DCA-CLA: A scRNA-seq Classification Framework based on Deep Count Autoencoder
2021 INTERNATIONAL JOINT CONFERENCE ON NEURAL NETWORKS (IJCNN)(2021)
摘要
Identifying cell types is crucial for single-cell RNA sequencing (scRNA-seq) analysis and can be potentially utilized to understand high-level biological processes. Supervised models based on neural networks have recently been successfully applied in the scRNA-seq cell type classification problem and achieved promising results. While most existing works directly use the raw or transformed data, we argue that the original data are too sparse and high-dimensional, and extracting their effective low-dimensional features can better train downstream classifiers, thereby improving the cell type classification performance. In this paper, we propose a novel framework, named Deep Count Autoencoder-based Classifier (DCA-CLA), to leverage the discriminative low-dimensional features for classification. Specifically, DCA-CLA first denoises the original count matrix and extracts the data features from the hidden layer using a deep count autoencoder module, then it feeds these bottleneck features into the classifier network to train the learnable parameters and test the performance. Experimental results on eight separate datasets and four pairs of datasets demonstrate that the proposed DCA-CLA framework achieves competitive performance over the state-of-the-art frameworks.
更多查看译文
关键词
Denoising autoencoder, single-cell RNA sequencing classification, supervised learning, bioinformatics
AI 理解论文
溯源树
样例
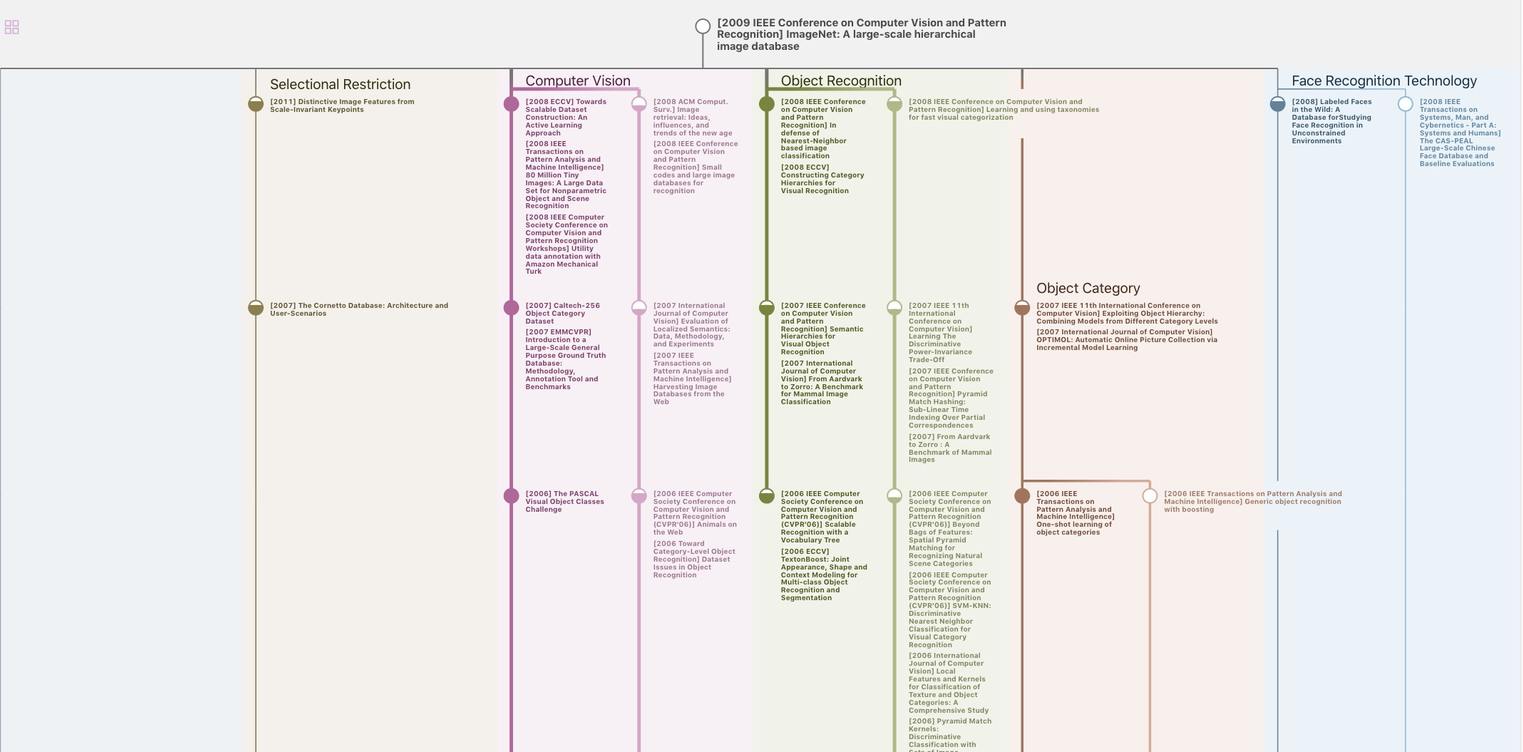
生成溯源树,研究论文发展脉络
Chat Paper
正在生成论文摘要