A Recurrent Reinforcement Learning Approach for Small Object Detection with Dynamic Refinement
2021 INTERNATIONAL JOINT CONFERENCE ON NEURAL NETWORKS (IJCNN)(2021)
摘要
Small object detection is one of the challenging tasks in recent computer vision researches. The low resolution and noisy representation are internal reasons associated with small object detection. To better address this task, we propose a novel reinforcement learning approach with dynamic refinement. Specifically, we design a Recurrent Reinforcement Module (RRM) to iteratively learn contextual information and improve semantic label dependency and the image-label relevance. Besides, the Dynamic Refinement Module (DRM) is designed to dynamically adjust the attentive regions that are related to small objects. To evaluate the effectiveness of our proposed approach, we design extensive experiments and comparisons on four datasets (i.e. PASCAL VOC, MS COCO, Google AVA and UA-DETRAC). The comprehensive experiment results demonstrate that our approach has the superiority of small object detection for both images and videos.
更多查看译文
关键词
small object detection, recurrent reinforcement learning, dynamic refinement
AI 理解论文
溯源树
样例
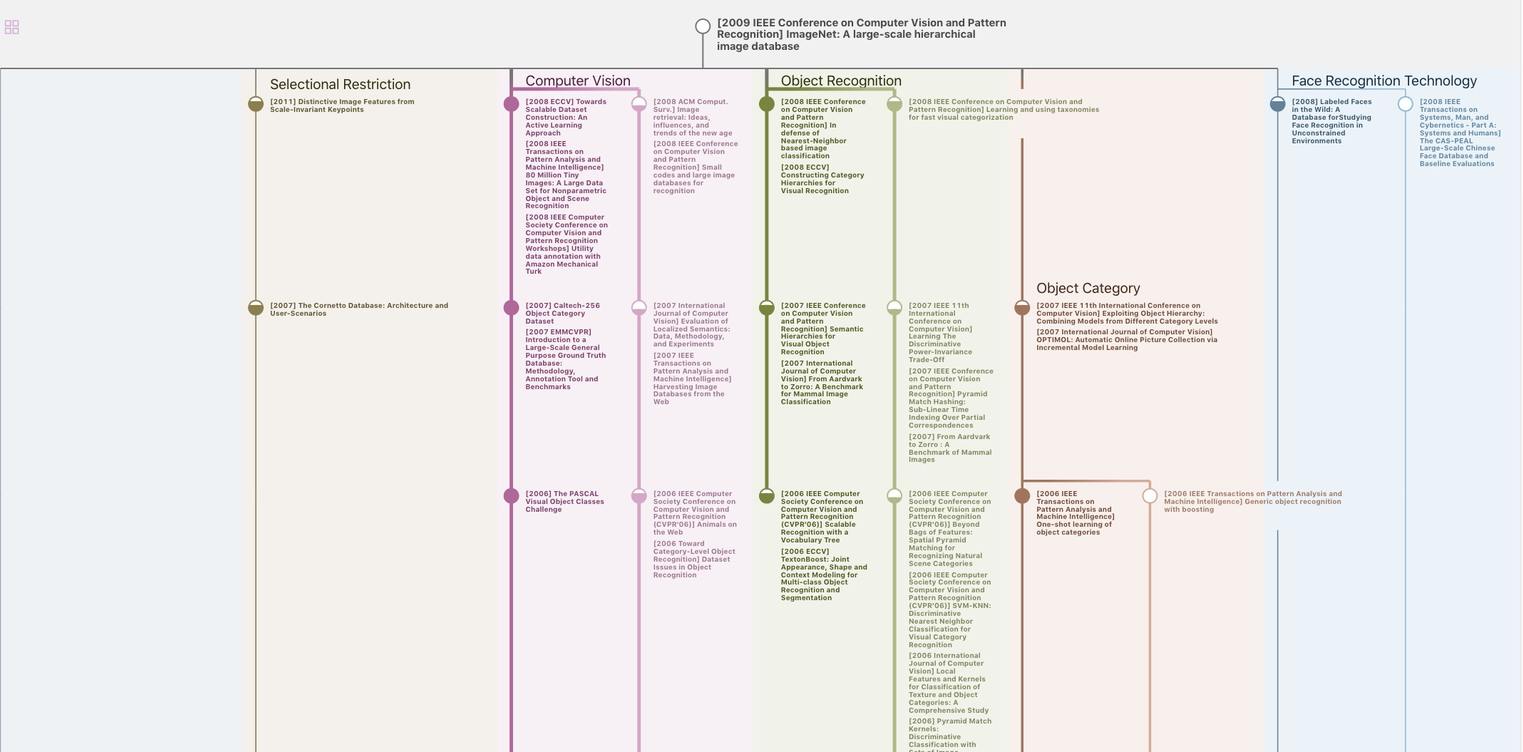
生成溯源树,研究论文发展脉络
Chat Paper
正在生成论文摘要