GAN-Based Adversarial Patch for Malware C2 Traffic to Bypass DL Detector
INFORMATION AND COMMUNICATIONS SECURITY (ICICS 2021), PT I(2021)
摘要
The constantly evolving malware brings great challenges to network security defense. Fortunately, deep learning (DL)-based system achieved good performance in the malware command and control (C2) traffic detection field due to its excellent representation capabilities. However, DL models have been shown to be vulnerable to evasion attacks, that is, DL models can easily be misled by adding subtle perturbations to the original samples. In this paper, we propose a GAN-based evasion method, which can help malware C2 traffic bypass the DL detector. Our main contributions contain: (1) directly generate adversarial traffic that can implement malicious functions by inserting additional adversarial patches in the original flow; (2) adaptively imitating victim's normal traffic by training GAN in victim environment, and introducing transfer learning to reduce the additional victim resource usage caused by GAN training. Results show that the adversarial patch generated by GAN can prevent malware C2 traffic from being detected with 51.4% success rate. The higher time efficiency and smaller malware impact make our method more suitable for real attacks.
更多查看译文
关键词
Malware C2 traffic, Evasion attacks, GAN, Transfer learning
AI 理解论文
溯源树
样例
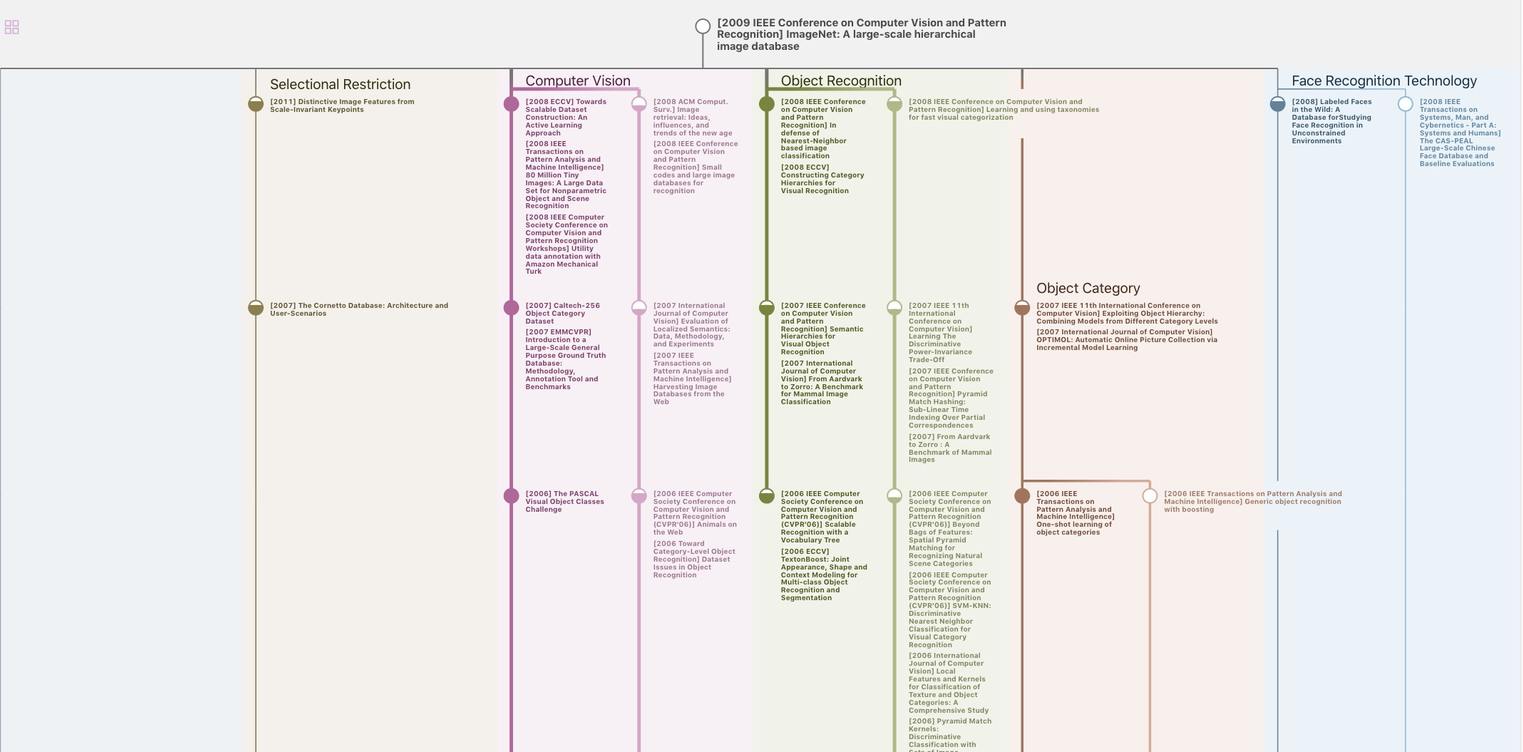
生成溯源树,研究论文发展脉络
Chat Paper
正在生成论文摘要