Intragroup sparsity for efficient inference
2021 INTERNATIONAL JOINT CONFERENCE ON NEURAL NETWORKS (IJCNN)(2021)
摘要
This work studies intragroup sparsity, a fine-grained structural constraint on network weight parameters. It eliminates the computational inefficiency of fine-grained sparsity due to irregular dataflow, while at the same time achieving high inference accuracy. We present theoretical analysis on how weight group sizes affect sparsification error, and on how the performance of pruned networks changes with sparsity level. Further, we analyze inference-time I/O cost of two different strategies for achieving intragroup sparsity and how the choice of strategies affect I/O cost under mild assumptions on accelerator architecture. Moreover, we present a novel training algorithm that yield models of improved accuracies over the standard training approach under the intragroup sparsity constraint.
更多查看译文
关键词
Deep learning, Sparse Neural network, Model pruning, Lightweight neural network, Convolutional neural network
AI 理解论文
溯源树
样例
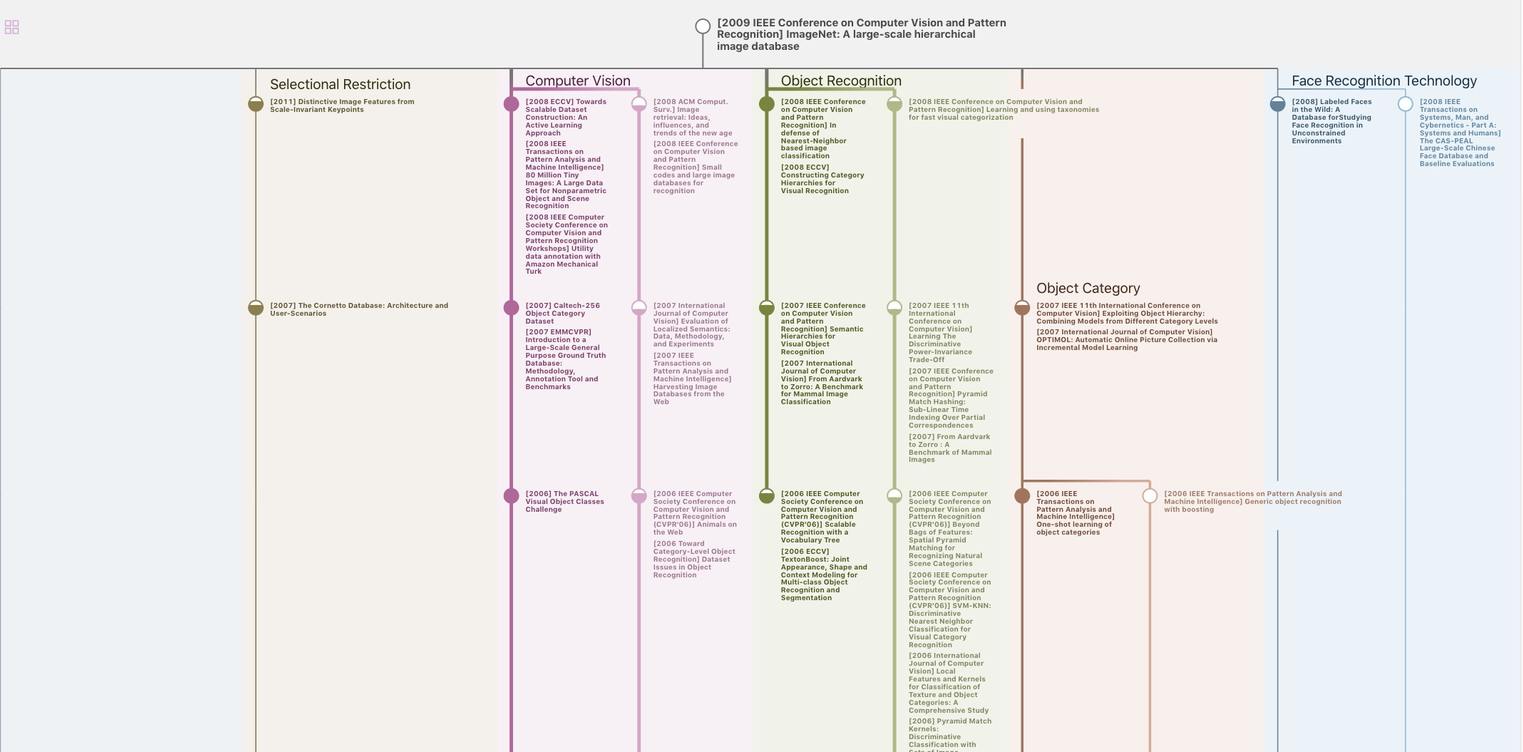
生成溯源树,研究论文发展脉络
Chat Paper
正在生成论文摘要