Hint Pyramid Learning Via Salient Semantic Mining
2021 INTERNATIONAL JOINT CONFERENCE ON NEURAL NETWORKS (IJCNN)(2021)
摘要
Feature pyramid network (FPN) has dominated the task of object detection for many years with its congenital capability of semantic information interaction between different feature levels. Satisfactory performance can be easily achieved via the heavy backbones or tricky designs of anchors. However, the heavy structure brings about a huge increase of parameters and calculation. To address this challenge, this paper proposes a novel Hint Pyramid Learning(HPL) strategy to optimize a lightweight FPN-based detector such as one based on ResNet18. In HPL, the hint feature representation is learnt via salient semantic mining based on the teacher-student learning mechanism and self learning mechanism. Besides minimizing of the discrepancy of features belonging to the same feature pyramid layer, HPL also focuses on the relationships between feature pyramid layers. Unlike the popular mimicking algorithms such as hint learning and knowledge distillation which achieve comparable performance on classification tasks but failed to generalize to the detection tasks with FPN, HPL can be easily plugged into different FPN-based pipelines such as one-stage RetinaNet or two-stage Faster R-CNN and improves the performance straightforwardly. Without any meticulous designing, when the backbone of students is ResNet18 and the backbone of teachers is ResNet50, HPL effectively improves the performance by 2.1 AP for RetinaNet and 1.0 AP for Faster R-CNN on COCO benchmark.
更多查看译文
关键词
Hint Learning, Knowledge Distillation, Feature Pyramid Network, Object Detection, Deep Learning
AI 理解论文
溯源树
样例
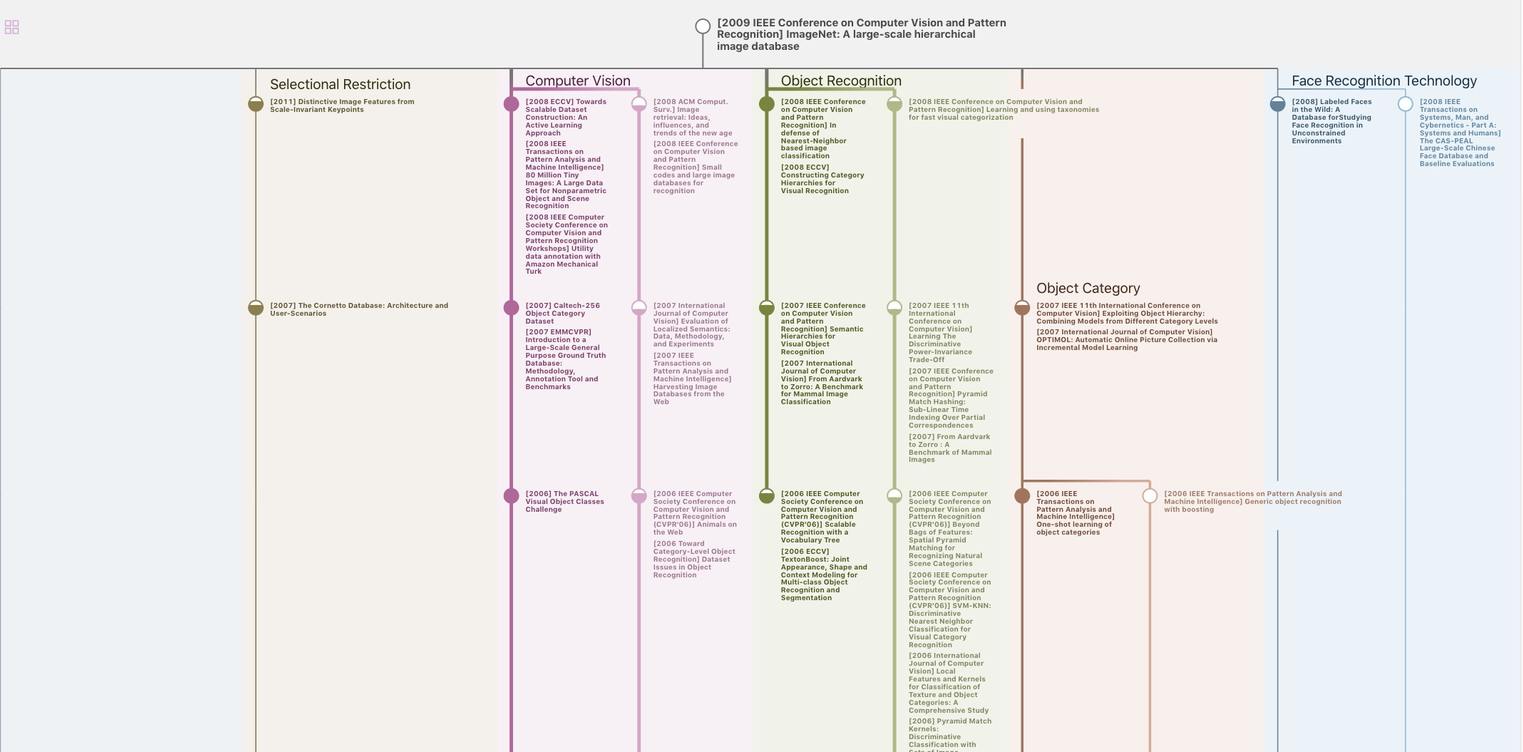
生成溯源树,研究论文发展脉络
Chat Paper
正在生成论文摘要