Mitigation of Adversarial Policy Imitation via Constrained Randomization of Policy (CRoP)
arxiv(2021)
摘要
Deep reinforcement learning (DRL) policies are vulnerable to unauthorized replication attacks, where an adversary exploits imitation learning to reproduce target policies from observed behavior. In this paper, we propose Constrained Randomization of Policy (CRoP) as a mitigation technique against such attacks. CRoP induces the execution of sub-optimal actions at random under performance loss constraints. We present a parametric analysis of CRoP, address the optimality of CRoP, and establish theoretical bounds on the adversarial budget and the expectation of loss. Furthermore, we report the experimental evaluation of CRoP in Atari environments under adversarial imitation, which demonstrate the efficacy and feasibility of our proposed method against policy replication attacks.
更多查看译文
关键词
adversarial policy imitation,constrained randomization,crop
AI 理解论文
溯源树
样例
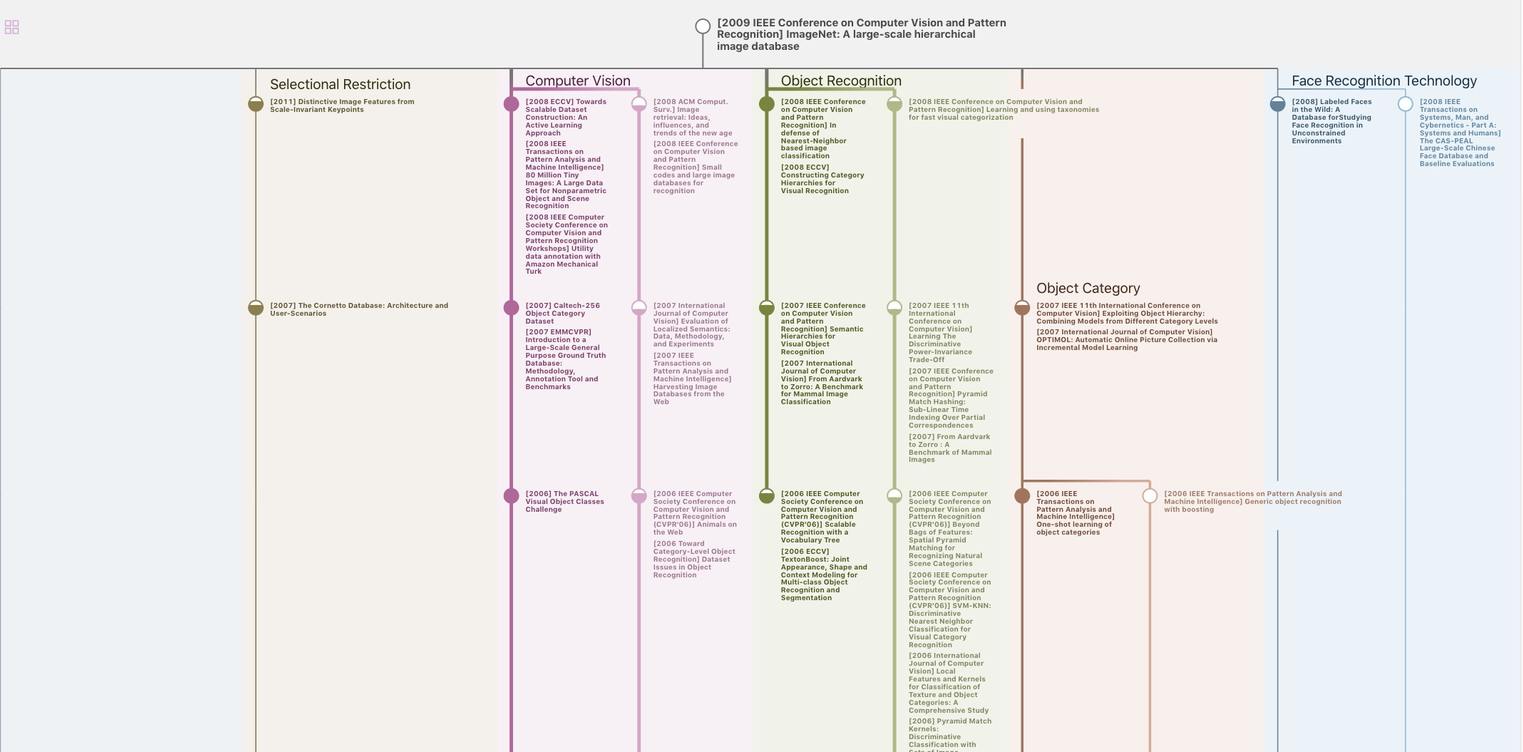
生成溯源树,研究论文发展脉络
Chat Paper
正在生成论文摘要