Topic Enhanced Multi-head Co-Attention: Generating Distractors for Reading Comprehension
2021 INTERNATIONAL JOINT CONFERENCE ON NEURAL NETWORKS (IJCNN)(2021)
摘要
In the construction of multiple-choice Machine Reading Comprehension(MRC), in addition to questions and answers, it is necessary to generate distractors corresponding to them. Recent models based on Seq2Seq have shown good results in text generation, while previous work has only succeeded in producing a few interfering words or phrases per question. Our goal is to generate more meaningful distractors based on reading comprehension of articles that are closer to the semantics of the question to help better diagnose gaps in text understanding. A major drawback of recent studies is that they do not take the relationship between the distractors and background text into account when generating distractors. This often results in the distractors being either too general or too close to the correct answer. We propose a Topic Enhanced Multi-head Co-Attention model (TMCA) based on hierarchical networks to better capture the interactions between sentences. By adding query-relevance loss, our model enables the distractors to be as semantically relevant to the question as possible based on the reading comprehension of the article, while trying to ensure that they are false answers. The results show that the proposed approach achieves superior performance over the baselines in terms of automatic metrics on two multiple-choice datasets (RACE and DREAM). For further evaluation, we use another advanced reading comprehension model and human evaluation to demonstrate that our model outperforms several strong baselines in generating high-quality and educationally meaningful distracters.
更多查看译文
关键词
Natural language processing, Distractor generation, Attention mechanism, Reading comprehension
AI 理解论文
溯源树
样例
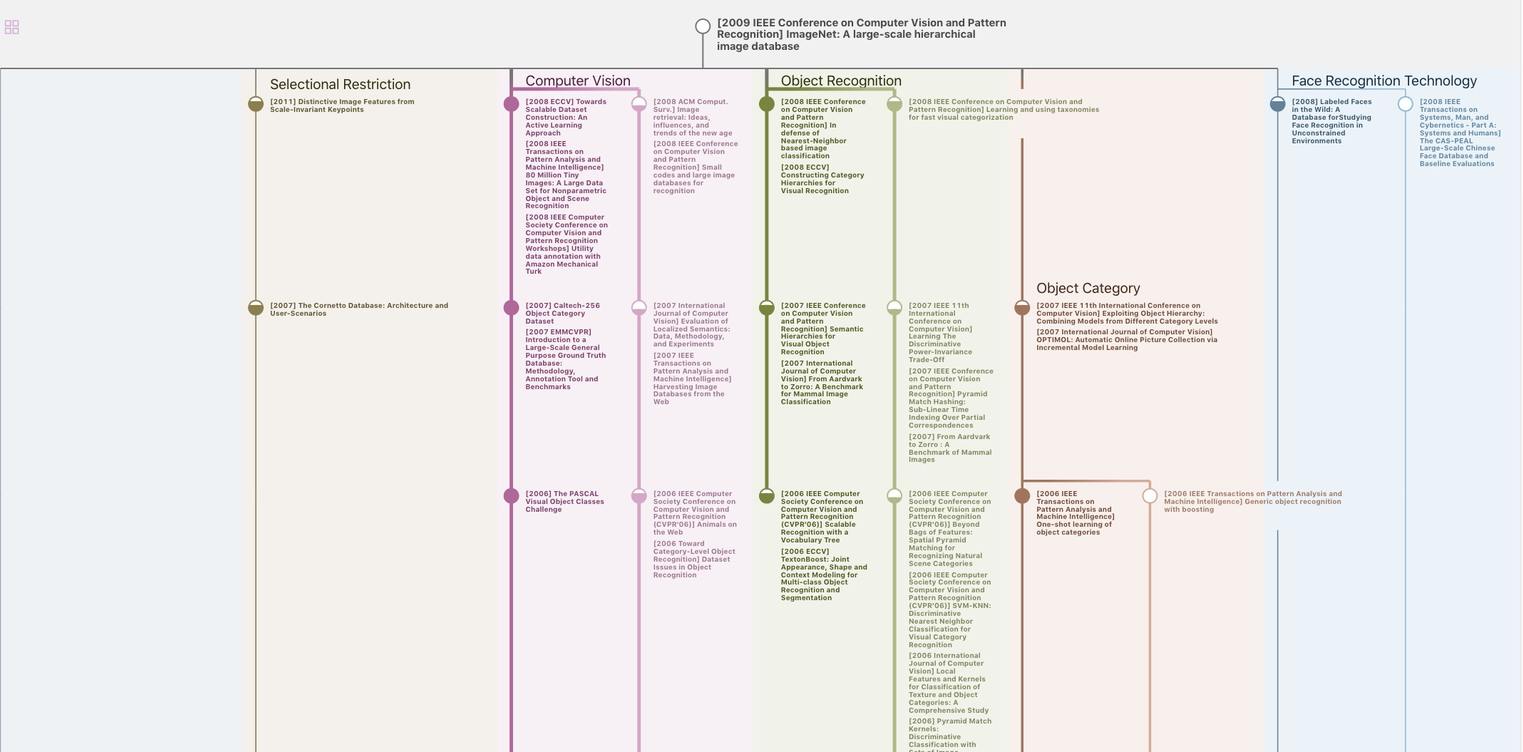
生成溯源树,研究论文发展脉络
Chat Paper
正在生成论文摘要