Learning Diverse Local Patterns for Deepfake Detection with Image-level Supervision
2021 INTERNATIONAL JOINT CONFERENCE ON NEURAL NETWORKS (IJCNN)(2021)
摘要
To prevent the Deepfake-like videos from spreading, researchers have proposed many anti-forgery methods. However, most approaches require pixel-wise annotation, which conflicts with the real Deepfake detection scenario. To make full use of the image-level label, we propose a Local-Prediction framework that indirectly allows the image-level label to supervise local regions. To further enrich the local feature, we introduced the Local-Diversity concept in the Deepfake detection field for the first time. We proposed the Local-Diversity Loss based on the motivation that regional pattern differences can provide semi-supervised information during training. Compared to the previous method, our approach limits each classification unit's receptive field and enriches the feature diversity. In the experiment, our method is evaluated on three benchmark datasets of four widely-used manipulation types. The result shows that the Local-Prediction framework is beneficial to different CNN backbones and achieved significant performance. The proposed LD loss enriches the learned patterns of binary classifiers. Furthermore, we provide visualization and ablation studies to understand the mechanism.
更多查看译文
关键词
Deepfake, face forensics, neural networks, local-diversity loss
AI 理解论文
溯源树
样例
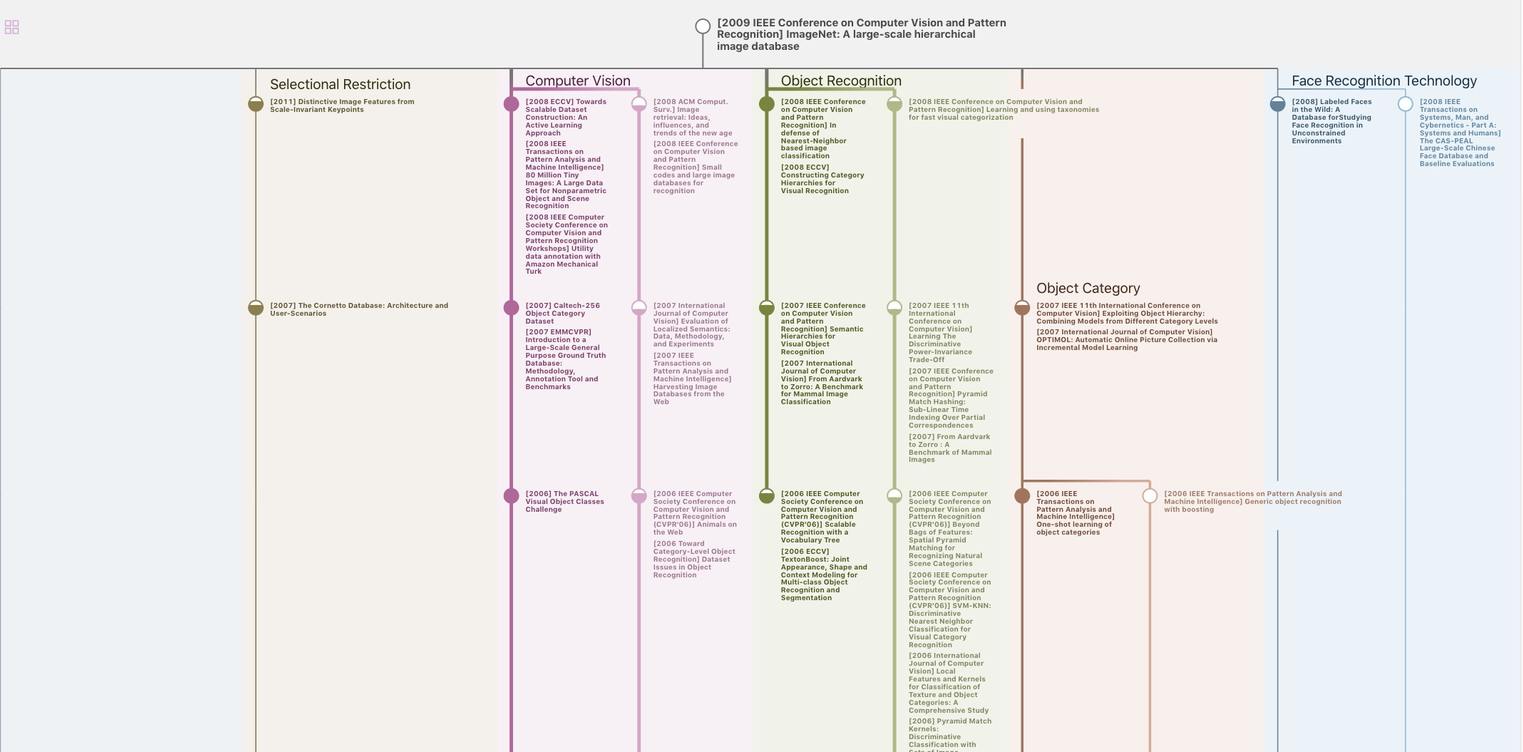
生成溯源树,研究论文发展脉络
Chat Paper
正在生成论文摘要