Hierarchical Attention Guided Framework for Multi-resolution Collaborative Whole Slide Image Segmentation
MEDICAL IMAGE COMPUTING AND COMPUTER ASSISTED INTERVENTION - MICCAI 2021, PT VIII(2021)
摘要
Segmentation of whole slide images (WSIs) is an important step for computer-aided cancer diagnosis. However, due to the gigapixel dimension, WSIs are usually cropped into patches for analysis. Processing high-resolution patches independently may leave out the global geographical relationships and suffer slow inference speed while using low-resolution patches can enlarge receptive fields but lose local details. Here, we propose a Hierarchical Attention Guided (HAG) framework to address above problems. Particularly, our framework contains a global branch and several local branches to perform prediction at different scales. Additive hierarchical attention maps are generated by the global branch with sparse constraints to fuse multi-resolution predictions for better segmentation. During the inference, the sparse attention maps are used as the certainty guidance to select important local areas with a quadtree strategy for acceleration. Experimental results on two WSI datasets highlight two merits of our framework: 1) effectively aggregate multi-resolution information to achieve better results, 2) significantly reduce the computational cost to accelerate the prediction without decreasing accuracy.
更多查看译文
关键词
Segmentation, Whole slide image, Deep Learning, Acceleration
AI 理解论文
溯源树
样例
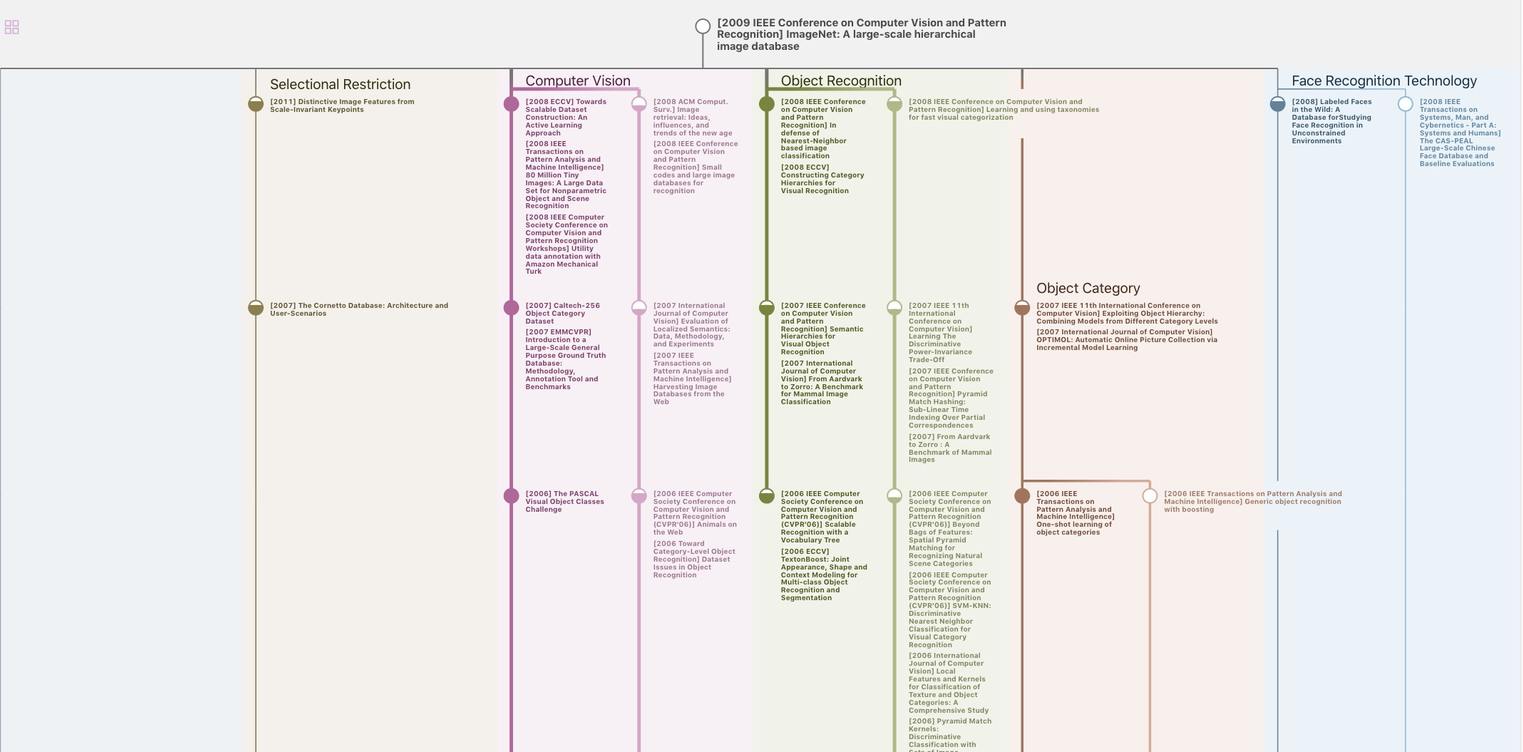
生成溯源树,研究论文发展脉络
Chat Paper
正在生成论文摘要