A binary moth search algorithm based on self-learning for multidimensional knapsack problems
Future Generation Computer Systems(2022)
摘要
A key issue for moth search algorithm (MS) is the ability to maintain sufficient diversity in the population so as to be able to track a dynamically changing landscape. However, for the original MS algorithm, the implicit learning mechanism of flight straightly operator focuses mainly on the global best individual, which inevitably causes a deteriorated evolution. To increase the population diversity and enhance the global search ability of MS, a binary moth search algorithm based on self-learning (SLMS) is proposed to solve an NP-hard combinatorial optimization problem with many diverse applications, the 0–1 multidimensional knapsack problem (MKP). In SLMS, a self-learning flight straightly operator is proposed to make each individual learn from any one better than itself, not just the global best individual. The performance of the proposed SLMS is validated by testing 89 different widely used benchmark instances. Experimental results show the effectiveness of the proposed SLMS in solving the MKP problem and the self-learning flight straightly operator can enhance population diversity and global search ability. The effect of transfer functions on the performance of SLMS is also investigated.
更多查看译文
关键词
Combinatorial optimization,Multidimensional knapsack problem,Moth search algorithm,Self-learning,Quick repair operator
AI 理解论文
溯源树
样例
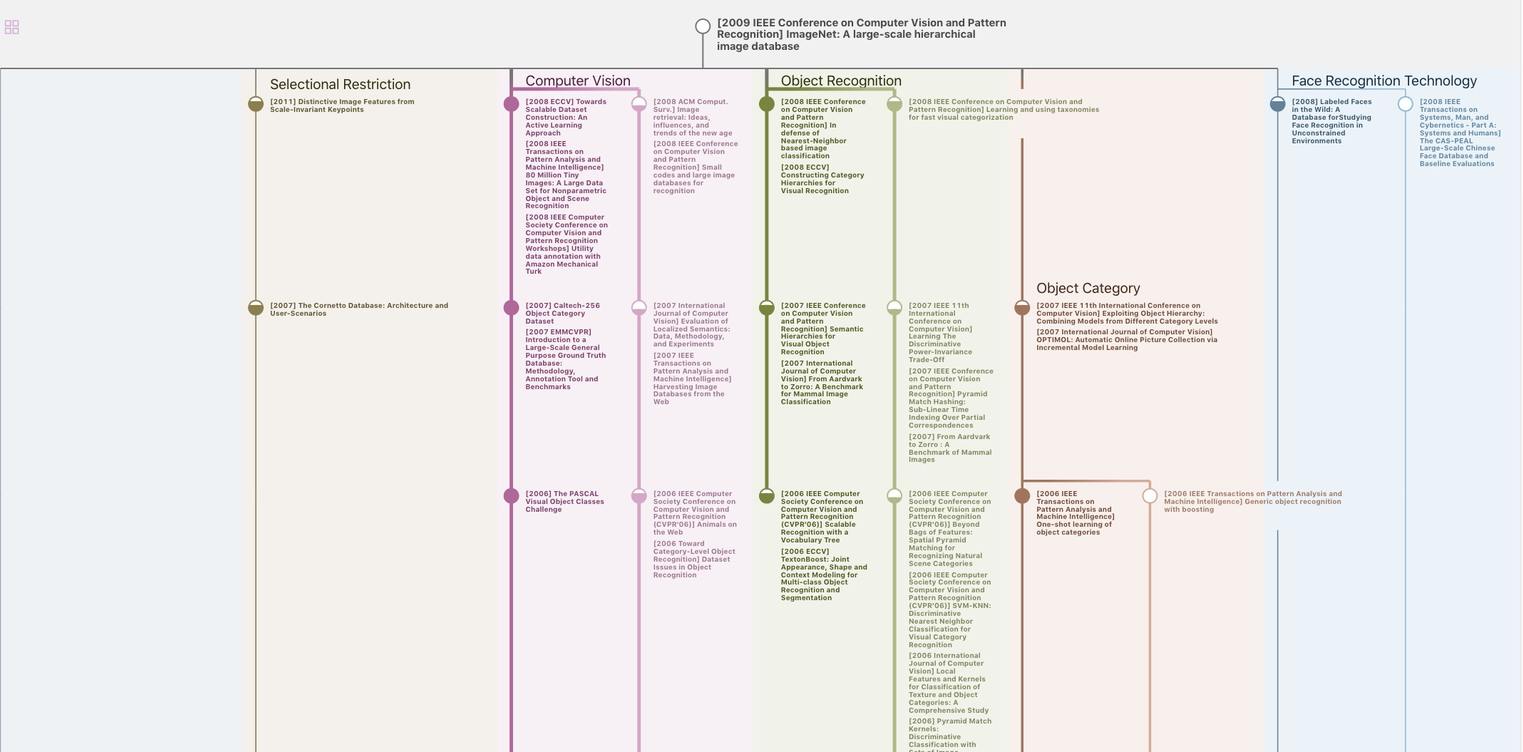
生成溯源树,研究论文发展脉络
Chat Paper
正在生成论文摘要