BERT got a Date: Introducing Transformers to Temporal Tagging
arxiv(2021)
摘要
Temporal expressions in text play a significant role in language understanding and correctly identifying them is fundamental to various retrieval and natural language processing systems. Previous works have slowly shifted from rule-based to neural architectures, capable of tagging expressions with higher accuracy. However, neural models can not yet distinguish between different expression types at the same level as their rule-based counterparts. n this work, we aim to identify the most suitable transformer architecture for joint temporal tagging and type classification, as well as, investigating the effect of semi-supervised training on the performance of these systems. After studying variants of token classification and encoder-decoder architectures, we ultimately present a transformer encoder-decoder model using RoBERTa language model as our best performing system. By supplementing training resources with weakly labeled data from rule-based systems, our model surpasses previous works in temporal tagging and type classification, especially on rare classes. Additionally, we make the code and pre-trained experiment publicly available
更多查看译文
关键词
temporal tagging,bert,transformers,date
AI 理解论文
溯源树
样例
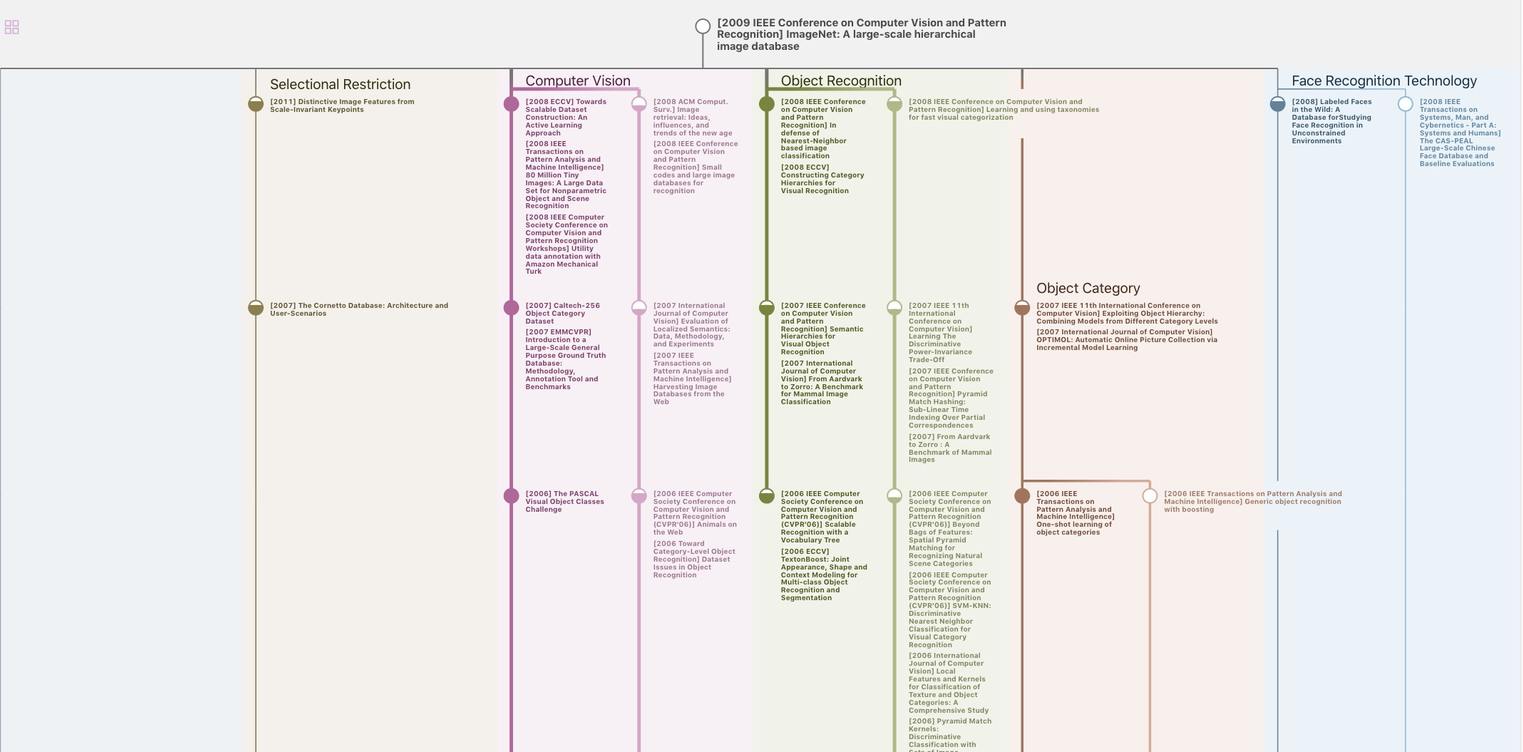
生成溯源树,研究论文发展脉络
Chat Paper
正在生成论文摘要