An Energy-Efficient SNN Processor Design based on Sparse Direct Feedback and Spike Prediction
2021 INTERNATIONAL JOINT CONFERENCE ON NEURAL NETWORKS (IJCNN)(2021)
摘要
In this paper, we present a novel spike prediction technique based spiking neural network (SNN) architecture, which can provide low cost on-chip learning based on the sparse direct feedback alignment (DFA). First, in order to reduce the repetitive synaptic operations in feedforward operations, a spike prediction technique is proposed, where the output spikes of active and inactive neurons are predicted by tracing the membrane potential changes. The proposed spike prediction achieves 63.84% reduction of the synaptic operations, and it can be efficiently exploited in the training as well as inference process. In addition, the number of weight updates in backward operations has been reduced by applying sparse DFA. In the sparse DFA, the synaptic weight updates are computed using the sparse feedback connections and the output error that is sparse as well. As a result, the number of weight updates in the training process has been reduced to 65.17%. The SNN processor with the proposed spike prediction technique and the sparse DFA has been implemented using 65nm CMOS process. The implementation results show that the SNN processor achieves the training energy savings of 52.16% with 0.3% accuracy degradation in MNIST dataset. It also consumes 1.18 uJ/image and 1.34 uJ/image for inference and training, respectively, with 97.46% accuracy on MNIST dataset.
更多查看译文
关键词
Spiking neural network, spike prediction, sparse direct feedback alignment, energy-efficient neuromorphic system, on-chip learning
AI 理解论文
溯源树
样例
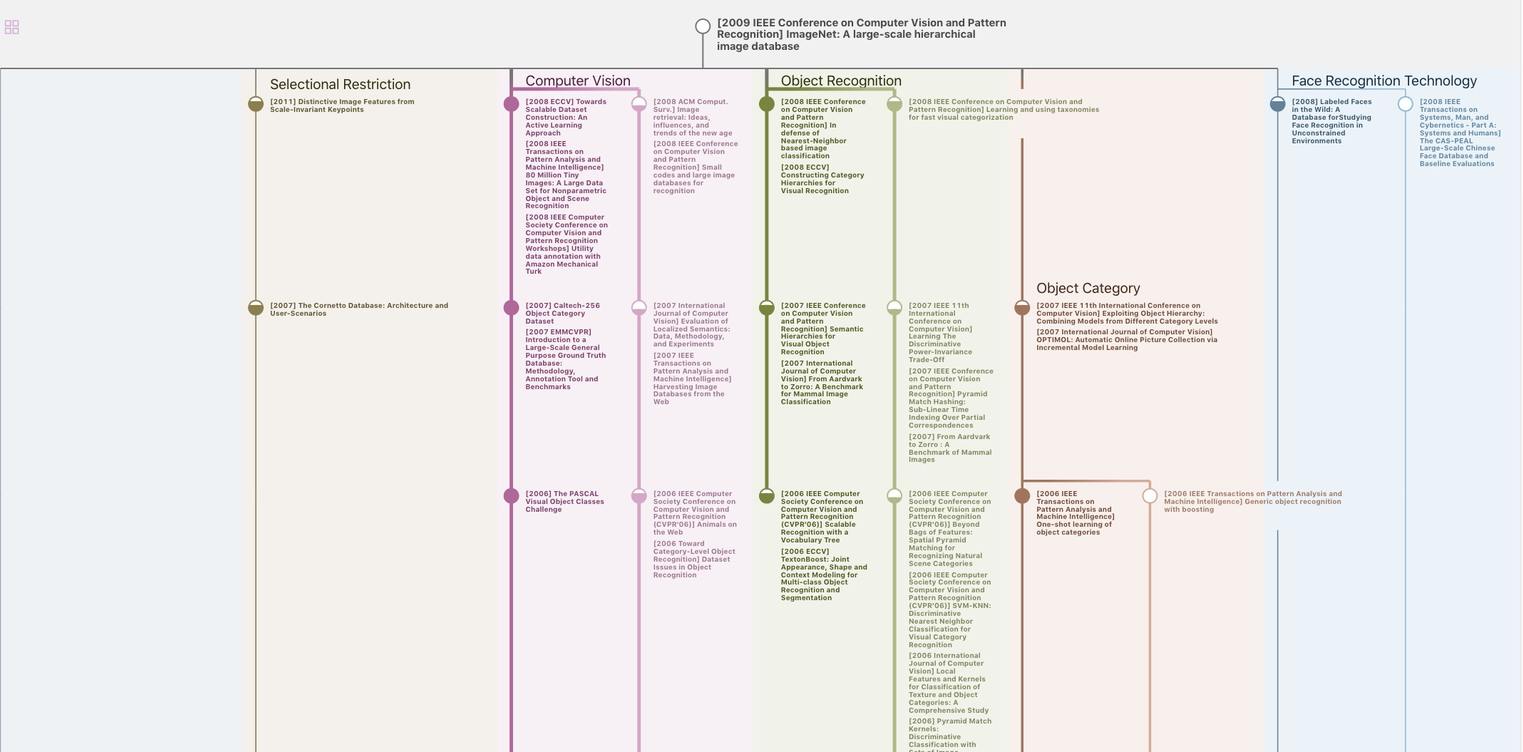
生成溯源树,研究论文发展脉络
Chat Paper
正在生成论文摘要