SPAD plus : An Improved Probabilistic Anomaly Detector based on One-dimensional Histograms
2021 INTERNATIONAL JOINT CONFERENCE ON NEURAL NETWORKS (IJCNN)(2021)
摘要
In today's world, databases are growing rapidly. Fast automatic detection of anomalous records in these massive databases is a challenging task. Traditional distance-based anomaly detectors are limited to small datasets because of their high time complexities. The univariate histogram-based method is arguably the fastest anomaly detection method. The anomaly score of a data instance is computed as the product of the probability mass of histograms in each dimension. Recent studies proved that such a simple method is comparable with many state-of-the-art methods on several datasets. However, as data features are assumed to be independent, it results in poor performance when features are correlated. Such an issue can be taken care of by using Principal Component (PC) features, which is the primary element of this paper. Our results show that integrating PCs with the original input features improves the performance of histogram-based anomaly detector with no real compromise in computational complexity.
更多查看译文
关键词
Big data, Fast anomaly detection, Histogram-based anomaly detection, SPAD, iForest, LOF
AI 理解论文
溯源树
样例
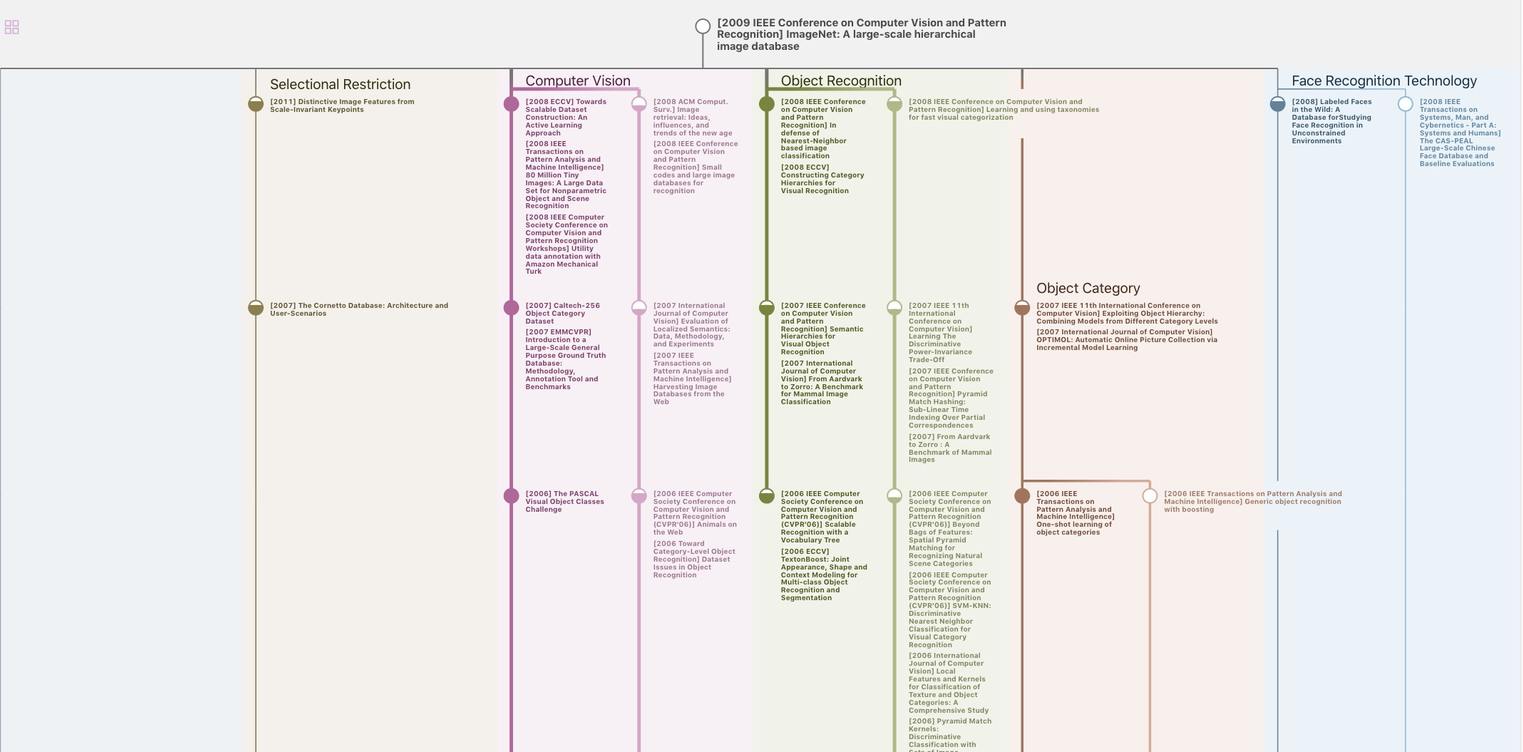
生成溯源树,研究论文发展脉络
Chat Paper
正在生成论文摘要