Bridging the Gap of Dimensions in Distillation: Understanding the knowledge transfer between different-dimensional semantic spaces
2021 INTERNATIONAL JOINT CONFERENCE ON NEURAL NETWORKS (IJCNN)(2021)
摘要
In recent years, knowledge distillation has been widely used in the field of deep learning in order to reduce the model size and save time and space. The student-teacher paradigm is a framework for knowledge distillation, and knowledge distillation proposed to minimize the KL divergence between the probabilistic outputs of a teacher and student network. However, apart from the probabilistic outputs, there are much valuable information contained in the middle layers of the teacher network. As for NLP tasks, the hidden vectors from different layers of a model have different semantic information, but the vectors' dimension of the student network is different from that of the teacher network in many cases, which makes hidden layer distillation hard to be performed directly. We propose to simply use a transition matrix to project the student's vector to a space of the same dimension as the teacher's vector, and we theoretically prove the effectiveness of this method. Our analysis shows how the transition matrix preserve important semantic information, which is closely related to the vector's characteristic in Euclidean space. We provide a geometric method for the interpretability of shared knowledge space for student-teacher architectures. Our experiments show that this method can significantly improve the performance of a small model in different tasks with different models.
更多查看译文
关键词
NLP, knowledge distillation, semantic space, transition matrix, interpretability
AI 理解论文
溯源树
样例
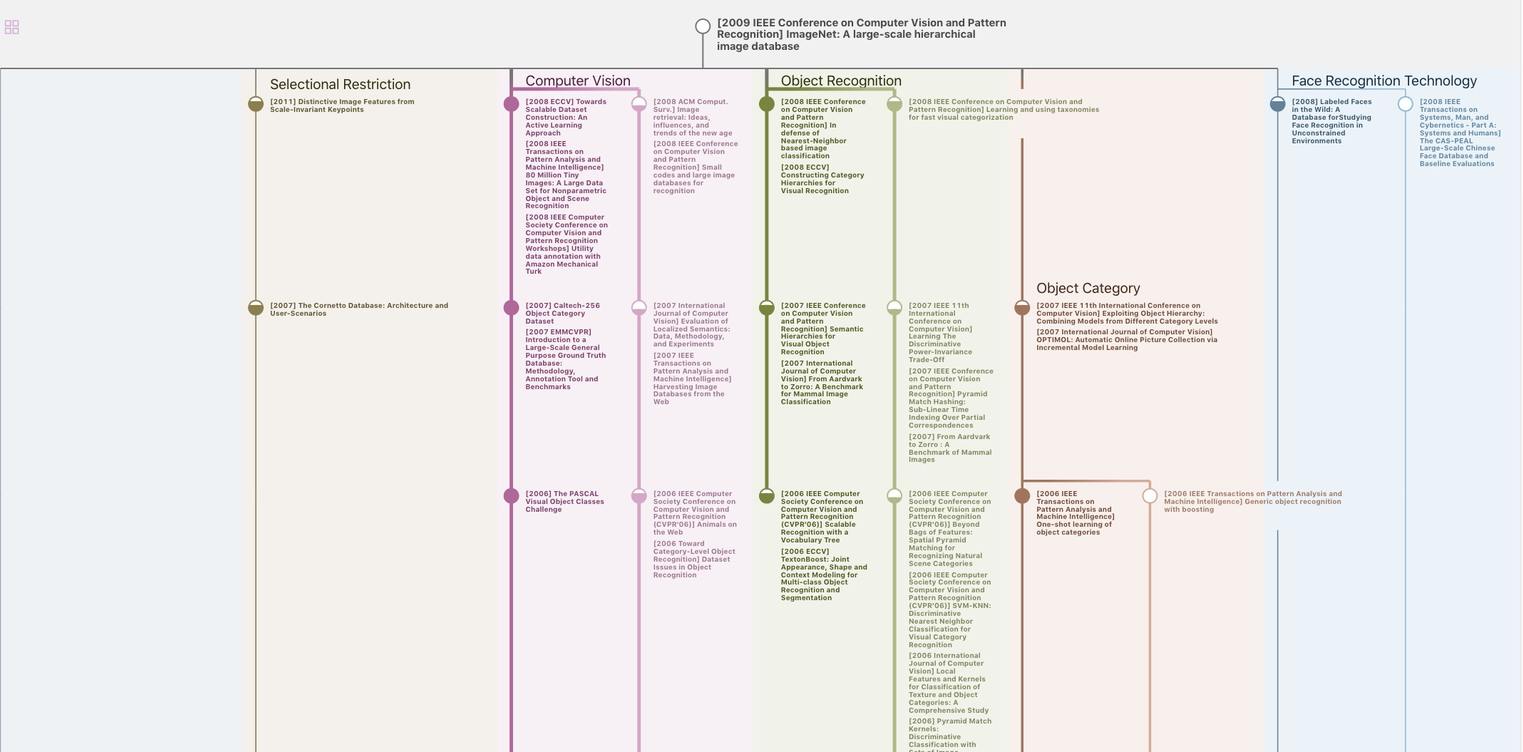
生成溯源树,研究论文发展脉络
Chat Paper
正在生成论文摘要