Energy-Based Supervised Hashing for Multimorbidity Image Retrieval
MEDICAL IMAGE COMPUTING AND COMPUTER ASSISTED INTERVENTION - MICCAI 2021, PT V(2021)
摘要
Content-based image retrieval (CBIR) has attracted increasing attention in the field of computer-aided diagnosis, for which learning-based hashing approaches represent the most prominent techniques for large-scale image retrieval. In this work, we propose a Supervised Hashing method with Energy-Based Modeling (SH-EBM) for scalable multi-label image retrieval, where concurrence of multiple symptoms with subtle differences in visual feature makes the search problem quite challenging, even for sophisticated hashing models built upon modern deep architectures. In addition to similarity-preserving ranking loss, multi-label classification loss is often employed in existing supervised hashing to further improve the expressiveness of hash codes, by optimizing a normalized probabilistic objective with tractable likelihood (e.g., multi-label crossentropy). On the contrary, we present a multi-label EBM loss without restriction on the tractability of the log-likelihood, which is more flexible to parameterize and can model a more expressive probability distribution over multimorbidity image data. We further develop a multilabel Noise Contrastive Estimation (ml-NCE) algorithm for discriminative training of the proposed hashing network. On a multimorbidity dataset constructed by the NIH Chest X-ray, our SH-EBM outperforms most supervised hashing methods by a significant margin, implying its effectiveness in facilitating multilevel similarity preservation for scalable image retrieval.
更多查看译文
关键词
Multimorbidity medical image retrieval, Supervised hashing, Energy-based model, Noise contrastive estimation
AI 理解论文
溯源树
样例
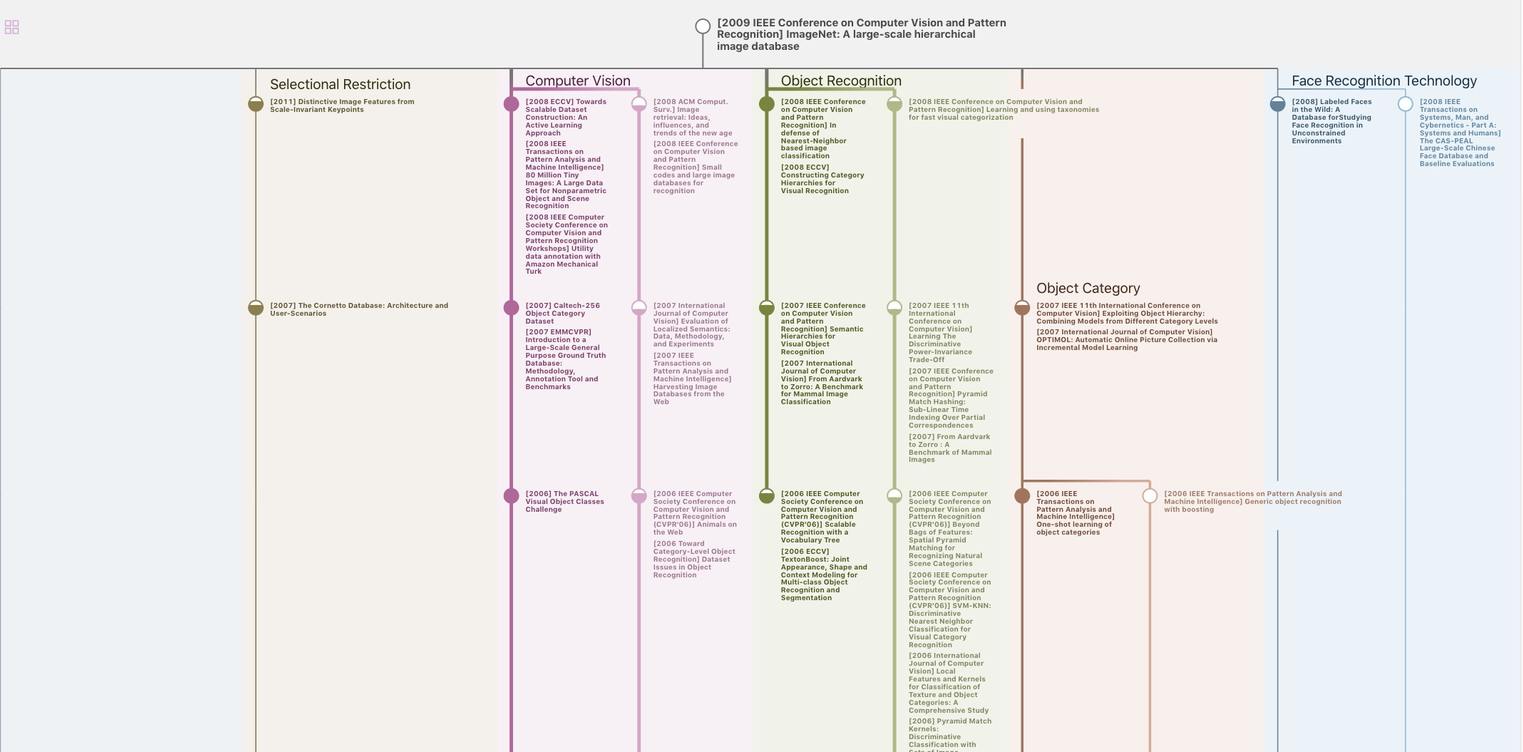
生成溯源树,研究论文发展脉络
Chat Paper
正在生成论文摘要