Scalable Feature Selection Using Relieff Aided By Locality-Sensitive Hashin
INTERNATIONAL JOURNAL OF INTELLIGENT SYSTEMS(2021)
摘要
Feature selection algorithms, such as ReliefF, are very important for processing high-dimensionality data sets. However, widespread use of popular and effective such algorithms is limited by their computational cost. We describe an adaptation of the ReliefF algorithm that simplifies the costliest of its step by approximating the nearest neighbor graph using locality-sensitive hashing (LSH). The resulting ReliefF-LSH algorithm can process data sets that are too large for the original ReliefF, a capability further enhanced by distributed implementation in Apache Spark. Furthermore, ReliefF-LSH obtains better results and is more generally applicable than currently available alternatives to the original ReliefF, as it can handle regression and multiclass data sets. The fact that it does not require any additional hyperparameters with respect to ReliefF also avoids costly tuning. A set of experiments demonstrates the validity of this new approach and confirms its good scalability.
更多查看译文
关键词
big data, feature selection, locality-sensitive hashing, ReliefF, scalability
AI 理解论文
溯源树
样例
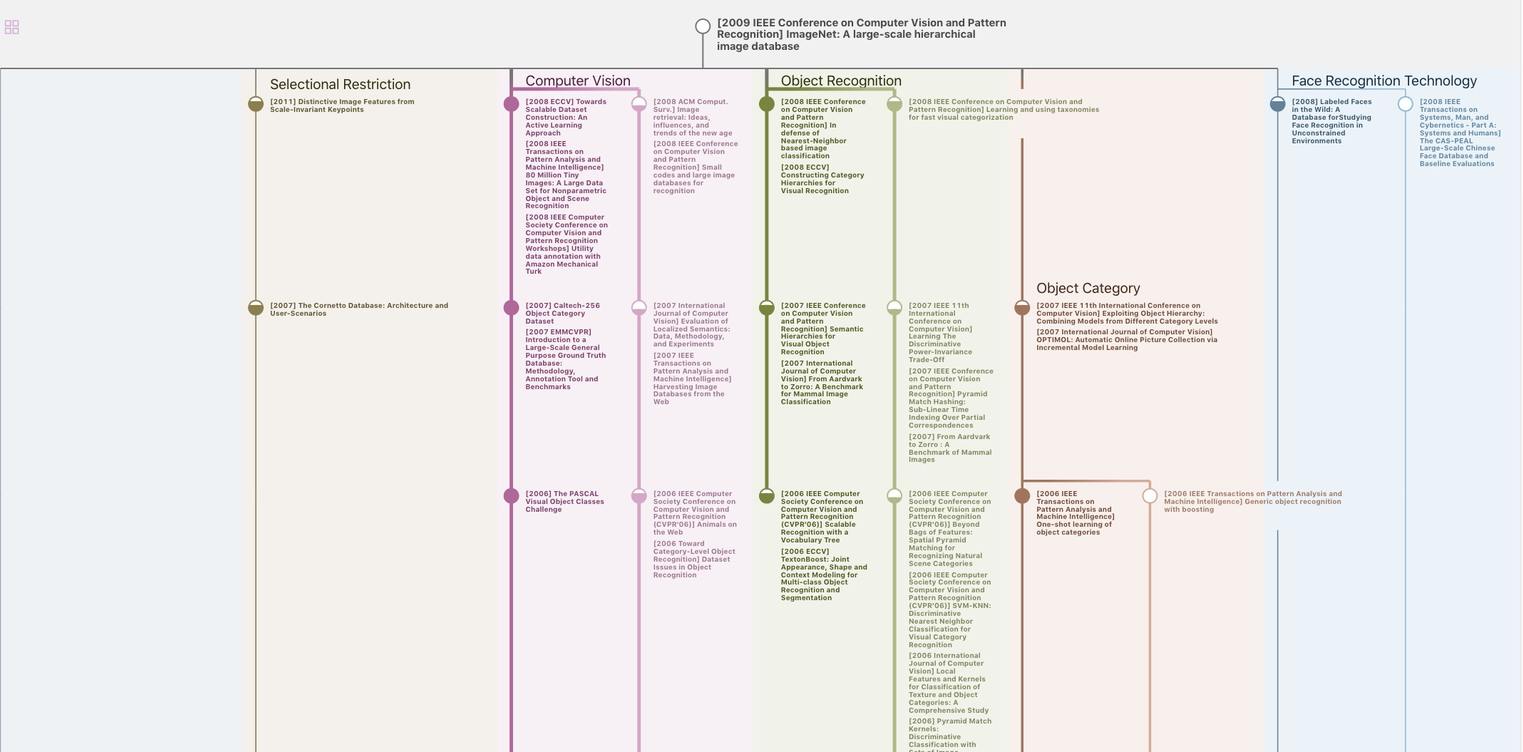
生成溯源树,研究论文发展脉络
Chat Paper
正在生成论文摘要