Def-IDS: An Ensemble Defense Mechanism Against Adversarial Attacks for Deep Learning-based Network Intrusion Detection
2021 International Conference on Computer Communications and Networks (ICCCN)(2021)
摘要
Network intrusion detection plays an important role in the Internet of Things systems for protecting devices from security breaches. Facing challenges of the rapidly increasing amount of diverse network traffic, recent research has employed end-to-end deep learning-based intrusion detectors for automatic feature extraction and high detection accuracy. However, deep learning has been proved vulnerable to adversarial attacks that may cause misclassification by imposing imperceptible perturbation on input samples. Though such vulnerability is widely discussed in the image processing domain, very few studies have investigated its perniciousness against network intrusion detection systems (NIDS) and proposed corresponding defense strategies. In this paper, we try to fill this gap by proposing Def-IDS, an ensemble defense mechanism specially designed for NIDS, against both known and unknown adversarial attacks. It is a two-module training framework that integrates multi-class generative adversarial networks and multi-source adversarial retraining to improve model robustness, while the detection accuracy on unperturbed samples is maintained. We evaluate the mechanism over CSE-CIC-IDS2018 dataset and compare its performance with the other three defense methods. The results demonstrate that Def-IDS is able to detect various adversarial attacks with better precision, recall, F1 score, and accuracy.
更多查看译文
关键词
Network intrusion detection,adversarial attacks,deep learning
AI 理解论文
溯源树
样例
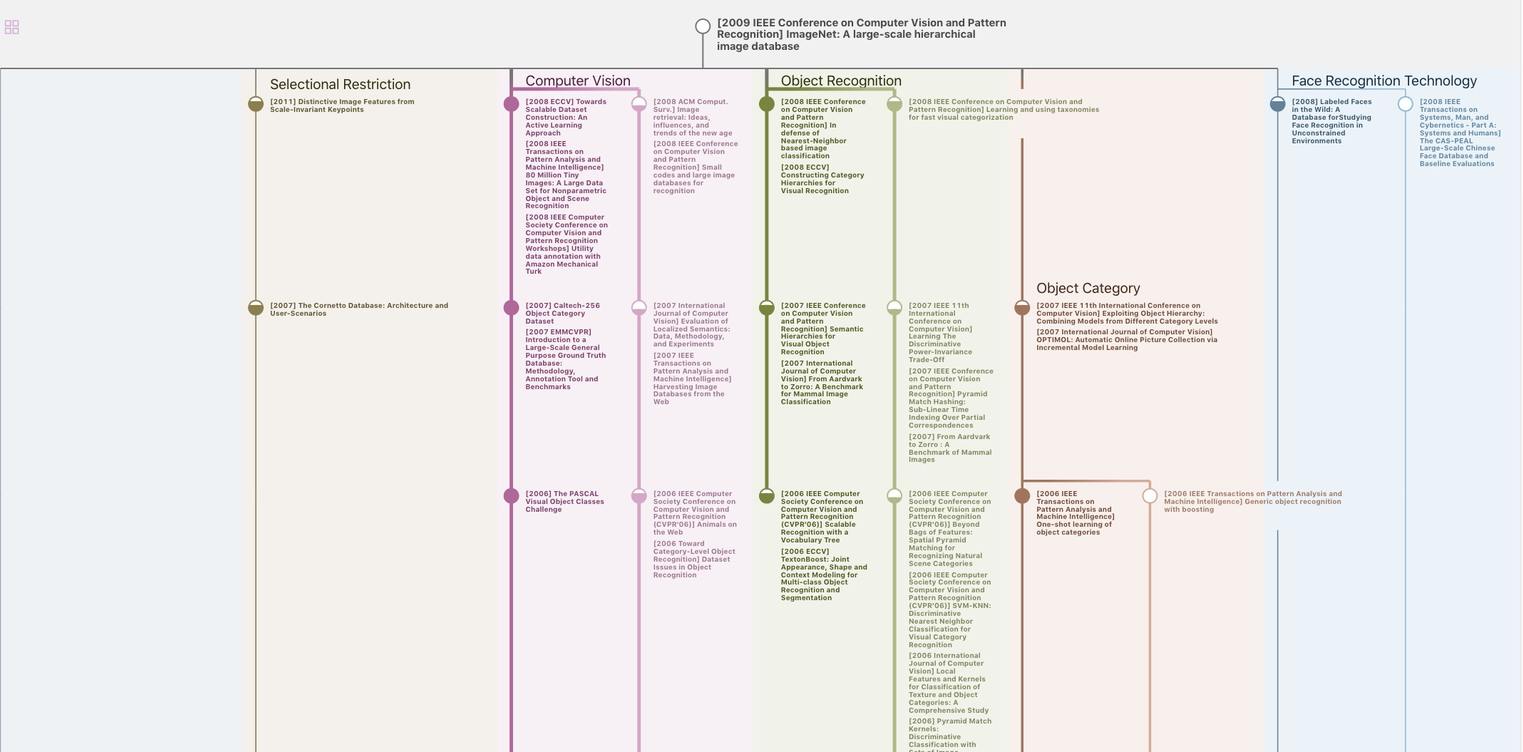
生成溯源树,研究论文发展脉络
Chat Paper
正在生成论文摘要