TranFuzz: An Ensemble Black-Box Attack Framework Based on Domain Adaptation and Fuzzing
INFORMATION AND COMMUNICATIONS SECURITY (ICICS 2021), PT I(2021)
摘要
A lot of research effort has been done to investigate how to attack black-box neural networks. However, less attention has been paid to the challenge of data and neural networks all black-box. This paper fully considers the relationship between the challenges related to data black-box and model black-box and proposes an effective and efficient non-target attack framework, namely TranFuzz. On the one hand, TranFuzz introduces a domain adaptation-based method, which can reduce data difference between the local (or source) and target domains by leveraging sub-domain feature mapping. On the other hand, TranFuzz proposes a fuzzing-based method to generate imperceptible adversarial examples of high transferability. Experimental results indicate that the proposed method can achieve an attack success rate of more than 68% in a real-world CVS attack. Moreover, TranFuzz can also reinforce both the robustness (up to 3.3%) and precision (up to 5%) of the original neural network performance by taking advantage of the adversarial re-training.
更多查看译文
关键词
Domain adaptation, AI security, Fuzzing, Black-box attack
AI 理解论文
溯源树
样例
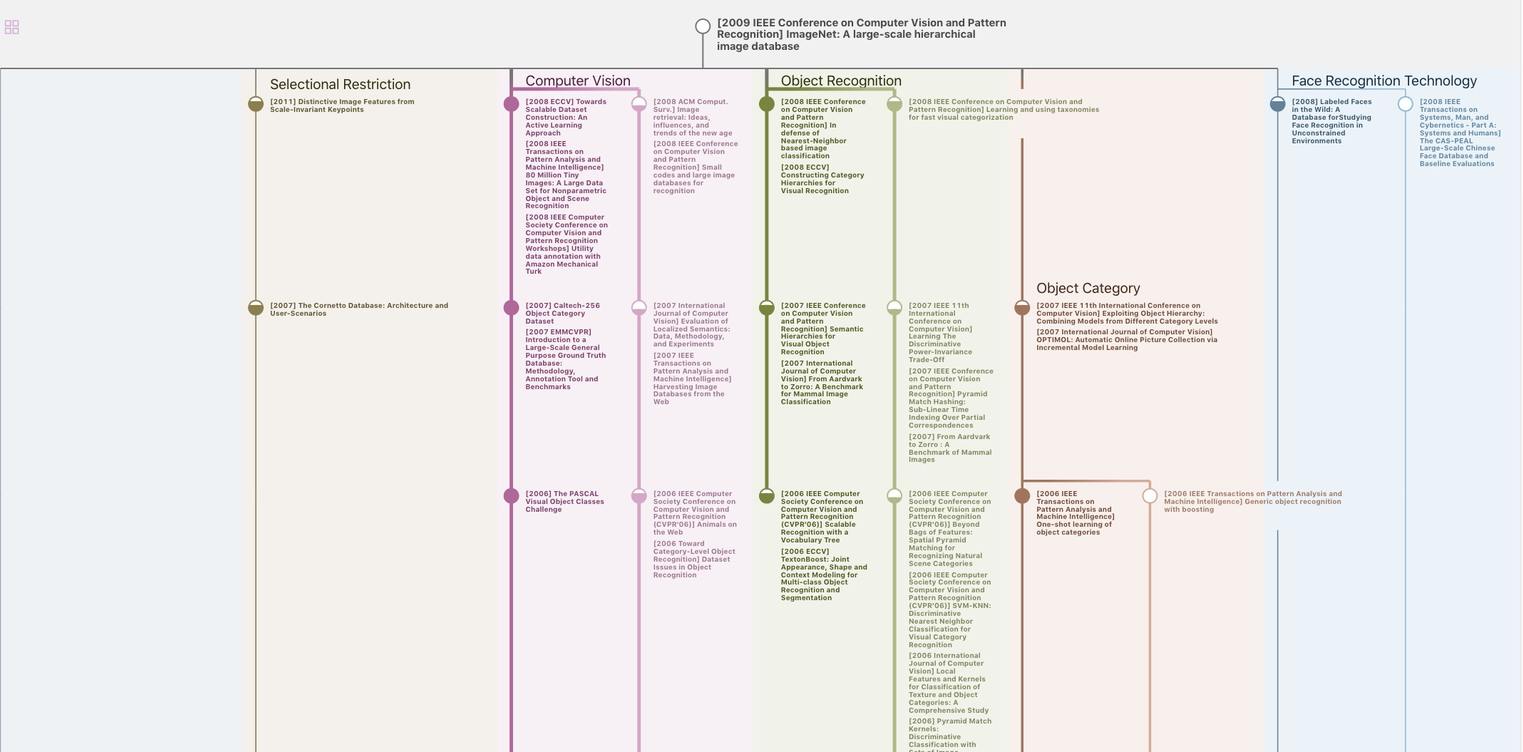
生成溯源树,研究论文发展脉络
Chat Paper
正在生成论文摘要