VDGAN: A Collaborative Filtering Framework Based on Variational Denoising with GANs
2021 INTERNATIONAL JOINT CONFERENCE ON NEURAL NETWORKS (IJCNN)(2021)
摘要
Generative Adversarial Networks (GANs) effectively capture the true posterior distribution. When applied to Collaborative Filtering (CF), GANs can generate a recommendation list through implicit feedback. However, the discriminators in the existing GANs-based CF methods are not utilized fully, and the generators perform poorly on sparse data mining. In this paper, we propose an improved collaborative filtering framework based on variational denoising for GANs (VDGAN). Specifically, VDGAN integrates the variational encoder and the self-attention mechanism into the GANs. By using the positive-negative sampling mechanism to add specific noise to the input data, the variational encoder obtains a robust feature matrix and improves the sparse data processing capability of the generator. In VDGAN, the denoising generator reconstructs the user-items interaction matrix through the feature matrix. And the discriminator is composed of the self-attention mechanism to obtain the explicit features of user preferences, which extends the ability of the discriminator. Furthermore, reinforcement learning replaces the traditional objective function of GANs, which better optimizes the generator and further improves the recommendation accuracy of the model. From our comprehensive experiments on three real-world datasets, we demonstrate that the performance of VDGAN significantly outperforms the state-of-the-art methods based on GANs and Auto-Encoders.
更多查看译文
关键词
collaborative filtering, generative adversarial networks, variational autoencoders, self-attention, reinforcement learning
AI 理解论文
溯源树
样例
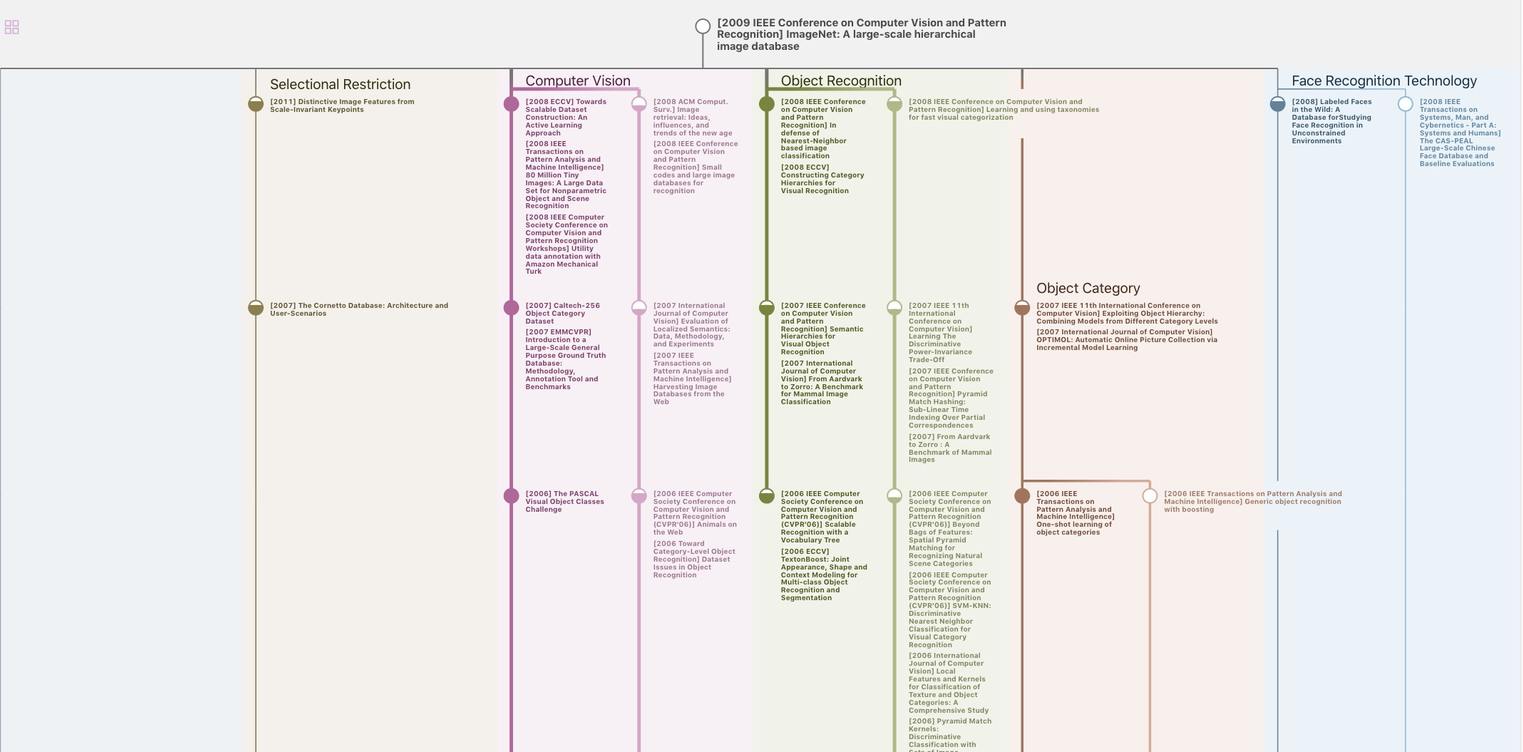
生成溯源树,研究论文发展脉络
Chat Paper
正在生成论文摘要