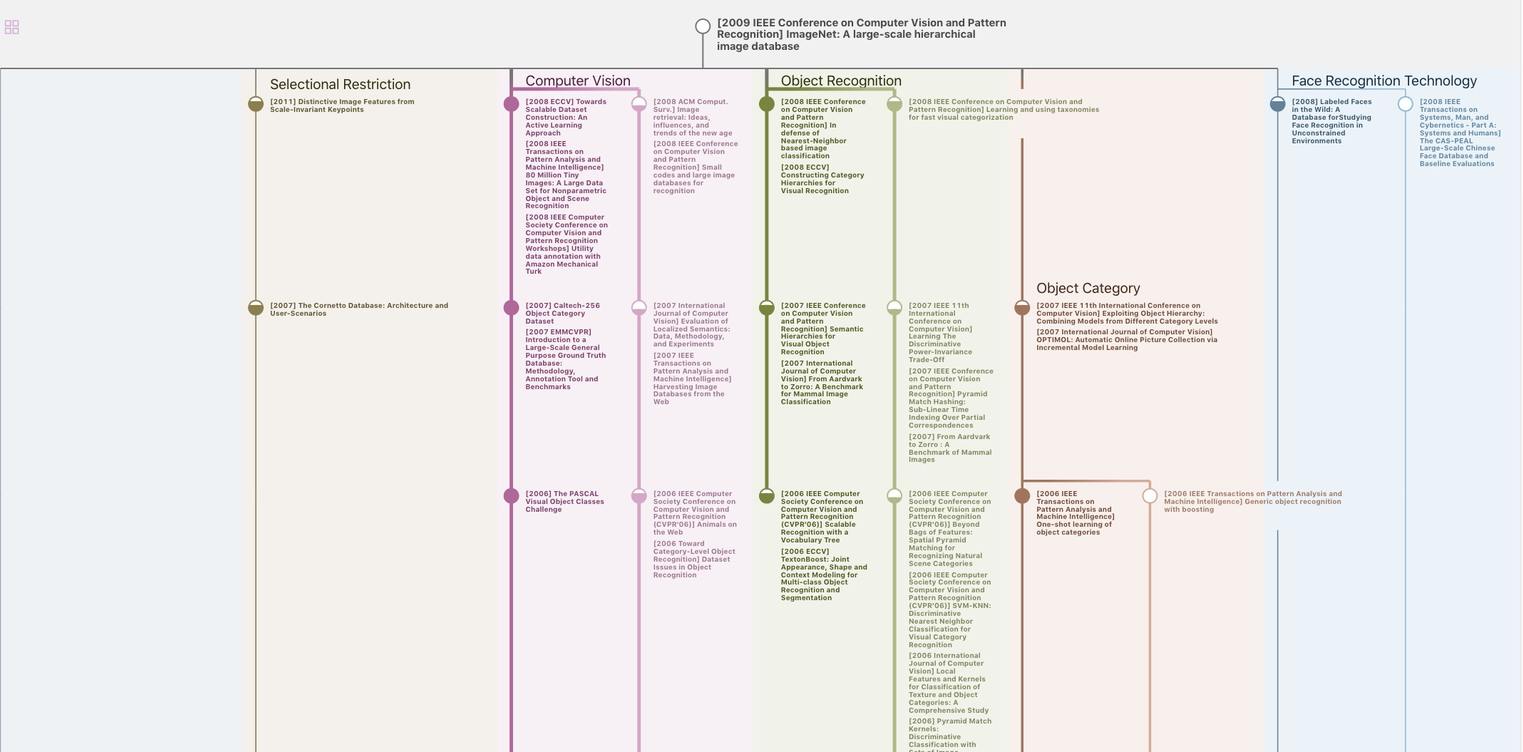
生成溯源树,研究论文发展脉络
Chat Paper
正在生成论文摘要