A Novel Feature Importance Based Layer to Improve Neural Networks
2021 INTERNATIONAL JOINT CONFERENCE ON NEURAL NETWORKS (IJCNN)(2021)
摘要
The normalized cross-correlation coefficient is a standard metric that can be used to evaluate the relation of one vector with another. In machine learning and as part of feature selection, the coefficient can be used to evaluate the importance of each feature in relation to the required output. This work aims to utilize this coefficient or similar feature importance indicators to improve the accuracy of a neural network by first computing the corresponding coefficient of each of the input features with the required output and then by creating a specialized network layer that can be used to improve the accuracy of the network. The specialized and novel layer requires adding a number of parameters that depends on the number of input features to the network. These parameters are optimized through the learning algorithm utilized by the network. The proposed layer guides the network to perform better with little effect on the computational cost by initially weighing each feature according to its importance such as the correlation. It is possible to use meaningful coefficients such as the Pearson correlation, the Spearman coefficient or others. The results indicate a consistently enhanced performance with the accuracy gain often exceeding 1% to more than 5% in some cases. The work considers several types of data including regression datasets, multi-output and convolutional neural networks related problems with concentration on binary classification datasets.
更多查看译文
关键词
Pearson correlation,accuracy gain,multioutput,convolutional neural networks,novel feature importance,neural network,normalized cross-correlation coefficient,machine learning,feature selection,required output,similar feature importance indicators,corresponding coefficient,specialized network layer,learning algorithm,computational cost,meaningful coefficients
AI 理解论文
溯源树
样例
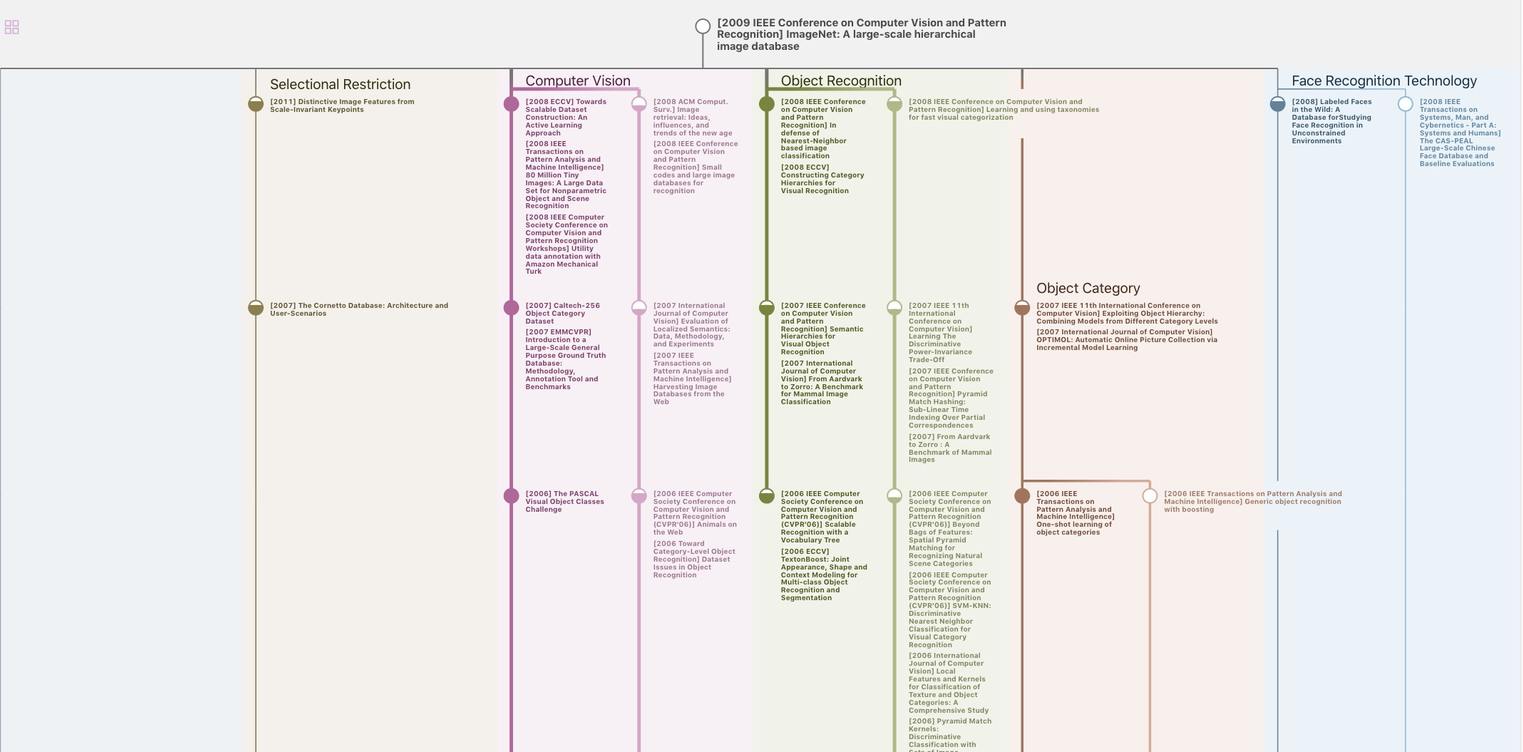
生成溯源树,研究论文发展脉络
Chat Paper
正在生成论文摘要