M-SEAM-NAM: Multi-instance Self-supervised Equivalent Attention Mechanism with Neighborhood Affinity Module for Double Weakly Supervised Segmentation of COVID-19
MEDICAL IMAGE COMPUTING AND COMPUTER ASSISTED INTERVENTION - MICCAI 2021, PT VII(2021)
摘要
The Coronavirus Disease 2019 (COVID-19) pandemic has swept the whole world since 2019. Chest computed tomography (CT) plays an important role in clinical diagnosis, management and progression monitoring of COVID-19 patients. In order to decrease the cost of manual segmentation, weakly supervised segmentation methods, such as class activation maps (CAM) based methods, have been applied to achieve COVID-19-related lesion segmentation. Such methods could be used to localize the lesion preliminarily, but it is not precise enough to segment the lesion. In this paper, we propose a double weakly supervised segmentation method to achieve the segmentation of COVID-19 lesions on CT scans. A self-supervised equivalent attention mechanism with neighborhood affinity module is proposed for accurate segmentation. Multi-instance learning is adopted for training using annotations weaker than image-level. A simple pre-training process is also proved to be effective. We achieve a higher average Dice compared to Unet (0.782 vs 0.601) on COVID-19 lesion segmentation tasks. Codes in this paper will be available at https://github.com/TangWen920812/M-SEAM-NAM.
更多查看译文
关键词
Weakly supervised segmentation,Multi-instance learning,COVID-19
AI 理解论文
溯源树
样例
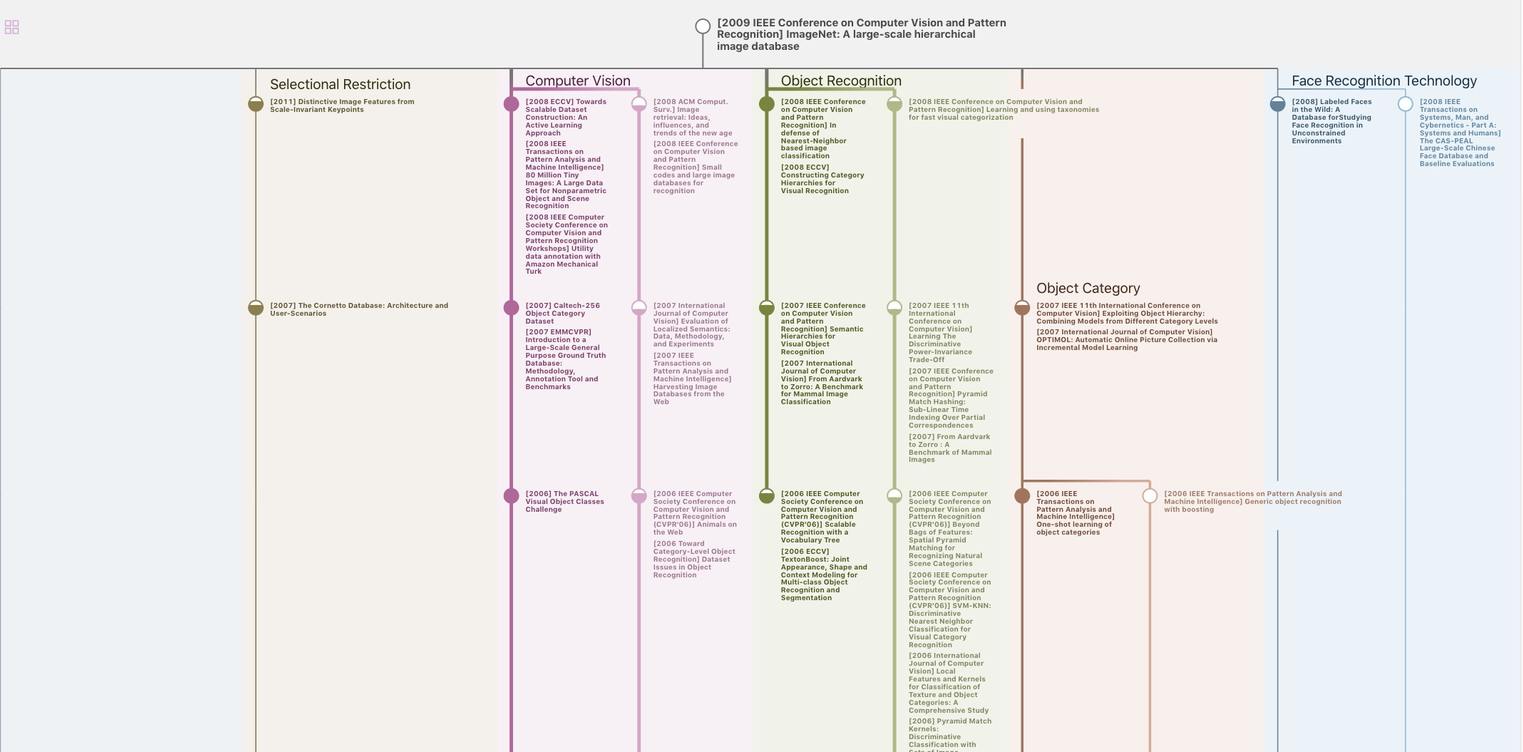
生成溯源树,研究论文发展脉络
Chat Paper
正在生成论文摘要