Attention-based Multi-proximity Preserved Attributed Network Embedding
2021 INTERNATIONAL JOINT CONFERENCE ON NEURAL NETWORKS (IJCNN)(2021)
摘要
Network embedding is an essential task in network analysis, which aims to learn low-dimensional representations for nodes in the networks. To obtain better embeddings, the structural information and inherent properties of networks should be preserved during the network embedding procedure. Recent studies only take into consideration the lower-order or microscopic structure of networks, such as the first- and second-order proximities between nodes. Most of them, however, fail to capture the mesoscopic structure and higher-order structure of networks which are also prominent features of networks, such as community structure and motifs (i.e. the frequently occurred small dense subgraph patterns of networks). In this paper, we propose a novel attention-based graph neural network called Multi-proximity Attention Network (MAN) for network embedding that simultaneously utilizes the underlying proximity dependencies between nodes from multiple aspects. Extensive experiments are conducted in two downstream tasks, namely node classification and node clustering. Experimental results demonstrate the effectiveness of the proposed method.
更多查看译文
关键词
Graph Representation Learning, Community Structure, Higher-order Structure, Graph Neural Network, Attention
AI 理解论文
溯源树
样例
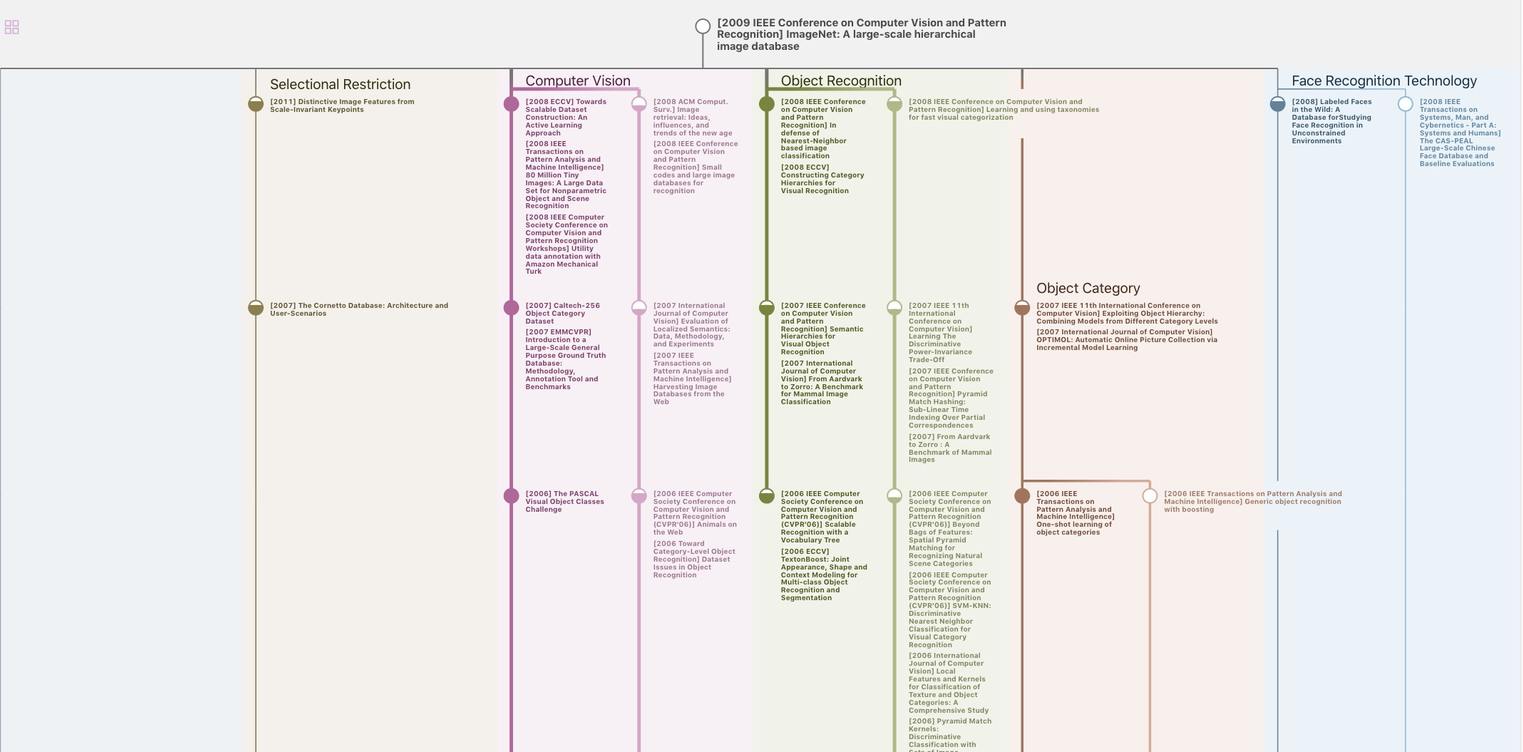
生成溯源树,研究论文发展脉络
Chat Paper
正在生成论文摘要