Medical named entity recognition of Chinese electronic medical records based on stacked Bidirectional Long Short-Term Memory
2021 IEEE 45TH ANNUAL COMPUTERS, SOFTWARE, AND APPLICATIONS CONFERENCE (COMPSAC 2021)(2021)
摘要
The wide adoption of electronic medical record (EMR) systems causes rapid growth of medical and clinical data. It makes the medical named entity recognition (NER) technologies become critical to find useful patient information in the medical dataset. However, the medical terminologies usually have the characteristics of inherent complexity and ambiguity, it is difficult to capture context-dependency representations by supervision signal from a simple single layer structure model. In order to address this problem, this paper proposes a hybrid model based on stacked Bidirectional Long Short-Term Memory (BILSTM) for medical named entity recognition, which we call BSBC (BERT combined with stacked BILSTM and CRF). First, we use Bidirectional Encoder Representation from Transformers (BERT) to perform unsupervised learning on an unlabeled dataset to obtain character-level embeddings. Then, stacked BILSTM is utilized to obtain context-dependency representations through the multi hidden layers structure. Finally, Conditional Random Field (CRF) is used to predict sequence tags. The experiment results show that our method significantly outperforms the baseline methods, it serves as a strong alternative approach compared with traditional methods.
更多查看译文
关键词
Electronic medical record (EMR), Named entity recognition (NER), Bidirectional Encoder Representation from Transformers (BERT), Stacked Bidirectional Long Short-Term Memory (BILSTM)
AI 理解论文
溯源树
样例
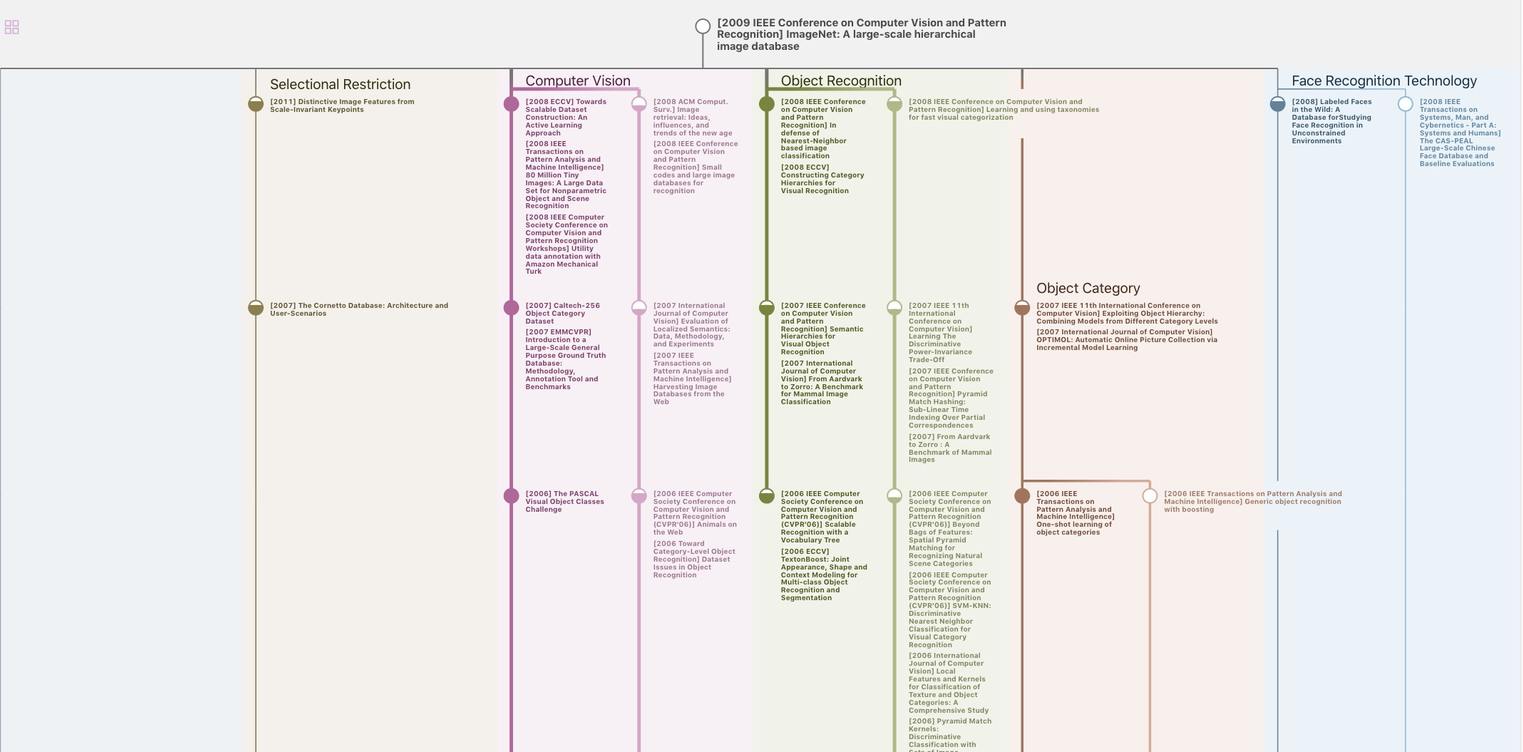
生成溯源树,研究论文发展脉络
Chat Paper
正在生成论文摘要