Label-Free Physics-Informed Image Sequence Reconstruction with Disentangled Spatial-Temporal Modeling
MEDICAL IMAGE COMPUTING AND COMPUTER ASSISTED INTERVENTION - MICCAI 2021, PT VI(2021)
摘要
Traditional approaches to image reconstruction uses physicsbased loss with data-efficient inference, although the difficulty to properly model the inverse solution precludes learning the reconstruction across a distribution of data. Modern deep learning approaches enable expressive modeling but rely on a large number of reconstructed images (labeled data) that are often not available in practice. To combine the best of the above two lines of works, we present a novel label-free image reconstruction network that is supervised by physics-based forward operators rather than labeled data. We further present an expressive yet disentangled spatial-temporal modeling of the inverse solution, where its latent dynamics is modeled by neural ordinary differential equations and its emission over non-Euclidean geometrical domains by graph convolutional neural networks. We applied the presented method to reconstruct electrical activity on the heart surface from body-surface potential. In simulation and real-data experiments in comparison to both traditional physicsbased and modern data-driven reconstruction methods, we demonstrated the ability of the presented method to learn how to reconstruct using observational data without any corresponding labels.
更多查看译文
关键词
Image reconstruction, Neural ODE, Graph convolution
AI 理解论文
溯源树
样例
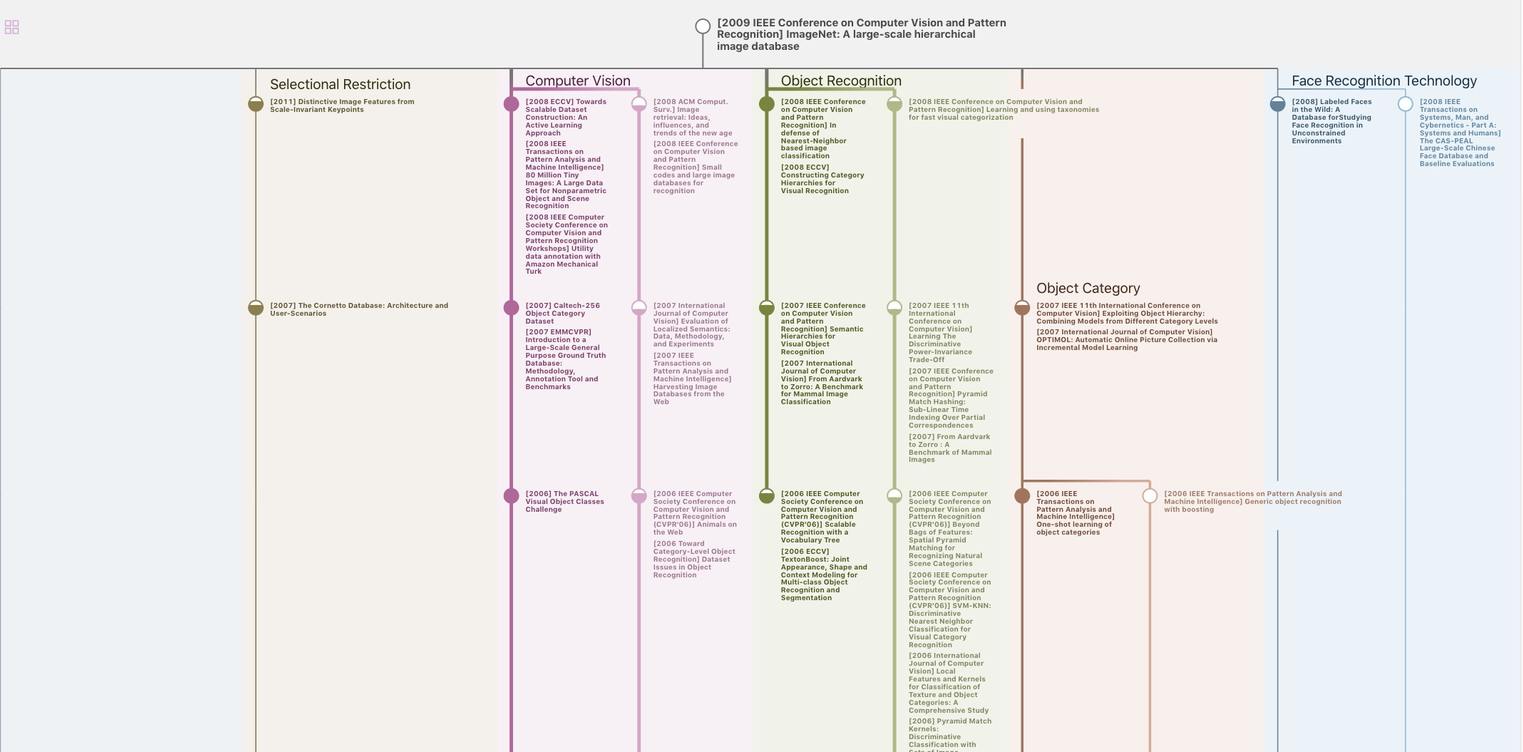
生成溯源树,研究论文发展脉络
Chat Paper
正在生成论文摘要