Improving Real Age Estimation from Apparent Age Data
2021 INTERNATIONAL JOINT CONFERENCE ON NEURAL NETWORKS (IJCNN)(2021)
摘要
Recent advancements in facial analysis have contributed to the proliferation of applications that use technology for automatic face recognition, detection, and the extraction of useful information. In this context, the problem of automatically estimating the chronological (real) age of a person, given a picture of her face, emerges as a current hot topic. Although very popular across a wide range of applications, the problem remains challenging, especially due to the high variability of the aging process in humans. In this work, we introduce novel techniques that leverage apparent age estimation data to improve real age estimation. The estimation of apparent age is a related problem that aims to guess "how old does a person look like" rather than focusing on the real age. Given a dataset of face images, in which images are annotated with both real and apparent ages, we developed ways to combine statistical methods and convolutional neural networks to improve the current state-of-the-art on real age estimation. Our proposed methods are able to achieve an average MAE (mean absolute error) of 4.847, outperforming at least by 8.5% state-of-the-art methods when experimenting with the publicly available APPA-REAL dataset.
更多查看译文
关键词
Age Estimation, Convolutional Neural Network, Computer Vision, Computer Forensics
AI 理解论文
溯源树
样例
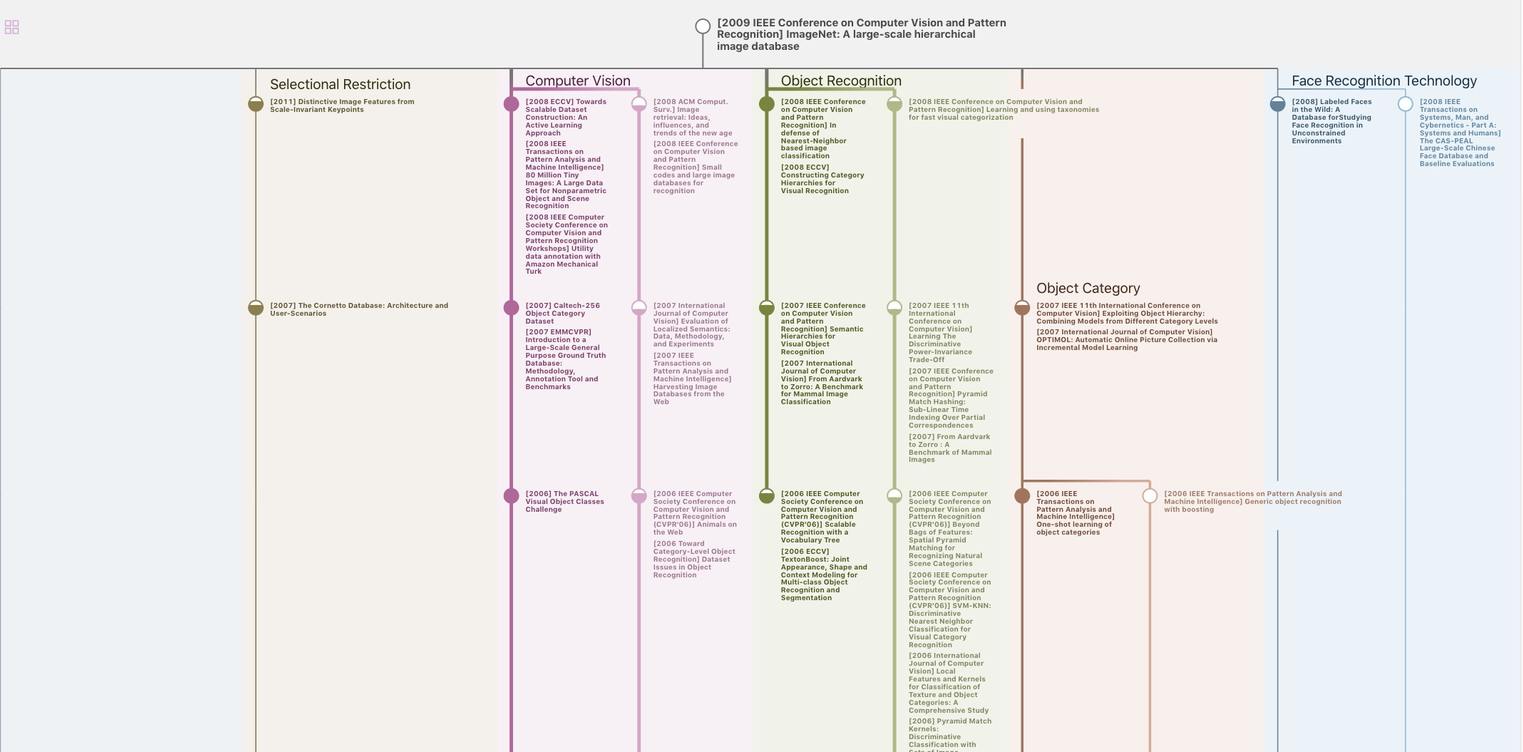
生成溯源树,研究论文发展脉络
Chat Paper
正在生成论文摘要