Reversible Gromov--Monge Sampler for Simulation-Based Inference\ast
SIAM JOURNAL ON MATHEMATICS OF DATA SCIENCE(2024)
摘要
This paper introduces a new simulation -based inference procedure to model and sample from multidimensional probability distributions given access to independent and identically distributed samples, circumventing the usual approaches of explicitly modeling the density function or designing Markov chain Monte Carlo. Motivated by the seminal work on distance and isomorphism between metric measure spaces, we develop a new transform sampler to perform simulation -based inference, which estimates a notion of optimal alignments between two heterogeneous metric measure spaces (\scrX, \mu , c\scrX) and (\scrY, \nu , c\scrY) from empirical data sets, with estimated maps that approximately push forward one measure \mu to the other \nu , and vice versa. We introduce a new notion called the reversible Gromov--Monge (RGM) distance, providing mathematical formalism behind the new sampler. We study the statistical rate of convergence of the new transform sampler, along with several analytic properties of the RGM distance and operator viewpoints of transform sampling. Synthetic and real -world examples showcasing the effectiveness of the new sampler are also demonstrated.
更多查看译文
关键词
Gromov-Wasserstein metric,transform sampling,simulation-based inference,generative models,isomorphism,likelihood-free inference
AI 理解论文
溯源树
样例
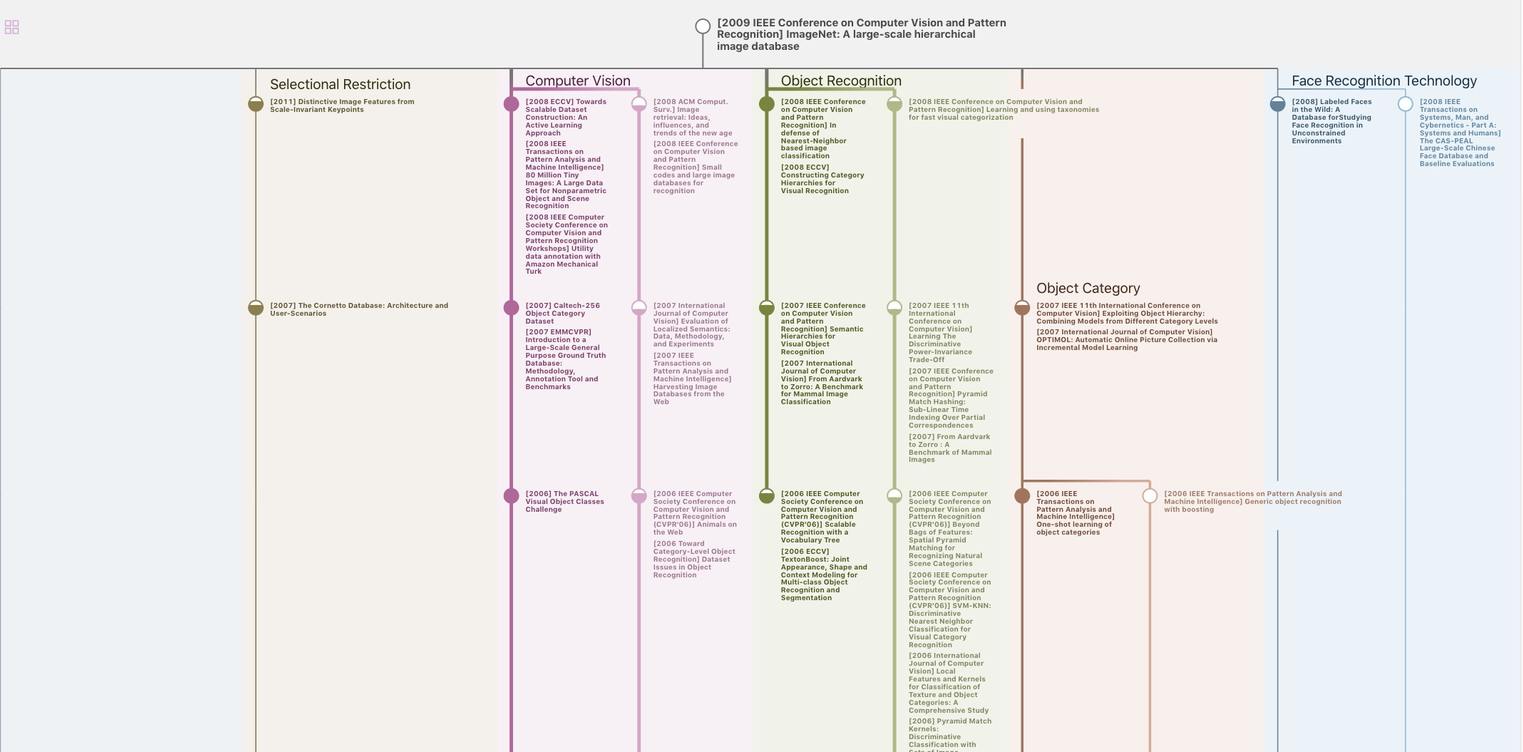
生成溯源树,研究论文发展脉络
Chat Paper
正在生成论文摘要