Wind Speed Forecasting via Multi-task Learning
2021 INTERNATIONAL JOINT CONFERENCE ON NEURAL NETWORKS (IJCNN)(2021)
摘要
The viability of wind power massive use goes through an effective estimation of the power to be produced in wind farms, one of the fastest growing sources of renewable energy. Therefore, short-term wind speed forecasting involving several turbines and possibly multiple wind farms becomes a crucial practical demand. Once there are historical time series of wind speed intensity associated with subgroups of turbines, multiple tasks of time series prediction should be solved simultaneously. Aiming at performance improvement, we propose here the adoption of information sharing in multi-task learning frameworks, involving linear and nonlinear prediction models, and time series with and without differentiation. The nonlinear multi-task learning approach is based on extreme learning machines (ELMs) and guided to the best accuracies on a real-world case study of short-term wind speed forecasting. A suitable tuning of the variance in the distribution of the random hidden layer weights was demonstrated to be an essential preprocessing step for the ELMs, together with regularization routines. Additionally, it was found that properly incorporating tasks relationship into the learning process can systematically contribute to better generalization, particularly when compared to the single task learning counterparts.
更多查看译文
关键词
Multi-task learning, extreme learning machine, wind speed forecasting, renewable energy
AI 理解论文
溯源树
样例
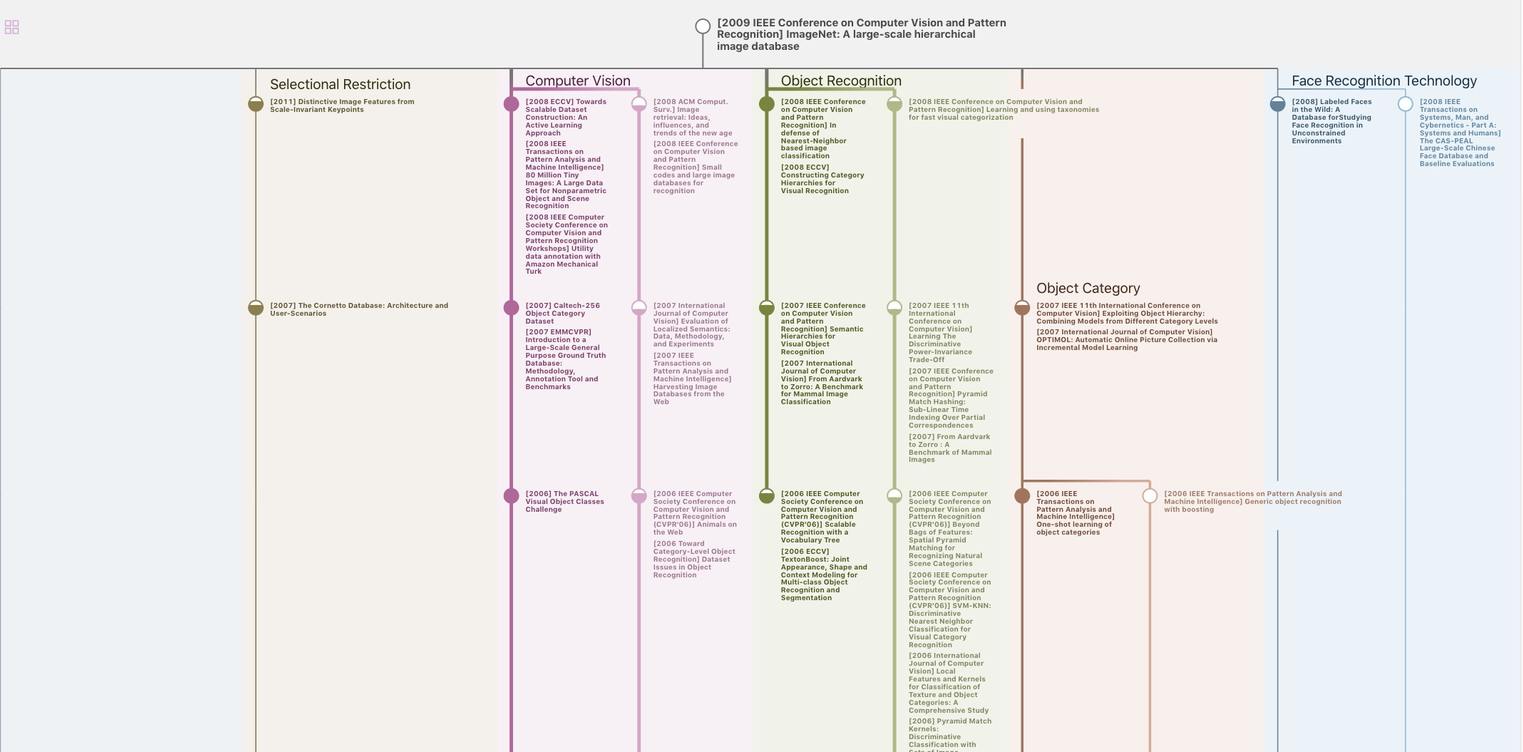
生成溯源树,研究论文发展脉络
Chat Paper
正在生成论文摘要