Acoustic Detection Of Regionally Rare Bird Species Through Deep Convolutional Neural Networks
ECOLOGICAL INFORMATICS(2021)
摘要
Bioacoustic monitoring with machine learning (ML) models can provide valuable insights for informed decisionmaking in conservation efforts. In this study, the team built deep convolutional neural networks to analyze field recordings and classify calls of Yellow-vented warbler (Phylloscopus cantator) and Rufous-throated wren-babbler (Spelaeornis caudatus), both of which are regionally rare in Nepal. Data augmentation techniques for calls of the two bird species were utilized to effectively increase the size of the training set and thus boost model performance. Nepali ornithologists were engaged in iterative data labeling from field recordings, leveraging ML technology in conjunction with expert manual labeling and verification. The model output provides insights of species activity and abundance throughout 2018-2019 in multiple ecosystems along an elevational transect in the Barun River Valley, Nepal. The results of this study may help conservationists better understand species distribution, behavior, diversity, and habitat preference. Additionally, the results provide baseline data to quantify future changes due to habitat disruption or climate change. This modeling methodology and its framework can be easily adopted by other acoustic classification problems.
更多查看译文
关键词
Deep learning, Convolutional neural networks (CNN), Bioacoustic classification, Transfer learning, Species population, Presence survey
AI 理解论文
溯源树
样例
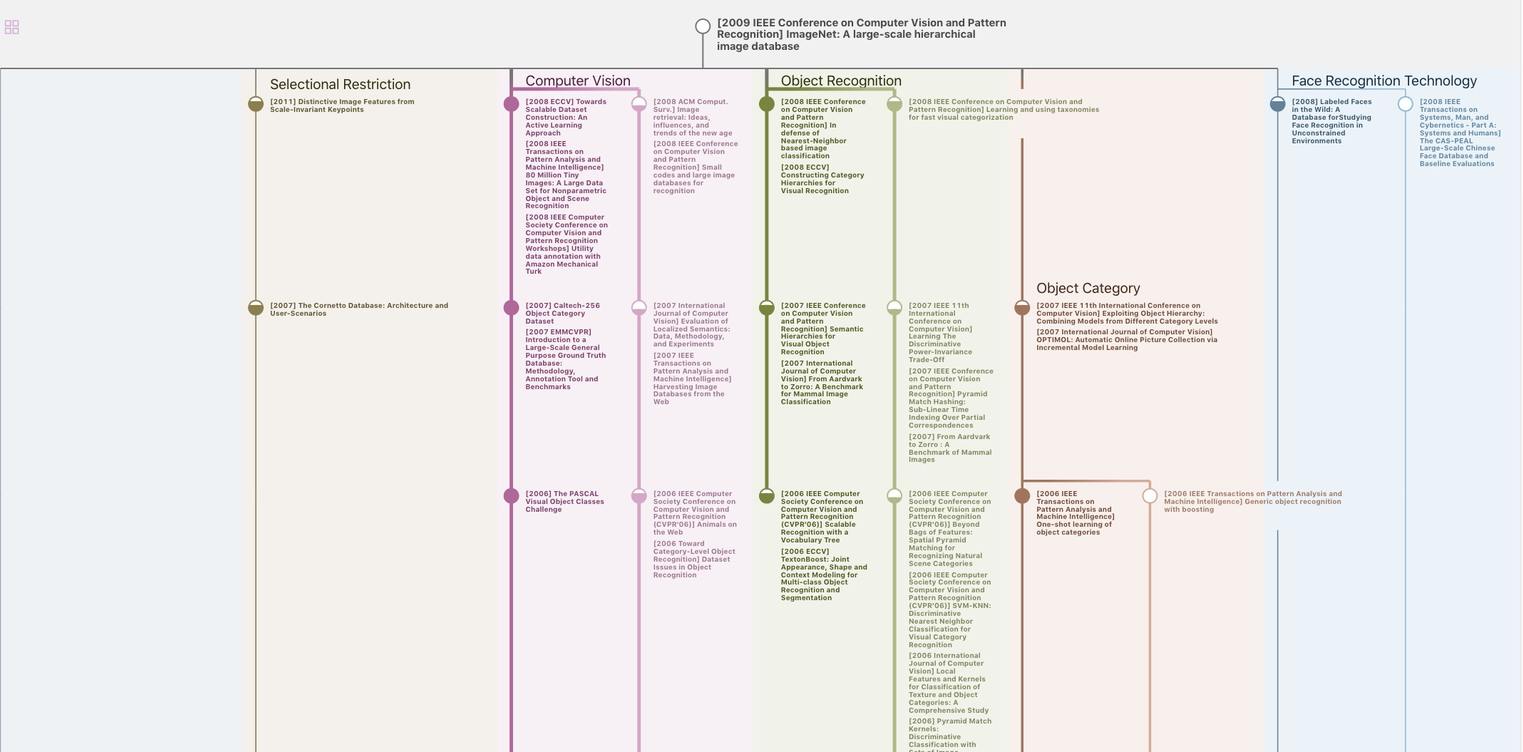
生成溯源树,研究论文发展脉络
Chat Paper
正在生成论文摘要