Word Sense Disambiguation based on Sequence Topic Model using sense dependency
2021 INTERNATIONAL JOINT CONFERENCE ON NEURAL NETWORKS (IJCNN)(2021)
摘要
Word sense disambiguation is a challenging task that aims to distinguish the correct sense for a target word. Unlike typical methods which only use the context information, we present the basic idea that incorporates the global sense distribution and contextual sense dependency to determine the correct sense. In this paper, we leverage the generative process of the sequence topic model to model the sense distribution from the whole global corpus directly. Since contextual sense dependency is more likely to happen between successive words, we hypothesize that the sense assignment of the target word depends on the sense of the previous word. Hence, the sense dependency could be modeled by the probabilistic topic model with hidden Markov chain assumption. Furthermore, the information in the sense inventory of WordNet is used as prior knowledge for obtaining the non-uniform sense distribution over words. It is notable that the prior knowledge contributes to parameter learning and inference during the Gibbs Sampling procedure, hence we call the proposed method a knowledge-based unsupervised approach. We evaluate the proposed method on Senseval-2, Senseval-3, SemEval-2007, SemEval-2013, and SemEval-2015 English All-Word WSD datasets and the experimental results show that although the performance of the proposed method is restricted by the sparse problem, it still achieves comparable performance compared with the state-of-the-art knowledge-based approaches.
更多查看译文
关键词
word sense disambiguation, sense dependency, sequence topic model, Markov chain
AI 理解论文
溯源树
样例
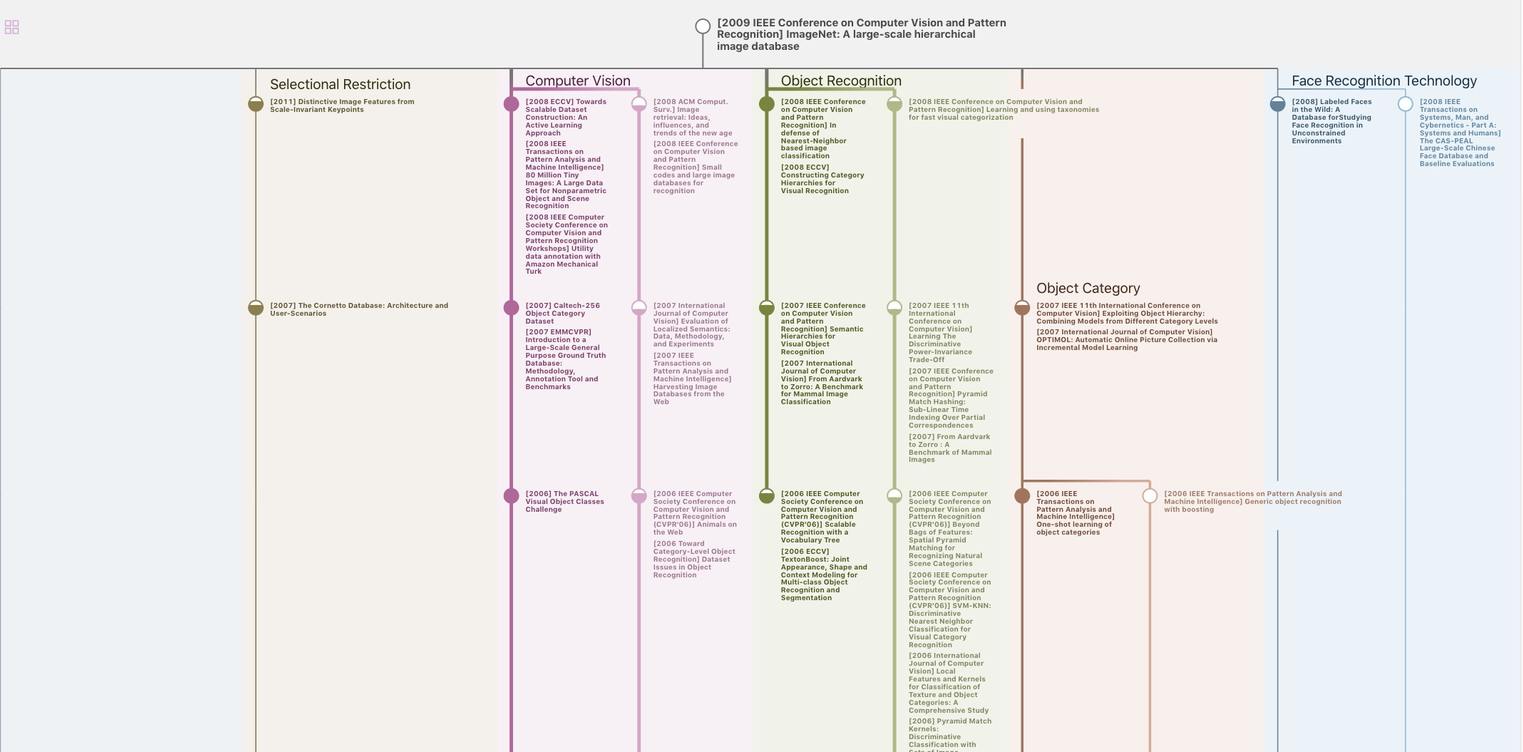
生成溯源树,研究论文发展脉络
Chat Paper
正在生成论文摘要