Human resource recommendation algorithm based on improved frequent itemset mining
Future Generation Computer Systems(2022)
摘要
There are large personalized differences among human resources, and the resource recommendation process easily encounters the problem of data loss, resulting in a short length of the final recommended resource and less information about the actual human resources included. To solve this problem, this research proposes a human resource recommendation algorithm based on improved frequent itemset mining. The dataset containing single items is called, the human resource is mined to improve frequent itemset, the positive matrix factorization (PMF) model is used to process the implicit features in human resource, and the similarity parameters of the human resource dataset are calculated. The algorithm of collaborative filtering is used to divide the recommendation mechanism, and the loss of data generated during the recommendation is controlled, thereby completing the construction of the human resource recommendation algorithm. The hardware and software environment for algorithm operation were constructed. The human resource dataset was arranged. Tests were conducted using the recommendation algorithm based on neural network, recommendation algorithm based on item weight ranking and the proposed recommendation algorithm. The test results showed that the proposed recommendation algorithm recommended the longest length of resources and the most information contained within the resources.
更多查看译文
关键词
Improved frequent itemset,Human resource,Recommendation algorithm,Data loss,PMF model,Implicit feature
AI 理解论文
溯源树
样例
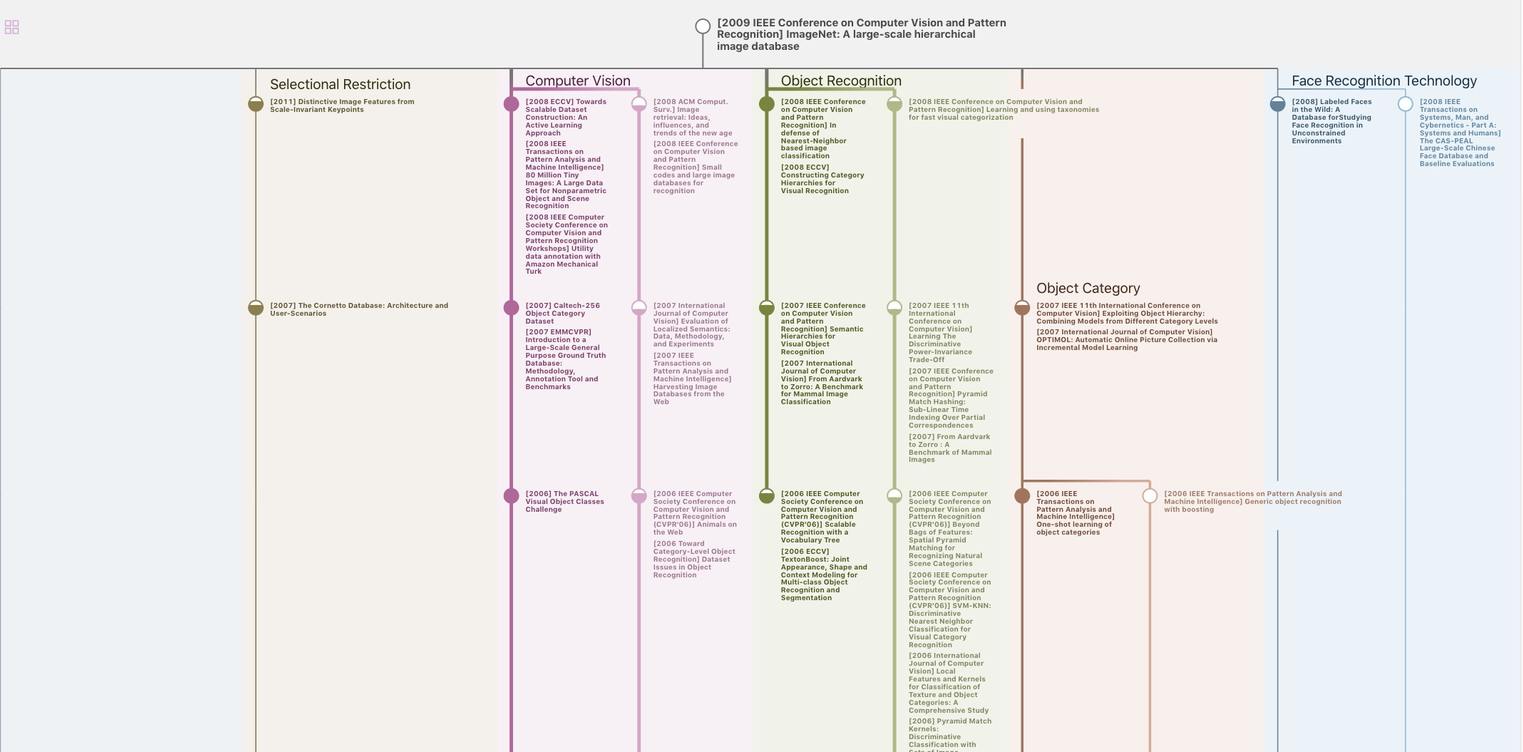
生成溯源树,研究论文发展脉络
Chat Paper
正在生成论文摘要