An improved ensemble learning method for exchange rate forecasting based on complementary effect of shallow and deep features
Expert Systems with Applications(2021)
摘要
In prior studies, either the shallow feature or deep feature has been extracted for accurate exchange rate forecasting. However, the complementary effect and distinguished predictive power of multiple features have rarely been investigated, which limits the utilization of comprehensive predictive information. Therefore, a novel ensemble learning method, Adaptive Linear Sparse Random Subspace (ALS-RS), is proposed based on the complementary effect of shallow and deep features. Concretely, in the first stage, the shallow feature is constructed manually combined with expert knowledge and the deep feature is extracted automatically by Bidirectional Gated Recurrent Units (Bi-GRU), then the features obtained are used as model inputs. After that, the improved RS with a feature weighting mechanism is designed to discriminate the importance of each feature and make an accurate ensemble prediction. The experimental results on four exchange rate datasets validate the superiority of our proposed ALS-RS. Besides, the enhanced forecasting capability of fusing multiple features including shallow and deep features is confirmed.
更多查看译文
关键词
Exchange rate forecasting,Deep learning,Multiple features,Ensemble learning,Feature weighting
AI 理解论文
溯源树
样例
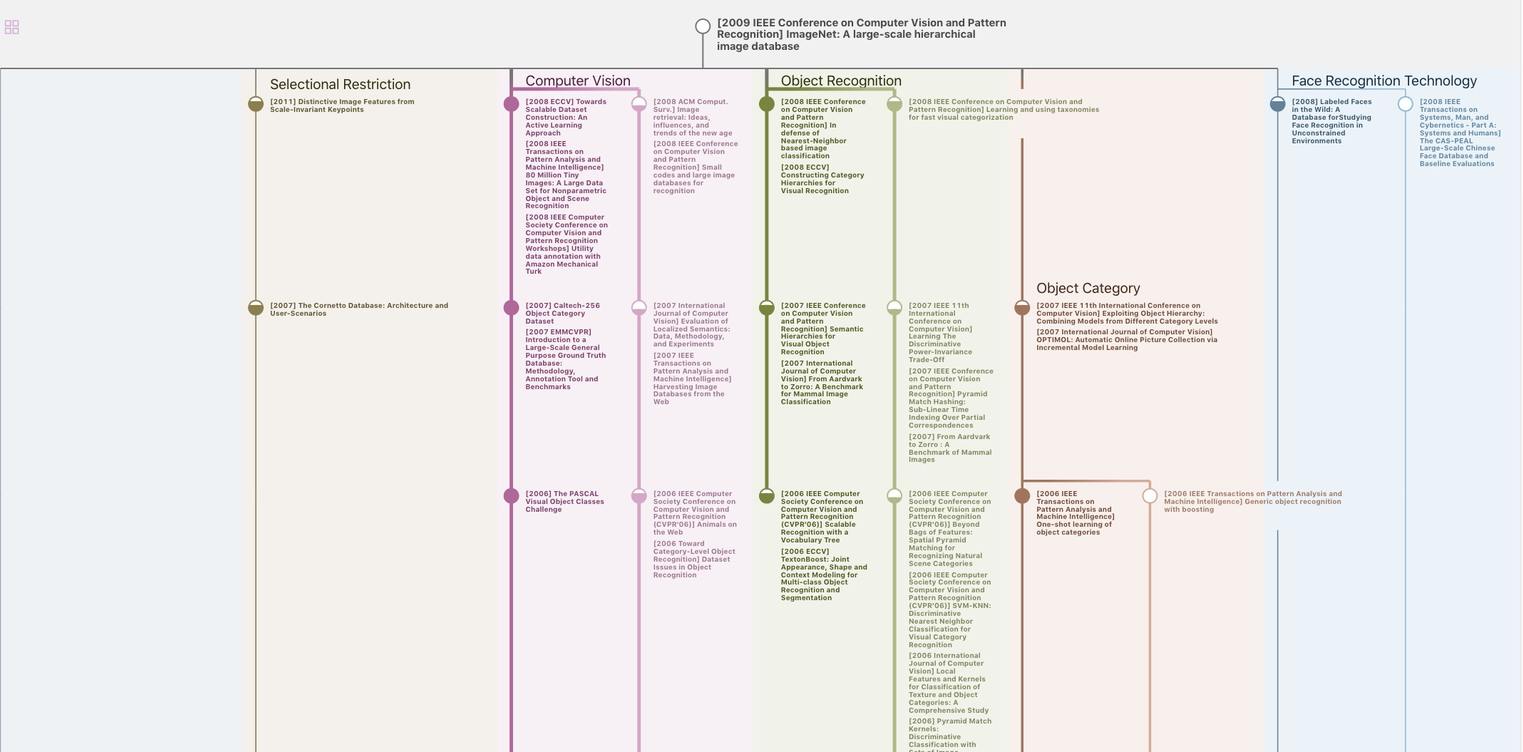
生成溯源树,研究论文发展脉络
Chat Paper
正在生成论文摘要