Personalized Session-Based Recommendation Using Graph Attention Networks
2021 INTERNATIONAL JOINT CONFERENCE ON NEURAL NETWORKS (IJCNN)(2021)
摘要
Personalized session-based recommendation systems aim to predict the next items that a user would be interested in based on previously recorded user interactions. The task contains two connotations. Firstly, “personalized” indicates the recommendation generating process does not involve other users. A recommendation system is expected to capture the preference patterns of the target user only. Secondly, “session-based” signifies that the preference patterns are expressed by sequences of items or interactions. Personalized session-based recommendation technologies are of high practical values in many application areas as explosive volumes of information available for customers. In this research we model user-item sessions using graphs and propose a novel graph neural network based model, namely, Personalized Session-based Recommendation using Graph Attention Networks (PSR-GAT). The PSR-GAT makes preferred item predictions by exploiting not only instantaneous preference patterns in the current session, but also more generic preference patterns in users' historical records. The proposed PSR-GAT is built on an attention mechanism basis with a consideration of item transition linkage. PSR-GAT does not have assumptions of preference expressions in sessions. Extensive experiments are conducted to evaluate the PSR-GAT model using three datasets, one is a vehicle infotainment dataset provided by the Ford Motor Company, and the other two are publicly accessible datasets. The performances of the PSR-GAT are compared with seven state-of-art methods for session-based recommendations. The results attest to the effectiveness and advantages of the PSR-GAT in the personalized session-based next interested items prediction.
更多查看译文
关键词
recommendation system, session-based recommendation, graph neural network, attention mechanism
AI 理解论文
溯源树
样例
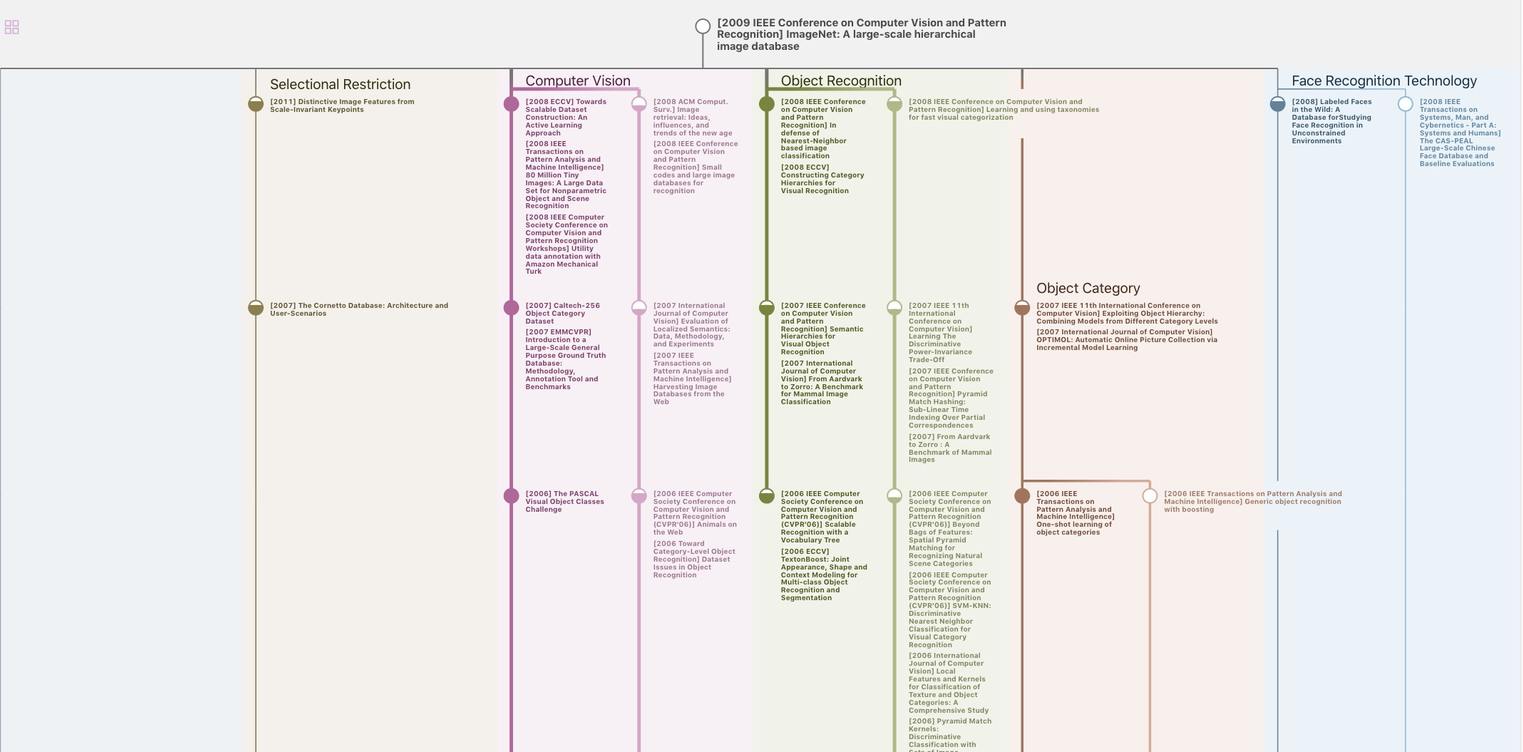
生成溯源树,研究论文发展脉络
Chat Paper
正在生成论文摘要