Hierarchical Graph Pathomic Network for Progression Free Survival Prediction
MEDICAL IMAGE COMPUTING AND COMPUTER ASSISTED INTERVENTION - MICCAI 2021, PT VIII(2021)
摘要
High resolution histology images contain information related to disease prognosis. However, survival prediction based on current clinical grading systems, which rely heavily on a pathologist's histological assessment, has significant limitations due to the heterogeneity and complexity of tissue phenotypes. To address these challenges, we propose a deep learning framework that leverages hierarchical graph-based representations to enable more precise prediction of progression-free survival in prostate cancer patients. Unlike conventional approaches that analyze patch-based or cell-based pathomic features alone without considering their spatial connectivity, we explore multi-scale topological structures of whole slide images in an integrative context. Extensive experiments have demonstrated the effectiveness of our model for better progression prediction.
更多查看译文
关键词
Progression free survival, Graph convolutional neural network, Self-supervised learning, Hierarchical graph representations
AI 理解论文
溯源树
样例
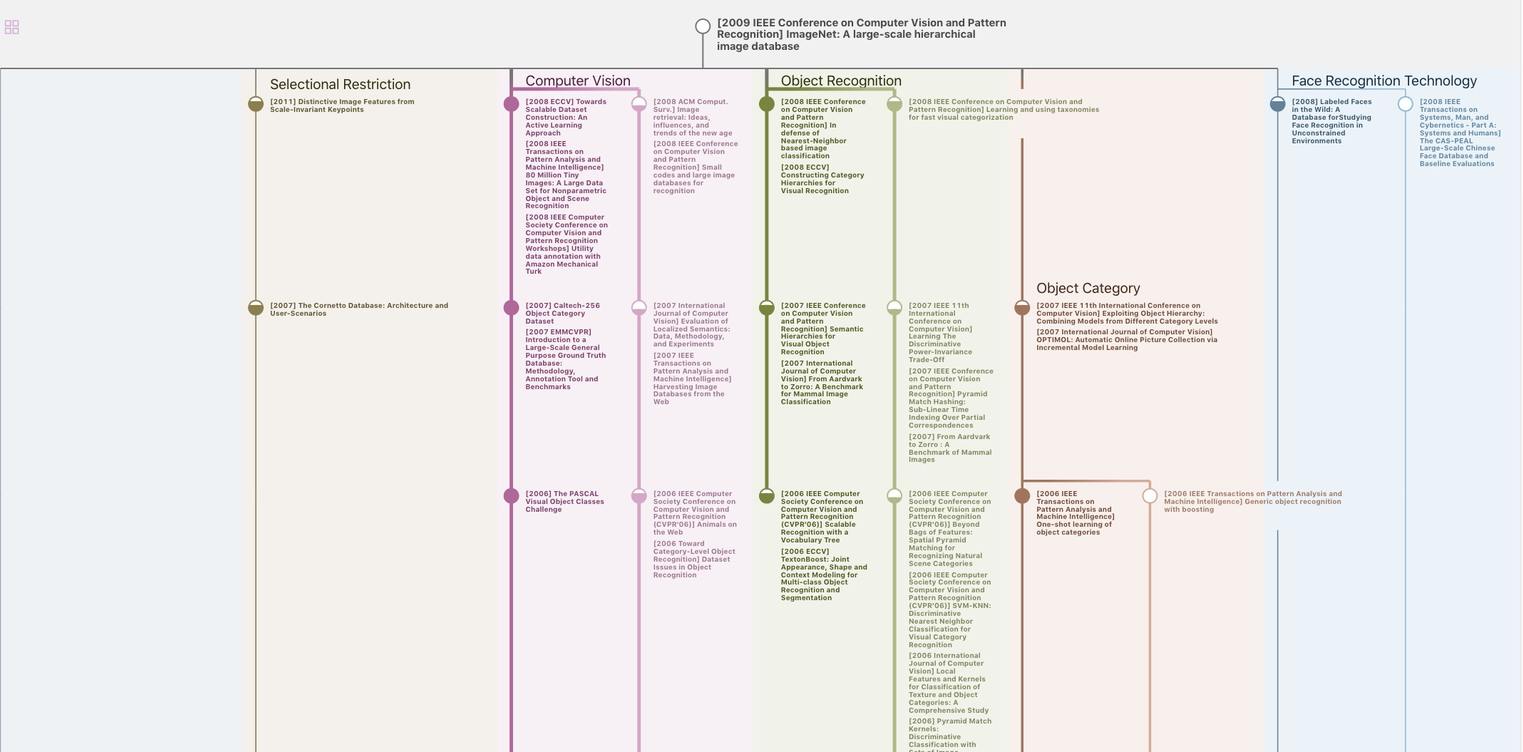
生成溯源树,研究论文发展脉络
Chat Paper
正在生成论文摘要